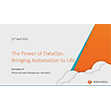
A New Model for Big Energy Data
In our homes, monitoring energy use is usually a matter of taking precautions to turn off lights and devices to keep usage down, particularly during peak. However, for large businesses—and even the utility providers—managing energy consumption is a critical operational task.
As one might imagine, massive, complex silos of data are required to thoroughly understand the energy grid at both the consumer and utility level. The energy management and provision industry encapsulates all of the “big V” concepts underlying most conversations about big data, with increasing emphasis on the velocity aspect as speed is needed to help businesses and utility reshape their usage in real-time to respond to pricing and peak demands.
For a company that seeks to let large-scale energy consumers understand and strategically alter their usage in near real-time, the volume piece of the “big data big Vs” is the easiest to spot—from smart meters to building management systems, voltage regulators, thermostats and the range of other sensors used within any large building, data sources are in no short supply.
One such company that seeks to let software solve the challenges of demand response and usage monitoring for load shifting is California-based AutoGrid. The company claims its Energy Data Platform can make use of complex predictive models across terabytes of smart meter and other energy monitoring data in much the same way that supercomputers are used to model weather for prediction purposes.
According to AutoGrid, there are around 50 million smart meters installed. Further, many large consumers of electricity have installed next-gen building management systems that track lighting, AC, and electrical outlet loads, among other power points. What this means for companies trying to help companies maximize the use of these systems is a lot of data—a fact that is useless without a sophisticated way to make use of it in the context of the overall grid.
AutoGrid notes that a single message from a smart meter to a utility might amount to a few kilobytes, but smart meters send a continual stream of information. “Five million smart meters sending updates every 15 minutes will produce nearly 16 terabytes of information a year, a 3,000-fold increase in the amount of data the same utility would process today by some estimates. The same number of meters collecting data every minute, which will give utilities a more accurate picture of power consumption, will produce 240 terabytes in a year.”
To make good use of the data, users, energy providers and demand response companies require advanced models that can put real-time and predictive context to these data. These models are not just focused on the grid as it relates to consumers and they go beyond just looking at real-time usage. Large utilities can mine their own consumption patterns to create predictive models to better understand usage across the grid and adjust their offerings accordingly. Further, demand response companies can tap into the same modeling tools to increase their power yields. For the average large-scale consumer of electricity, these models—predictive and otherwise—can help them create solid usage plans that dash around peaks and valleys with the potential for massive savings and a greener presence.
According to CEO, Amit Narayan, the petabytes required to model the grid aren’t a problem. With so many sensors and data types, meshing these data together into a coherent whole represents a critical challenge. Add to that the ability to offer near real-time modeling of the energy grid for energy consumers and large utilities alike and the problems are more complex. The combination of data volume, variety and velocity forms the right conditions for Narayan to, as he noted, “look to high performance computing (HPC) techniques” in addition to some widely used “big data” tools of the trade to achieve massive parallelism.
Narayan is no stranger to the energy or high performance computing arenas, both in terms of his research and enterprise interests. He maintains his post at Stanford University where he serves as Director of Smart Grid Research in Modeling and Simulation, working on many of the same concepts that found their way into AutoGrid. Narayan is also currently leading an interdisciplinary project that aims at modeling, optimization and control of the electricity grid and the companies behind it.
“What we are doing now has many elements from HPC,” said Narayan. “We have to use those same techniques to solve the many physics-based optimization problems we encounter, and to deal with the data tsunami from many sources. To process that in near real-time we have to use HPC to optimize and figure out very quickly what to do next.”
He described in detail about the complexities of modeling the grid—a process that is similar, both in terms of data complexity and size, to weather modeling. While weather models and forecasts are driven by supercomputing resources, however, their team uses Amazon’s EC2 infrastructure to deliver a software-as-a-service (SaaS) model, which he says lets utilities or large companies take advantage of demand and energy pricing without investing in so much heavy-duty infrastructure that it makes any potential for savings entirely unworthy of the investment.
MapReduce is at the heart of AutoGrid’s modeling approach. As Narayan noted, “when we generate models for end users we use MapReduce to parallelize that computation, but then we have to figure out, for example, the effect of load for end points within the rest of the grid. At that point we have a large-scale equation and MapReduce is not the right approach for parallelizing that computation so we have to look to other HPC techniques.
The company uses MapReduce for its batch processing while other techniques (which he chose not to disclose) power the stream processing end of the business. He said that the benefit of this dual approach is that they can do certain computations, namely millions of forecasts, in near real time.
The cloud model allows the company to offer a service that costs users only time and some expertise to manage the software. Such a setup involving MapReduce, Hadoop, and stream processing would require an advanced team of on-site experts. This freedom from hardware is the root of the company’s claim that it can become “a new source of energy” since so many smart metering initiatives don’t go far enough beyond mere pricing optimization.
According to the company, the data they make use of gives both consumers and power producers their “first opportunity to truly track power consumption. Unfortunately, these entities don’t have the computer systems, time or budget to fully exploit this data.”