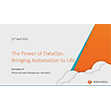
Migration Tools Needed to Shift ML to Production
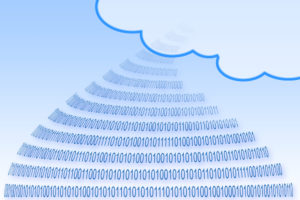
via Shutterstock
The confluence of accelerators like cloud GPUs along with the ability to handle data-rich HPC workloads will help push more machine learning projects into production, concludes a new study that also stresses the importance of cloud migration and accompanying tools.
The survey released this week by workload management specialist Univa Corp. confirms the rush to machine learning. However, it also found a lack of workloads in production. The reason, according to a survey of 344 IT managers is lingering problems with cloud migration, including workloads, data and applications.
While the vast majority of respondents (93 percent) said they have machine learning projects underway, only 22 percent have been able to move them to production.
“Our customers are already asking for guidance with migrating their HPC and machine learning workloads to the cloud or hybrid environment,” said Rob Lalonde, a Univa vice president. What is needed, Lalonde added, is new tools and migration options to transform more machine learning workloads into actual applications.
Among the tools are GPU accelerators available in the cloud that are rapidly becoming the foundation of machine learning infrastructure. Nine of out ten respondents in the Univa survey said they expect to use cloud GPUs to accelerate the shift of machine learning workloads to production.
Another 80 percent said they will leverage hybrid cloud infrastructure for their machine learning projects as a way to reduce costs.
The survey also found a direct correlation between high-performance computing and machine learning, with 88 percent utilizing HPC-class capabilities as they rolled out new data-driven projects. Among the early enterprise leaders in machine learning are the financial services and health care sectors, the survey found.
Chicago-based Univa specializes in software designed to manage big data workloads by maximizing shared infrastructure while accelerating application deployment either on-premises or in the cloud. Its flagship Grid Engine platform includes a preemption feature that allows administrators to pause workloads, then resume them without starting from scratch.
Cloud and tool vendors like Univa are increasingly focusing on the shift to HPC and the growing role of GPUs for the number-crunching required for deep learning workloads. Public cloud giants Amazon Web Services (NASDAQ: AMZN) and Google (NASDAQ: GOOGL) have moved aggressively to offer access to cloud GPUs for accelerating machine learning workloads.
Meanwhile, GPU leader Nvidia (NASDAQ: NVDA) is expanding its hybrid offerings with a new Turing-based T4 GPU released last week that is aimed at AI inference tasks in the datacenter.
Recent items:
Univa Gives ‘Pause’ to Big Fast Apps
The Art of Scheduling in Big Data Infrastructures Today