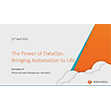
Univa Gives ‘Pause’ to Big Data Apps
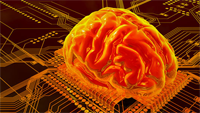
Scheduling workloads on today’s big analytic clusters can be a big challenge. Your team may have carefully everything lined up, only to have a last-minute change leave your schedule in shambles. One company that’s close to a solution is Univa, which today announced the addition of its “preemption” feature that allows admins to momentarily “pause” workloads so they can run a higher priority application.
Historically, admins were loathe to stop HPC or big data jobs before they ended, because it could be so expensive to get the job started again. But the preemption feature that Univa is shipping in Grid Engine 8.3 gives admins the power to pause a workload, and then resume it moments later, without the need to start from the beginning.
“You could liken the function to a home DVR system,” says explains Bill Bryce, Vice President of Products at Univa. “Users can be confident that if they pause one program to watch another, they will always be able to come back to finish the first.”
According to Univa, which made the announcement at the International Supercomputing Conference being held this week in Frankfurt, Germany, says this is the first time a preemption feature has been able to work with big data apps.
While the Univa Grid Engine is commonly found in HPC environments, it also supports big data workloads, such as Hadoop and MapReduce. In fact, the Univa Grid Engine provides essentially the same basic functionality that resource schedulers like YARN and Mesos—as well as more advanced functions, such as the new preemption feature.
Univa CTO Fritz Ferstl provided a good description of the Univa Grid Engine’s place in a big data world in a 2013 Datanami story. He writes:
“Univa Grid Engine and similar workload management tools have matured over the past two decades in technical and scientific computing, as well as in HPC, and have evolved to become an essential cog in Big Data infrastructures. Today Univa Grid Engine supports an impressive and essentially open-ended spectrum of use case scenarios. They are being deployed across all market sectors and typically in business and performance- critical situations.
“Univa Grid Engine, in particular,” he continues, “also supports a scale exceeding 100,000 cores with massive throughput of jobs of any size. Scale and throughput is essential in Big Data environments because Big Data workloads (also known as ‘jobs’) often have comparatively short runtimes, while the amount of data to be analyzed is massive. This results in a large count of jobs to be processed on growing cluster sizes as data volumes and time-to-result requirements increase.”
Related Items:
The Art of Scheduling in Big Data Infrastructures Today
Managing MapReduce Applications in a Shared Infrastructure