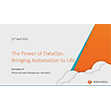
Embedded FPGA Core Aimed at Deep Learning
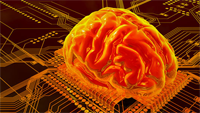
Different implementations of FPGA designs continue to make inroads in the development of AI applications such as neural network processing. The latest is an embedded FPGA core unveiled this week by chip designer Flex Logix.
The chip startup based in Mountain View, Calif., said Monday (June 25) the latest addition to its embedded FPGA family aims to boost the performance of deep learning tasks by as much as ten times while enabling greater neural network processing per square millimeter of chip real estate.
Flex Logix differentiates its FPGA design approach to AI development by stressing a critical function called matrix multipliers consisting of arrays of multiplier accumulators. Most FPGAs are optimized for digital signal processors with large multipliers. The company argues that approach is “overkill for AI.” Instead, the startup’s embedded FPGA scheme uses smaller multipliers (8- or 16-bit, for example) better suited to AI applications.
One reason is that smaller multipliers support both accumulator modes, meaning they allow more neural network processing within the chip.
Along with greater processor density, Flex Logix argues that AI developers want more flexibility to reconfigure designs as AI algorithms evolve. Hence, the ability to switch between 8- and 16-bit modes to implement matrix multipliers of various sizes is seen as way to meet application performance and cost requirements, the company said.
Further, Flex Logix said its AI embedded FPGA core can be implemented on most chip manufacturing processes in as little as six months.
The four-year-old startup’s patented interconnect technology for implementing embedded FPGAs is touted as delivering twice the density of traditional FPGA mesh interconnects.
AI researchers at Harvard University were among the first customers for the embedded FPGA core. Geoff Tate, a co-founder of Flex Logix, said Harvard researchers will present a research paper during the Hot Chips symposiumin August. They also are working on a follow-on 16-nanometer AI chip.
Tate was the founding CEO of Rambus, which pioneered the semiconductor intellectual property business model.
The embedded FPGA core is part of a growing list of custom AI hardware designed among other things to improve deep learning training. For example, chip giant Intel Corp. (NASDAQ: INTC)
has targeted the deep learning sector with its 2015 acquisition of FPGA specialist Altera, followed by its acquisition of Nervana Systems.
Recent items:
A Wave of Purpose-Built AI Hardware is Building
Microsoft Beefs Up Being with Intel FPGAs
AWS Partner Ryft Leverages Cloud FPGAs