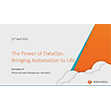
One Giant Leap for Psychohistory
For science fiction buffs, many news items coming out of the world of predictive and large-scale historical analysis invoke Asimov’s concept of Psychohistory, in which probabilistic group patterns can predict major future events in history.
While big data platforms today may not be able to predict the eventual fall of Galactic Empires (although predicting revolutionary events from social data is a reality) they can generate insights based on large swaths of historical data.
In particular, Kalev Leetaru from the University of Illinois carried out a fascinating historical analysis project where he mapped “the world according to Wikipedia” SGI’s UV2, a system which the company has touted as “the world’s largest in-memory data mining system.” Leetaru explains the genesis of the project below.
Leetaru had already used similar analytics in publishing his Culturomics 2.0, where he, according to SGI, predicted the Arab Spring and the location of bin Laden’s hideout. When he was approached by SGI’s Michael Woodacre about the new UV2 system, which would apparently carry 4,000 processors and 64,000 terabytes of cached coherent shared memory, he thought immediately of Wikipedia. “Wikipedia,” Leetaru said “has become such a fundamental part of our daily life. What could we do if we made a map of this or a series of maps over time?”
So Leetaru set out to model the world according to Wikipedia’s English-Language edition. The task itself is simple to comprehend, essentially Leetaru wanted to mark down every mention of a name, date, or place found in Wikipedia.
“We used this UV2 system to pull out every geographic location across every page,” said Leetaru “every date across every page, and every connection among those, basically capturing the spatial and temporal view of history as captured by Wikipedia’s pages…We can actually see history before our own eyes.”
Of course, there are over four million entries in the English version of Wikipedia, each of which have multiple references to any given date, place, or name. If those references are the neurons of Leetaru’s project, the connections are the synapses. Leetaru had to deal with and analyze one heck of a historical neural net.
UV2’s impressive in-memory capabilities made this possible for Leetaru. “I didn’t spend hours or days writing some fancy code that was distributed memory or using any of these fancy extensions, having to worry about memory management, allocating the right buffer sizes. I just wrote a ten line Perl script in a matter of minutes and just ran it… If I had to summarize the advantage of the UV2 platform in a single sentence, I think it would be ‘Outcomes over algorithms.’”
The outcome is represented in a fascinating infographic on SGI’s Facebook page, which goes over number of date mentions per year, sentiment over time and much more. For example, the sentiment over time graph shows sharp dips around the 1860s, 1910s, and 1940s. Those dips correspond with the American Civil War (the sharpest dip, perhaps shedding some light on the American bias in English-language Wikipedia articles) and both World Wars.
There are plenty more insights to be gleaned and plenty to be extrapolated. Leetaru’s research shows that the world has become exponentially more interconnected over the last fifty years. This connectivity makes it easier to digitalize human patterns and apply data analysis to them. Perhaps Asimov’s psychohistory is not thousands of years away after all.
Related Articles
MapReduce Makes Further Inroads in Academia
In-Memory Tweaks Boost Proteomics Research
Researchers Germinate Novel Approach to Big Bio Data