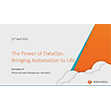
Increased Complexity Is Dragging on Big Data
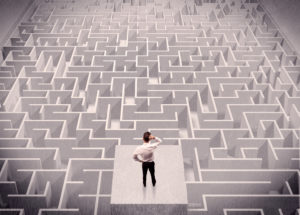
(ra2studio/Shutterstock)
For all the progress that companies are making on their big data projects, there’s one big hurdle holding them back: complexity. Because of the high level of technical complexity that big data tech entails and the lack of data science skills, companies are not achieving everything the’d like to with big data.
That’s one of the key takeaways from a new report issued today by Qubole, the big data as a service vendor founded by Apache Hive co-creator Ashish Thusoo. Qubole hired an outfit called Dimensional Research, which surveyed more than 400 technology decisions makers about their big data projects, and published the results today in “2018 Survey of Big Data Trends and Challenges.”
Big data’s complexity problem surfaced in several ways. For starters, while seven out of 10 survey-takers report they want to enable self-service access to data analytics environments eventually, fewer than one in 10 actually have enabled self-service at this point in time, according to the survey.
Complexity was also evident in the personnel that companies are trying to hire. While nearly 80% of companies say they are planning to increase big data practitioners over the coming year by a moderate or significant amount, only 17% said they found recruiting easy. More than 80% say finding qualified big data professionals is difficult.
The number of administrators required to support big data users is another indication of the complexity problem infiltrating big data. Qubole says only 40% of survey-takers report their admins are able to support more than 25 users, “a startling number, since today’s flat budgets call for admins to serve more than 100 users,” the report states.
“All of these data points point to that — complexity on one side and lack of experience on the other. That gap is very, very stark,” Thusoo tells Datanami. “They do know that the potential of building out data lakes is there, but the complexity is too high and the capability is not there. So that’s the gap. The data points in the survey all point to that.”
The complexity is a result of several factors, including the types of big data projects that companies are embarking upon, the expectations they carry, and the diversity of tools they’re using, Thusoo says. When you couple that with a data science talent shortage, the complexity problem becomes more acute.
“Data getting bigger. That hasn’t changed,” says Thusoo, who previously ran Facebook‘s Data Infrastructure when he created Hive with colleague Joydeep Sen Sarma. “What has changed is the analytics are becoming more and more advanced. In the past couple of years, the AI stuff has become all the rage.”
A lot of companies are trying to deliver the same type of self-service access that they delivered for data warehousing and business intelligence users. Those delivery patterns have been well established and the results are good. “But the same sort of transformation hasn’t happened in the land of advanced analytics, AI, and machine learning,” Thusoo says. “That jumps out.”
The survey also tracked usage of popular big data technologies, and highlighted some interesting trends. Apache Flink, in particular, appears to be enjoying a breakout year, as its usage increased by 125% compared to the same survey Qubole conducted in 2017. That was the biggest increase among the 11 frameworks that it tracked, although Flink’s usage is still relatively small, as it’s used at just 9% of the professionals surveyed.
Presto, a promising next-gen SQL engine that runs on Hadoop and other platforms, had the second-biggest gain, at 63%, followed by Impala at 37% and Spark at 29%. Presto outgunned Impala in the overall usage category, 13% to 11%, among SQL engines, although Spark trounced them all with a 31% usage rating.
Thusoo says the churn in big data engines is a good thing, and shows that users are willing to try new technology that will give them an edge. But the diversity also contributes to an increase in technical complexity, and that is a problem that’s holding people back.
“It used to be the case, back in the day….that all the workloads would run on Hadoop and Hive. It’s like when you have a hammer, every problem looks like a nail,” he says. “Then Spark emerged and Spark took a lot of the machine learning workloads, which were very hard to implement on Hadoop and Hive.”
But then Apache Spark became the hammer, and was used for everything from machine learning and SQL processing to real-time streaming (its graph environment never seemed to make much progress). Now the big data community is realizing other engines may do a better job than Spark. The beneficiaries appears to be Flink (for real-time streaming) Presto, for (at-scale SQL processing), and Tensorflow (for deep learning).
According to Thusoo, 75% of Qubole customers use more than one engine (the company doesn’t support Flink on its cloud offering, but could in the future). “All this is emblematical of the fact that the complexity of the analytics that is being done on the database is changing,” he says. “It’s evolving. There was no deep learning three or four years back. It was very nascent, and now it is becoming suddenly mainstream.”
Hadoop so far is weathering this approach, as it supports many of the engines that people want to use, although neither Spark, Flink, Presto, nor Tensorflow are tied to Hadoop. “There’s still growth,” Thusoo says of Hadoop. “It’s not like it’s not growing. But no single engine can solve all the analytics issues. And I feel that’s being reflected in what is happening in Hadoop as well, that they cannot solve every use case.”
The big winners out of this could be the cloud vendors. According to Qubole’s survey, 73% of survey-takers are using the cloud, up from 58% in 2017. There are several reasons for the growth of clouds for big data workloads.
“Because of the complexity involved, a turnkey solution in the cloud, or a cloud service, helps to hide or abstract away the complexity form users and that also helps for big data,” Thusoo says. “The machine learning, AI, and advanced analytics workloads have certain needs from infrastructure, certain peculiar properties, and those properties are best met by cloud platforms.”
We’re still in the midst of hashing out reference architectures for machine learning, AI, and advanced analytics workloads, but it’s a good bet that those architectures will look more complex than BI and DW architectures of the past. If there are customers to be won and dollars to be made, then you can bet that companies will do it, complexity be damned.
“The data complexity is the same, but the technology complexity as well as the analytic complexity have both increased,” Thusoo says, “and I think that makes this an even harder problem than it was previously.”
Related Items:
Presto Use Surges, Qubole Finds
Don’t Let Data Complexity Stunt Your Company’s Growth
Hiding Hadoop: How Data Fabrics Mask Complexity and Empower Users