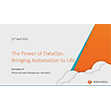
Outsmarting Wine Snobs with Machine Learning
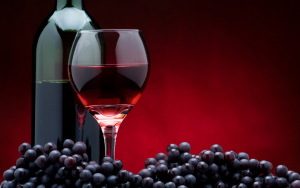
For most of us, picking good wine is a bit like picking ponies: Everybody has a method, but at the end of the day, the results aren’t much better than chance. But one budding wine connoisseur/hacker at a big data analytics firm thinks he may have landed upon an approach to predicting the quality of wine. His secret? Machine learning.
When he’s not solving big data problems or riding his road bike, H2O’s Alex Tellez enjoys exploring the world of wine. Recently Tellez was exposed to some of the wines coming out of Bordeaux, the legendary wine growing region along France’s southwest coast.
“I thought, can I apply some machine learning to Bordeaux wine?” says Tellez, who has the enviable title “cyclist applications and community hacker” at the Mountain View, California company. All too often, machine learning applications are cloaked in obtuse examples, so he jumped at the chance to apply the technology with hearty reds.
As he looked at the data, Tellez was immediately struck by what appeared to be an anomaly. In 2009 and 2010, it was the first time in half a century with consecutive years where the world’s top wine experts agreed that the Bordeaux region produced wines that were superior to average years.
Then the idea came to him: What if he could find a correlation between historical weather data for the Bordeaux region and the quality of the wine for that year? Tellez readily admits that there’s a lot more that goes into wine than the weather. But it’s generally understood that weather is one of the key factors dictating how good or bad a given year’s crop will be.
So Tellez decided to build a neural network to test his theory. First, he loaded 60 years’ worth of weather data for the wine growing region of Bordeaux. This included the amount of winter rain (from October to April), the average summer temperature (April to September), the existence of rain during harvest (in August and September), and the number of years since the last great vintage.
Then he ran the data through a type of unsupervised machine learning algorithm in the H2O product set called an auto encoder. After training the auto encoder to learn the typical, or the non-vintage year weather patterns, then Tellez fed the model with weather data from the vintage years. If everything worked correctly, those vintage years should show up in the results as outliers, or anomalies.
It worked. “Every single time, it’s bang on the spot,” Tellez says. “The really cool thing here is there’s not many great years of Bordeaux wine. But for every single time the reconstruction error is above that .01 threshold, it corresponds to every single great vintage of Bordeaux.”
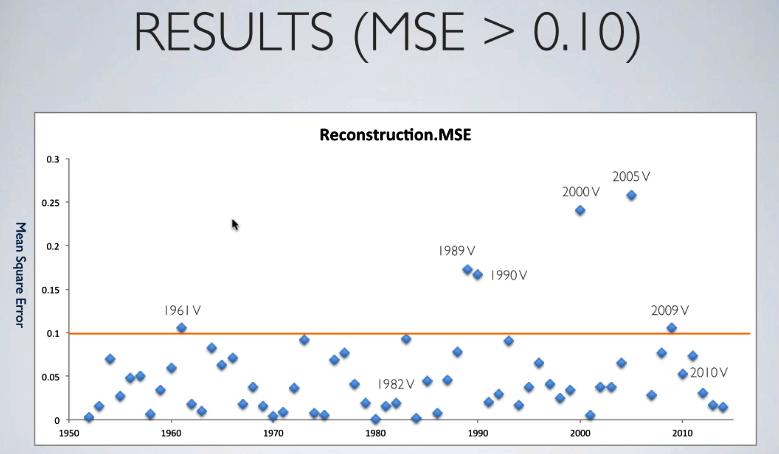
Six of the eight years with great Bordeaux vintages (those with Vs) over the past 60 years positively correlated with a mean square error in excess of 0.01.
Tellez was glad to see that his weather model found correlations between anomalous weather and vintage years in six of the eight great vintages since 1950. “I’ll be the first one to tell you that wine is far more complicated than the weather,” he says. “Each chateau does it their own special way.”
But Tellez may have been even more relieved that the model didn’t make the opposite error: flagging non-vintage years as being outliers in his historical weather model. “For neural networks in general, you obviously want to use a lot more data than 60 years I have here,” he says. “But it’s a fun experiment to do because you actually see the power of what it’s doing in these reconstruction errors.”
This exercise was not entirely theoretical. Tellez was hoping that machine learning could give him an edge when buying wine before it’s been bottled, or “en primeur,” which is basically a futures market. If he could use weather data to accurately predict whether the 2014 Merlots or Cabernet Franks from Bordeaux would be outstanding, then he could either make a lot of money selling wine, or enjoy great wine at a reduced cost. (The machine did not indicate 2014 would be a great year, by the way.)
“We’re trying to beat the wine snobs at their own game,” Tellez says. “I have no clue what goes on behind closed doors of wine shops. That’s their business and of course they don’t advertise that….But I was hoping to come up with a [weather-based] prediction of what are the amazing vintages, so you and I can invest in those Bordeaux wines at the en primeur stage, as opposed to waiting until it’s bottled.”
Tellez is hoping to expand the study and build an even smarter algorithm that factors in additional weather data, such as dew point and number of consecutive days without rain (the drier the weather, the more concentrated the juices in the grapes), with the goal of achieving 100 percent classification of the great vintages of Bordeaux. He’s also considering using the model to classify other wine growing regions, such as Napa or Washington State.
There are a lot of variables that go into making good wine, and not all of them are within our control. The variation from year to year is part of the allure of the wine trade. But as H2O’s Tellez demonstrates, a machine can be as accurate as the wine snobs at predicting great wine, at least as far as the weather is concerned.
Related Items:
The Rise of Predictive Modeling Factories
‘What Is Big Data’ Question Finally Settled?