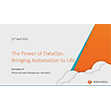
Databricks Eyes Data Engineers With Spark Cloud
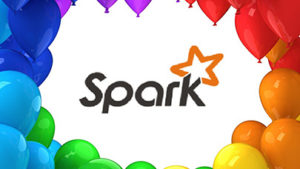
(Leigh Prather/Shutterstock)
Apache Spark creator Databricks rolled out a new version of its cloud platform based on Spark that specifically targets data engineering workloads.
The company said Wednesday (April 12) its data science platform would enable data engineers to combine SQL, structured streaming, ETL and machine learning workloads running on the cluster-computing framework. The goal is to accelerate secure deployment of data pipelines in production, the San Francisco-based company said.
The data engineering platform also seeks to move Spark deeper into enterprises by delivering what Databricks calls a “unified data analytics platform” that promotes collaboration among data scientists and decision makers. With that in mind, the cloud platform integrates with the company’s data science “workspaces” to streamline the “transition between data engineering and interactive data science workloads.”
The new Databricks platform also appears to address the growing demand for data engineers, a relatively new position that is a kind of hybrid between data analysts and data scientists. Data engineers excel at manipulating huge amounts of data and ensuring the entire big data software stack can scale to support massive workloads.
Databricks maintains organizations face challenges building Spark-based systems to meet the demands of data engineers that include tasks such as data cleansing and analysis. Hence, it is offering a “unified environment” to boost collaboration between data engineers and scientists along with Spark performance increases to develop, for example, intelligent algorithms for automating business processes.
The company claims its cloud-based platform can deliver as much as a ten-fold boost in an optimized version of Spark that handles a variety of instance types. It also is offering an accelerated access layer via Amazon Web Services’ (NASDAQ: AMZN) Simple Storage Service. Meanwhile, tools and services such as Amazon Redshift data warehousing along with machine learning frameworks like TensorFlow are deployed via REST APIs that also launch clusters and jobs.
At the same time, the expanding Spark community has been working to boost the performance of applications running under the SQL and Dataframe APIs, which have been stabilized.
Databricks said pricing for its data-engineering platform is based on workloads such as ETL and automated jobs, which works out to 20 cents per Databricks unit plus the cost of the AWS cloud.
The data-engineering platform is among a raft of new Spark features and enhancements planned for this year. Apache Spark creator Matei Zaharia said recently they include the introduction of a standard binary data format, better integration with Kafka, and even the capability to run Spark on a laptop. Automated creation of continuous applications in Spark remains a long-term goal, Zaharia said during a recent company event.
Recent items:
What’s In the Pipeline For Apache Spark?
Databricks CEO on Streaming Analytics, Deep Learning and SQL