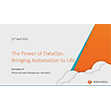
Humanitarian Project Culls Data to Forecast Disasters
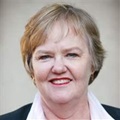
A research project sponsored by the National Science Foundation is attempting to apply the tools of big data to humanitarian efforts like forecasting force migrations of populations whether by natural or man-made disasters.
One goal is to leverage data analytics to forecast humanitarian disasters in an attempt to mitigate the consequences of mass displacements caused by conflicts or disasters. “Early warning has been the holy grail of the humanitarian community for decades,” Jeff Crisp, senior director for policy and advocacy at Refugees International, told a recent NSF advisory meeting.
The advisory group includes technical experts in areas like data retrieval and risk management as well as experts on displaced populations and immunologists. One goal is to create an interdisciplinary community of computer and social scientists. Another is boosting the capacity of humanitarian organizations to analyze early warning indicators.
Ultimately, the project seeks to use big data “to test the feasibility for creating an early warning system to detect forced migration in the context of humanitarian crises,” said Elizabeth Ferris, co-director of Brookings-LSE Project on Internal Displacement at the Washington-based think tank, the Brookings Institute.
The NSF-funded project is based on analysis of a unique dataset called “Raptor” maintained at Georgetown University in Washington, D.C., which includes more than 600 million data points. The data were compiled through daily scraping of more than 20,000 newspaper sources in more than 45 languages, including local, national, regional and international sources. Initially, the dataset did not include US-based media sources, Ferris noted.
“That’s a lot of data,” she added in a blog post. “The computational scientists are figuring out not only how to retrieve useful data from all those newspaper articles, but how to identify what is important amid all the noisy data?”
One issue is the reliability of data based on the news sources being culled. One reason is that widely-circulated rumors, especially via unfiltered social media, can trigger a refugee exodus.
“Perceptions may matter more than the truth, an observation reflected in the project’s use of the Dread Threat concept,” Ferris added. “It’s not just an objective, observable phenomenon that constitutes a threat, but also the perception of the threat that can act as a driver of migration.”
Among the issues that big data analytics could address is how people respond when they are exposed to violence or other threats. Will they defend themselves or flee? It appears that in cases such as the Middle East, especially in war-torn Syria and, now, Iraq, entire towns and villages are fleeing sectarian violence that is real, not perception.
“I wonder if big data can provide a better way not only of understanding how and why people decide to flee from their homes, but of forecasting when and where they will move,” Ferris wrote. “If we ever get to that point, that were be a whole different set of issues which will need discussion.”