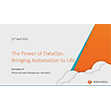
Stanford COVID-19 Model Identifies Superspreader Sites, Socioeconomic Disparities
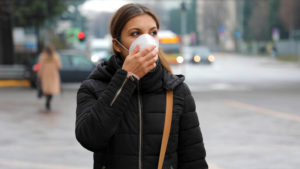
The COVID-19 pandemic is once again surging to unprecedented levels in the U.S., and all signs point to a grim winter punctuated by the return of many curfews and shutdowns. The conversation now focuses on how much of the economy can continue to operate without irresponsibly enabling the transmission of COVID-19 during a deadly surge. To that end, a team of researchers led by Stanford University has created a model that uses cell phone mobility data to map the most dangerous hubs for COVID-19 transmission in a given city.
The study used a combination of demographic data, epidemiological data, and anonymized cell phone location data to track how nearly 100 million Americans moved around from March to May in ten large cities: Atlanta, Chicago, Dallas, Houston, Los Angeles, Miami, New York, Philadelphia, San Francisco, and Washington. The location data was provided by SafeGraph, which offered mobility data matched with over 550,000 public locations like shops, restaurants, and churches, showing how many people visited those establishments, when they visited, and for how long. The dataset even included the square footage of those locations, allowing for density calculations.
Then, the researchers began tuning a model to predict infections in an area, tinkering with the variables until “predicted” results for past infections matched actual reported infections. Once predicted and reported infections matched, they tested the model on future infections, with the model tracking closely to reality. Finally, the researchers integrated demographic data to understand how different populations were affected.
“We built a computer model to analyze how people of different demographic backgrounds, and from different neighborhoods, visit different types of places that are more or less crowded,” said Jure Leskovec, the Stanford computer scientist who led the research, in an interview with Stanford’s Tom Abate. “Based on all of this, we could predict the likelihood of new infections occurring at any given place or time.”
The model identified a series of common “superspreader” sites in any given city, with dine-in restaurants, gyms, and cafes proving common culprits due to extended exposure to strangers in a relatively high-density environment.
Further, though, the integrated model painted a picture of how the ubiquitous pandemic was far from equally distributed. The model showed how minority and low-income residents were disproportionately exposed to these dangerous conditions – a new factor that compounds previous assumptions about the higher infection rates among those populations, and which opens doors to new solutions.
“In the past, these disparities have been assumed to be driven by preexisting conditions and unequal access to health care, whereas our model suggests that mobility patterns also help drive these disproportionate risks,” said David Grusky, a professor of sociology at Stanford. “Because the places that employ minority and low-income people are often smaller and more crowded, occupancy caps on reopened stores can lower the risks they face. We have a responsibility to build reopening plans that eliminate – or at least reduce – the disparities that current practices are creating.”
The reverse was true for socioeconomically advantaged populations: in areas where more people were able to stay at home, infection rates were lower. Overall, Leskovec said, the model offered “the strongest evidence yet” of the efficacy of stay-at-home policies in curbing infections.
The model and its data are now publicly available.