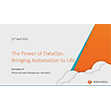
Data Lakes Get Structured
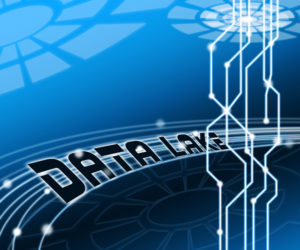
The explosion of unstructured and partially structured data has made traditional data lakes harder to manage. Adding to the challenge are “brittle” data pipelines that are time-consuming to create as well as ephemeral.
Or to put it another way, “Pipelines Suck,” asserts autonomous dataflow startup Ascend, which is rolling out a “structured data lake” designed to connect existing data processing engines, business intelligence tools and notebooks on its data management platform.
The startup based in Palo Alto, Calif., emerged in July with its dataflow service designed to allow data engineering teams to build and scale Apache Spark-based data pipelines. Ascend claims its service enables pipeline creation with 85 percent less code and reduces the time from prototype to production by 90 percent.
The unstructured data lake is touted as addressing the dataflow challenges that often sink AI and big data deployments via a tool for accelerating data development across managed storage. The idea is to provide development teams access to more organized data. “We are eliminating siloed access based on preferred tools or skills,” said Sean Knapp, Ascend’s founder and CEO.
Ascend’s structured data lake is implemented on Amazon Web Services’ (NASDAQ: AMZN) Simple Storage Service (S3) API, an interface the startup said it best suited to working with external data processing platforms. Along with Apache Spark for handling S3 data paths, the new data lake uses the MinIO open-source protocol designed for AI object storage to simplify implementation of the S3 API layer.
In Ascend’s framework, MinIO handles processing of the S3 API protocol, so only logic need be implemented to map virtual data paths and the underlying objects.
Other capabilities included in the structured data lake include automated storage maintenance, de-duplication of redundant storage and operations along with tighter management of all data and updates.
“Managed data is unified and dynamically synchronized with the pipelines that operate on it,” the company noted in a blog post. Those capabilities would allow data scientists and engineers to “build on top of a common data lake that automatically ensures data integrity, tracks data lineage, and optimizes performance.”
Ascend announced a Series A funding round in July led by Accel with participation from Sequoia Capital, Lightspeed Venture Partners and 8VC. Among the startup’s advisors are Scott McNealy, former CEO of Sun Microsystems and Microsoft (NASDAQ: MSFT) CTO Kevin Scott.
Recent items:
Ascend Launches from Stealth With $19M
Four Ways Automation Can Rescue Your Data Lake