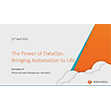
Collaborative, Data-Intensive Science Key to Science & Commerce Challenges
Data-intensive science has the potential to transform not only how we do science but how quickly we can translate scientific progress into complete solutions, policies, decisions and, ultimately, economic success. It is clear: the nations that can most effectively transform tons of scientific data into actionable knowledge are going to be the leaders in the future of science and commerce. Furthermore, creating the required new insights for complex challenges cannot be done without effective collaboration. Experts from many fields must be enabled to work together more seamlessly across discipline, organizational and geographical boundaries in data-intensive environments. And, because many science domains already are unable to explore all of the data they collect (or which is relevant to their research), progress in collaborative, data-intensive science is the key to unlocking the potential of big data.
The big, global grand challenges of big data
The U.S. National Academy of Engineering has outlined today’s grand challenges associated with big data to include: make solar energy economical, provide energy from fusion, develop carbon sequestration methods, advance health informatics, engineer better medicines, and secure cyberspace. But they know these challenges only will be achieved by engaging broad, tightly interacting, multidisciplinary, geographically distributed teams. The speed at which these teams can make new discoveries and translate scientific progress into viable solutions, policies and decisions ultimately will determine economic success. Time-to-solution will be determined by the ability to communicate theories, hypotheses, methods and results more effectively, and also by the ease of access to an advanced research infrastructure and the resulting body of knowledge represented in publications, patents and data.
The evolution of the big data conundrum
In 2008, the European Strategy Forum on Research Infrastructures, or ESFRI, roadmap recognized, for the first time, the importance of an underpinning infrastructure consisting of integrated communication networks, high-performance computing and digital data repository components. The ESFRI roadmap further stated that data in its various forms (from raw data to scientific publications) will need to be stored, maintained and made available and openly accessible to all scientific communities to ensure future sustained scientific progress. The influential 2009 publication ‘The Fourth Paradigm’ declared data was now seen as the emerging fourth pillar of science after observation, theory and computational prediction. In 2010, the U.S. reiterated this sentiment by issuing the America Competes Reauthorization Act focusing on the necessity to make the results of publicly funded research accessible to all in order to further U.S. scientific progress and prosperity.
Since then science and academia have come a long way, and data has universally been accepted as a critical ingredient. Conferences, journals and online publications such as Datanami have emerged in response to a universal desire to understand better how to explore the inherent value in data most effectively. A recent article, ‘When all science is data science,’[i] highlights the pervasiveness of the need for better approaches to dealing with data in all areas of research, business and industry.
But the primary driver of the need for better data analysis, storage and capabilities, since the beginning of the global data discussion, is the volume and velocity of the data that has emerged. Recent studies[ii] [iii]have shown that the rate of data increase far outstrips the rate of computer and storage hardware capabilities (Moore’s Law). We can no longer rely on hardware improvements alone to cope with increasing data amounts, but need to develop fundamentally new mathematical and computational approaches to gain insight from growing data volumes – data-intensive science. And while we must seek these insights often through multi-disciplinary, geographically distributed teams and research facilities, we in the data-intensive science community also need to focus strongly on the collaborative aspects required by modern scientific knowledge and discovery.
So what have we achieved in data-intensive science since 2008? Europe has funded a series of research programs focused, in particular, on long-term data curation – including policies which keep data accessible and usable, as well as data policies and data annotation standards. The recently established international Research Data Alliance, or RDA, is aiming to standardize approaches in this domain on a worldwide level to enable the creation of compatible, collaborative, data-intensive infrastructures around the world. Industry has focused on new, often Hadoop-based, divide and recombine solutions for the deep analysis of data at scale, as well as new, scalable data mining and warehousing methods. New hardware appliances are emerging specifically designed to satisfy the needs of data-intensive analytics.
The U.S. has focused its efforts on the development of underpinning new mathematical and computer science approaches to data analysis and collaboration at the extreme scale in support of accelerated scientific discovery, such as efforts supported by the Department of Energy’s (DOE) Advanced Scientific Computing Research (ASCR) office.
DOE’s Pacific Northwest National Laboratory started its Data-Intensive Computing Initiative in 2006, focused on the creation of tools and capabilities to address the data overload challenge in the fields of bioinformatics, energy and cyber analytics. However while individual technologies for data-intensive science have emerged, few have ventured to integrate these into comprehensive tool sets or complete collaborative infrastructures, with a few notable exceptions. A new initiative called the European Data Infrastructure, or EUDAT, is building a collaborative data infrastructure with the intent to create a distributed, collaborative data management infrastructure to ensure a coherent approach to data access and preservation. In the U.S., the DOE’s Office for Biological and Environmental Systems Research (BER) is establishing the Systems Biology Knowledgebase (KBase), a collaborative effort designed to accelerate the understanding of microbes, microbial communities and plants. It will be a community-driven, extensible and scalable, open-source software framework and application system. KBase will offer free and open access to data, models and simulations, enabling scientists and researchers to build new knowledge and share their findings.
The key components of successful, collaborative, data-intensive science
Are we there yet? No; collaborative, data-intensive science is still a very young field of science. While the field has made great strides over the past few years, critical challenges remain which can, when done right, unlock the potential of collaboration with big data:
• High-capacity, reliable networking – While the network layer is often simply assumed as a “given” by most science teams, and even most programmers, ensuring it continues to scale will be critical to the success of future collaborative, data-intensive science efforts, as it provides the vital connectivity and access to people, experimental, observational, computing and data resources.
- Semantics – Understanding the semantics of the data, specifically how using the data to define the ontology provides an effective approach for defining the underlying concepts and relationships. Federated semantic search and hypothesis creation are further open research topics.
• Curated, active data appliances – Standards-based, long -term, domain-specific data repositories are critical. These repositories will offer the ability to analyze data in situ and synthesize it easily with researchers’ new findings, which also are connected with other repositories to enable inter-and cross-disciplinary data analysis and discovery across different data repositories.
• Data analysis – In situ/real-time analysis and interpretation of streaming data, deep analysis of large data volumes and the ability to synthesize data from heterogeneous and potentially geographically distributed sources in highly dynamic scientific domains, is critical.
• Provenance at scale – Having a clear, understandable and easily consumable (by human and machine), data provenance chain is critical to establishing the trust required to effectively share information between science teams.
• Scientific discovery – How can we rebalance the interaction of instruments, computers and humans in new ways to make scientific discovery in extreme data more effective, and what role can artificial intelligence play in aiding discovery? Can we move from question answering (with technology such as IBM’s Watson) to hypothesis generation and ranking based on evolving scientific data.
Currently, much of this research is disjointed. Advances are being made in individual research communities. However, there is little being done to pull together all of these advances into overarching, collaborative, data-intensive solutions that could be adopted and customized by science teams. Even in cases where there is clear overlap of capability, for example when the provenance of in situ data analysis results is critical to the data’s acceptance, there is little cooperation between the leading researchers in each field.
We believe this will change over the coming years as funding agencies and their user communities are starting to develop visions for complete, integrated, collaborative data-intensive environments such as those described in a recent DOE BER Advisory Committee report for a BER Virtual Laboratory[iv] . In short, a framework that would allow the ‘seamless integration of multi-scale observations, experiments, theory and process understanding into predictive models for knowledge discovery’ is key to unlocking the potential of collaborative, data-intensive science.
About the Author
Dr. Kerstin Kleese van Dam is the associate division director for Computational Science and Mathematics as well as lead of the Scientific Data Management group at the U.S. Department of Energy’s Pacific Northwest National Laboratory. She has led collaborative data management and analysis efforts in scientific disciplines such as molecular science (e-minerals), materials (e-materials, materials grid), climate (PRIMA, NERC Data Services, DOE’s climate science for a sustainable energy future), biology (DOE’s Bio Knowledgebase Prototype Project, integrative biology) and experimental facilities (ICAT, chemical imaging). Her research is focused on data management and analysis in extreme-scale environments.
Kerstin has extensively published in the domain of data intensive science, including the newly released Data-Intensive Science, jointly edited with Terence Critchlow (late May 2013). In the book, a diverse cross-section of leading application, computer and data scientists explore the impact of data-intensive science on current research and describe emerging technologies that will enable future scientific breakthroughs. In the past, she has also contributed to other publications on this topic, such as Handbook on Data Intensive Computing (2011) and Data-Intensive Computing (2012).
[i] Venkatraman, Vijaysree. “When all science is data science.” Science Magazine, June 2013. http://sciencecareers.sciencemag.org/career_magazine/previous_issues/articles/2013_05_13/caredit.a1300099
[ii]Xun, Li and Chong, Frederic T. “A Case for Energy-Aware Security Mechanisms.” http://www.cs.ucsb.edu/~xun/papers/greensecurity-EASyCoSe13.pdf. University of California, Santa Barbara, 2013.
[iii] Kosar, Tevfik, Ph.D. “Wide Area Distributed File Systems.” Jan. 2013. http://www.cse.buffalo.edu/faculty/tkosar/cse710_spring13/slides/Introduction.pdf
[iv] Biological and Environmental Research Advisory Committee, U.S. Department of Energy. “BER Virtual Laboratory:
Innovative Framework for Biological and Environmental Grand Challenges.” Feb. 2013. http://science.energy.gov/~/media/ber/berac/pdf/20130221/BERACVirtualLaboratory_Feb-18-2013.pdf
Related Items:
Software Development Strategies for the Age of Data
On Algorithm Wars and Predictive Apps
Foreman: Don’t Forget the “What” and “Why” in Big Data