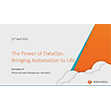
Data Startup Targets Machine Learning for Healthcare
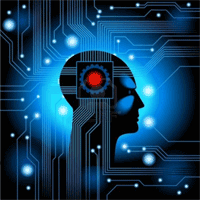
A medical diagnostic startup is attempting to use recent advances in machine learning as a way to make it easier for doctors to sort through medical information in the form of images, unstructured data like notes on a patient’s history and structured laboratory test results.
“Medical diagnostics is, at its heart, a data problem,” notes Jeremy Howard, founder and CEO of Enlitic, a San Francisco-based startup that wants to use machine-learning technology to transform diagnostic healthcare.
“Recent applied machine learning breakthroughs, especially using deep learning, have shown that computers can rapidly turn large amounts of data of this kind into deep insights, and find subtle patterns,” added Howard, who previously served as chief scientist at the data science startup and community Kaggle.
Enlitic has so far rounded up a small team of data scientists with specialties in computational neuroscience, distributed machine learning, cognitive systems and applied deep learning. It is still hiring.
As physicians are deluged with diagnostic data, Enlitic thinks it can leverage machine learning to, for example, develop new deep-learning algorithms that can be used to teach machines to recognize patterns. The tools could be used to flag images warranting further examination by physicians, thereby saving them time pouring through multiple images.
In one use case, physicians could use an Enlitic tool to scan databases for a specific illness.
Enlitic is part of a growing wave of big data efforts aimed at using data science techniques like machine learning to improve health care outcomes. Hospital networks have, for example, started using data analytics to identify cardiac patients at greatest risk while reducing the number of readmissions. Those big data initiatives are tied to cost-saving and resource-conserving efforts.
But observers note that federal laws aimed at protecting the privacy of healthcare data could limit the role of big data in healthcare. Indeed, critics are complaining that data brokers may have greater access to medical data than do patients.
Undaunted, startups like Entilic are looking for ways to use advances in areas like pattern recognition algorithms to improve medical diagnoses. The startup argues that physicians currently lack the tools needed to leverage medical data, particularly automation tools that use pattern recognition algorithms to, for example, characterize disease profiles.
To deliver these insights to physicians, Entilic is trying to leverage recent machine learning advances, especially deep learning, that have combined algorithmic analysis of images, natural language and structured data. IBM’s Watson cognitive computing platform is just one example of how machine-learning advances are being applied in problem-solving systems.
The startup said its goal is to develop software that will advance the start-of-the-art in machine learning and apply those advances to diagnostic healthcare.
Entilic offered no timelines for when it might release its first product. The startup did say it is looking to hire more machine-learning specialist along with data scientists and GPU programmers.
Recent items:
Big Data Faces Big Barriers to Adoption in Healthcare
Big-Data Backlash: Medical Database Raises Privacy Concerns