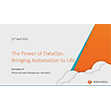
New MIT Analytics Tools Predict COVID-19 Patient Outcomes and More
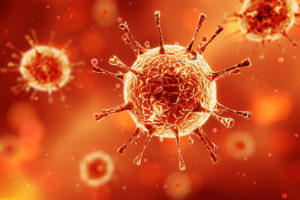
(red_diamond/Shutterstock)
With AI and supercomputing tools around the world laser-focused on producing candidates for COVID-19 therapeutics and vaccines, some doctoral students at the MIT Sloan School of Management are turning their machine learning and analytics skills against other COVID-19 targets, leveraging big data to analyze aspects of the pandemic ranging from ventilator allocation to patient risk factors.
The team, led by Dimitris Bertsimas (associate dean for business analytics at Sloan), is focusing on four key areas of the pandemic.
- Predicting patient outcomes
The team investigated a growing area of COVID-19 research: patient outcomes and risk factors. Bertsimas pointed out that most of the outcome prediction at the moment is based on age or symptoms, leaving room for more robust analysis. The team decided to work toward bridging that gap by applying machine learning to assess symptoms, demographics, pre-existing conditions, and test results in a clinically useful manner.
“We started with data published in Wuhan, Italy, and the U.S., including infection and death rate as well as data coming from patients in the ICU and the effects of social isolation,” Bertsimas said. “We enriched them with clinical records from a major hospital in Lombardy which was severely impacted by the spread of the virus. Through that process, we created a new model that is quite accurate. Its power comes from its ability to learn from the data. … By probing the severity of the disease in a patient, it can actually guide clinicians in congested areas in a much better way.”
From this work, they developed a mortality risk calculator that uses factors like gender, age, blood pressure, temperature, and cardiac frequency to predict COVID-19 risk mortality. The risk calculator can be found here.
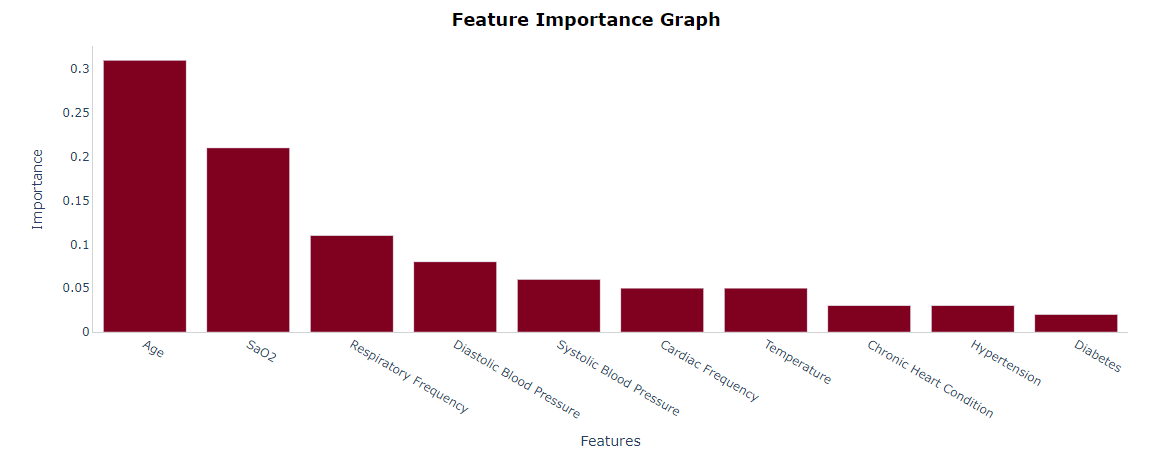
A chart of the predicted importance of various demographic and medical factors for COVID-19 mortality.
- Projecting the spread of COVID-19
The team developed a new epidemiological model, called DELPHI, to predict infections, hospitalizations and deaths. They describe DELPHI as a “standard SEIR model” (which breaks down populations of people who are Susceptible, Exposed, Infectious, or Recovered) with modifications to account for COVID-19-specific factors like difference among testing and policies between states. DELPHI can be found here.
- Optimizing ventilator allocation
With ventilators in such short supply that states are engaging in bidding wars with each other, the team examined the allocation of ventilators among states to see if there was room for optimization. They created another interactive application that allows users to tinker with variables (like the percentage of buffer ventilators beyond projected need) and outlines strategies for sharing ventilators among states to reduce long-term shortages. “Different regions will hit their peak number of cases at different times, meaning their need for supplies will fluctuate over the course of weeks. This model could be helpful in shaping future public policy,” Bertsimas said. The application can be found here.
- Testing for COVID-19 – conveniently
Finally, the team worked on developing an easier test for COVID-19 diagnosis. They pulled data from a set of samples in Morocco and fed it to a machine learning algorithm to modify an existing COVID-19 test. The researchers reported an improved accuracy rate of 90% with low false positives.
Moving forward
“The backbone for each of these analytics projects is data, which we’ve extracted from public registries, clinical Electronic Health Records, as well as over 120 research papers that we compiled in a new database. We’re testing our models against incoming data to determine if it makes good predictions, and we continue to add new data and use machine-learning to make the models more accurate,” says Bertsimas.
Looking ahead, the team aims to expand the epidemiological model around the globe, improve the risk calculator, and begin examining paths back to economic and social normalcy.
Related Items:
Data Transparency: Lessons from COVID-19
How Badly Will COVID-19 Hurt the Data Privacy Movement?
Coders Enlisted to Fight Virus as Post-Pandemic Data Gathering Begins
How AI Is Helping the Supply Chain Cope With COVID-19