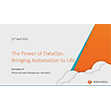
Three Myths of Big Data: Busted
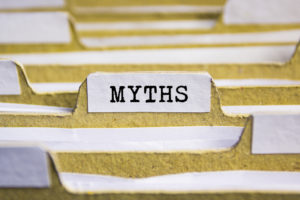
(Sinart Creative/Shutterstock)
Big data is a scary thing. If you’re tasked with moving, storing, or analyzing it, big data can cause all sorts of headaches. But as troublesome as it is, we shouldn’t create monsters where none exist.
Anil Gadre, the chief product officer with MapR Technologies, kicked off last week’s MapR Convergence event in San Diego with some myth busting. His first myth — that succeeding in AI is all about picking the right algorithm — didn’t stand a chance.
“In reality, it’s a continuum,” says Gadre, a Silicon Valley veteran who previously worked at Sun Microsystems. Many MapR customers employ a range of computational models in their big data operations, including batch, micro-batch, streaming analytic, and event processing, in addition to deep learning.
The key to succeeding with big data, Gadre says, comes down to combining informational context with speed to generate action. Many MapR customers want to stay open to the next popular big data tool, whether its Spark or TensorFlow or Caffe. And while many MapR customers are getting value from algorithms, maintaining the models as part of a DataOps strategy is a bigger chore than many realize.
The second myth busted by Gadre was that containers are just for stateless applications. In fact, the MapR platform uses containers to support stateful applications too, he says. What’s more, data science workloads are a perfect fit for containerization technologies like Docker and Kubernetes because of their constantly changing nature.
IT professionals should embrace containers as a way to appease data scientists’ insatiable appetite for new technologies and processing capacity. “They’re too demanding. They want too much freedom,” Gadre says, referring to how IT pros view data scientists. Containers are the answer, he says.
Think going all cloud with your big data setup is the way to go? Then you probably didn’t hear Gadre’s third busted myth at the La Jolla Hyatt Regency, which is that customers are better off putting their data and infrastructure in the hands of a cloud provider.
While you may think that moving into a cloud providers’ collection of big data services is a way to keep your options open, Gadre argues that you’re actually narrowing your options. “You’re really choosing a software stack,” he says of cloud adoption.
One way to keep your options open is to run a data platform, like MapR’s offering, on cloud infrastructure. And because of the different processing and scheduling options supported by MapR, customers can actually save money by running it in the cloud, Gadre says.
Related Items: