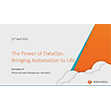
How 5G Will Serve AI and Vice Versa
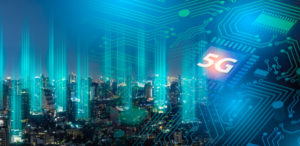
(Fit Ztudio/Shutterstock)
5G is the future of the edge. Though it’s still several years away from widespread deployment, 5G is a key component in the evolution of cloud-computing ecosystems toward more distributed environments. Between now and 2025, the networking industry will invest about $1 trillion worldwide on 5G, supporting rapid global adoption of mobile, edge, and embedded devices in practically every sphere of our lives.
5G will be a prime catalyst for the trend under which more workloads are executed and data resides on edge devices. It will be a proving ground for next-generation artificial intelligence (AI), offering an environment within which data-driven algorithms will guide every cloud-centric process, device, and experience. Just as significant, AI will be a key component in ensuring that 5G networks are optimized from end to end, 24×7.
How 5G Will Serve AI
AI will live at every edge in the hybrid clouds, multiclouds, and mesh networks of the future. Already, we see prominent AI platform vendors—such as NVIDIA—making significant investments in 5G-based services for mobility, Internet of Things (IoT) and other edge environments.
To better understand how 5G will superpower the online economy, let’s consider how this emerging wireless architecture will deliver value throughout the AI toolchain:
- Next-generation edge convergence with AI systems on chip: 5G converges digital cellular technology with wireless Long-Term Evolution and Wi-Fi interfaces. When implemented in cross-technology network interfaces, 5G will enable every edge device to seamlessly roam between indoor and wide-area environments. The technology’s adoption may someday lead to convergence of the radio spectra for these disparate radio channels and convergence of network interfaces down to single chips that are agile at maintaining seamless connections across multiple radio access technologies. These same 5G interfaces will undoubtedly be converged with neural network processing circuitry into low-power, low-cost systems on chip for many mass-market AI apps.
- Massive device concurrency replenishing AI data lakes in real time: 5G can support up to a million concurrent edge devices per square kilometer, which is an order-of-magnitude greater concurrency than with 4G technology. That “last mile” scale will enable businesses to collect vast amounts of data continuously from mobile phones, sensors, thermostats, and other 5G-equipped devices in an emerging paradigm known as “multi-access edge computing.” As 5G networks begin to flood data streams and lakes everywhere with fresh data from devices, AI application developers and data scientists will be able to build more sophisticated analytics and machine-learning models for real-time applications in IoT, mobility, industrial automation, smart cities, and countless other use cases.
- Ultra-fast, high-volume streaming for low-latency AI: 5G connections have much lower latencies than 4G, as low as 1 millisecond vs the 50 milliseconds that is characteristic of 4G. Consequently, 5G has much faster download and upload speeds than 4G: 20 gigabits per second, which is more than 1,000 times 4G’s rate of 5-12 megabits per second. 5G’s greater application bandwidth and transmission capacity connection stem from its ability to transmit multiple bitstreams of data simultaneously in both directions between the base station and edge devices. These performance advantages enable 5G to support AI DevOps pipeline workloads–from data ingest and preparation to model building, training, and serving—in low-latency, real-time streaming scenarios. In addition, 5G’s faster download speeds, when combined with its much lower latency, will enable analysts to collect, clean and analyze a lot more data in a much shorter time.
How AI Will Serve 5G
AI is also a key component in the infrastructure for ensuring that 5G networks, in all their complexity, can support with AI and other application workloads. Recently published research shows that many wireless operators around the world are well on their way to deploying AI for managing their 5G and other networks.
To serve the next generation of distributed AI apps effectively, 5G networks will need to become continuously self-healing, self-managing, self-securing, self-repairing and self-optimizing. That, in turn, relies on embedding of machine learning and other AI models to automate application-level traffic routing, quality-of-service assurance, performance management, root-cause analysis, and other operational tasks more scalably, predictably, rapidly, and efficiently than manual methods alone.
That capability, often known as AIOps, will be key to 5G delivering on its promise of substantially faster, more reliable, and more RF-efficient connections than prior wireless technologies. AIOps capabilities will need to be integral to the network virtualization and multicloud management suites that are used to manage 5G networks and associated applications from end to end.
At the very least, AIOps will drive differentiated quality-of-service from end-to-end across 5G environments. AI-based controls will ensure that RF channels and other infrastructure resources are provisioned dynamically and precisely to support changing quality-of-service requirements, traffic patterns and application workloads. They will also support continuously predictive alarm management, configuration and healing, and subscriber experience optimization.
AIOps tooling will supplement a 5G infrastructure capability known as “network slicing.” This enables 5G networks to run several virtual networks over one physical connection. Leveraging this virtualized resource provisioning capability, AIOps tooling will support predictive and dynamic delivery of distinct wireless quality-of-service tiers for diverse customer types and edge-device classes.
AIOps will also be needed to augment 5G’s dynamic RF-channel allocation features. 5G has smaller cells than 4G, reuses frequencies more intensively, and must continuously retarget “beamformed” base station phase-array millimeter-wave antennas at each edge device. To ensure quality of service, 5G base stations dynamically predict and provision the best wireless path to each device. They do this while continuously accounting for the difficulties that 5G’s millimeter waves encounter in passing through walls and other solid objects. AI-driven closed-loop real-time analytics are acutely needed to perform these calculations in real-time across dynamically changing wireless local loops.
Of course, all of this AI in the 5G network will create a demand for data management infrastructure to sustain it all. We can well expect that dedicated data lakes, AutoML tooling, AI DevOps repositories, and other key operational infrastructure will spring up throughout 5G networks to ensure that the best-fit AI models are deployed in real time. This data/model management infrastructure will be deployed in cloud-to-edge configurations that align with the complex public/private federated environments that will be characteristic of 5G.
For all of these reasons, the time has come for service providers and enterprise IT professionals alike to explore the critical role that AI will play in their 5G and edge-computing plans.
About the author: James Kobielus is Futurum Research‘s research director and lead analyst for artificial intelligence, cloud computing, and DevOps.
Related Items:
Are You Prepared for the 5G Data Crush?
Giving DevOps Teeth To Crunch Down on AI Ethics Governance
Let’s Accept That AI Leadership Is Everywhere