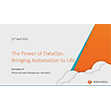
A Big Data Revolution in Astrophysics
Humanity has been studying the stars for as long as it has been able to gaze at them. The study of stars has to led to one revelation after another; that the planet is round, that we are not the center of the universe, and has also spawned Einstein’s general theory of relativity.
As more powerful telescopes are developed, more is learned about the wild happenings in space, including black holes, binary star systems, the movement of galaxies, and even the detection of the Cosmic Microwave Background, which may hint at the beginnings of the universe.
However, all of these discoveries were made relatively slowly, relying on the relaying of information to other stations whose observatories may not be active for several hours or even days—a process that carries a painful amount of time between image and retrieval and potential discovery recognition.
Solving these problems would be huge for astrophysics. According to Peter Nugent, Senior Staff Scientist of Berkeley’s National Laboratory, big data is on its way to doing just that. Nugent has been the expert voice on this issue following his experiences with an ambitious project known as the Palomar Transient Factory.
The research endeavor is a collaborative effort between Cal Tech and Berkeley to study the formation of various astronomical phenomena. Groups used the telescope on Palomar Mountain in attempts to detect planets beyond our solar system, dwarf novae, core collapsed supernovae, blazars (super compressed quasars which suggest the existence of the supermassive black holes at the center of galaxies), and neutrino emissions among other things. The ‘transient’ in the group’s name is meant to denote that the discoveries are meant to be new, emerging, or moving, essentially in transition. Nugent’s group’s specific focus was on identifying new type a1 supernovae.
The main focus, however, was not on the discovery of the phenomena but rather on the development of a machine learning system that could quickly identify worthy candidates. The process of identifying worthy candidates is straightforward. When an image is processed of a certain area of space, it is compared to an earlier image in a database of the exact same area of space. If the differences, or ‘subtractions,’ meet a certain threshold of significance, the transient is submitted for closer examination to the humans, where the people take their turn at classifying the potential phenomenon.
Over around 800 operational days, 1.8 million images were taken. Those were turned into 32,000 models of a particular portion of a sky, or references. 1.4 million subtractions were performed, leading to 900 million discovery candidates and 45,000 transients (candidates that made it past the machine’s vetting process). Every single item was stored. It is not difficult to see where this data got big.
Usually, the individual transient is discarded. Several factors, including time of day, cloud cover, and the amount of incoming starlight at a particular time, can lead to ‘significant’ readings that are not at all significant. The goal, simply, is to get the machine to classify better than the humans so the humans may only be bothered by the most serious of candidates.
In true scientific fashion, the project’s first major discovery was made when everything possible went wrong with the computing system. According to Nugent, Cal Tech had changed a key IP address, the main programmer was in Israel and the entire process had to be done by hand. As it turned out, Nugent found his emerging type a1 supernova, occurring only hours before (plus the amount of time it took for light to travel there).
However, that debacle pointed out areas of weakness and the system improved. According to Nugent, it is actually outperforming the humans in both speed and accuracy. While the improvement in accuracy is perhaps a tad unexpected, Nugent notes that the computer will never be classifying phenomena two hours after returning from the pub.
As a result, three or four emerging type a1 supernovae or other similar-scale astronomical events are found each day according to Nugent. Contrast this with the beginning of the project, when it was a big deal when Nugent found the first one. Such a discovery rate is truly remarkable and could significantly advance the world (or universe) of astrophysics.
That would be especially true if the PTF can accomplish its future goals in improving their survey. On the docket for Nugent is a five-fold expansion of the range, image time intervals of 100 seconds, and a candidate list turnaround of 10-20 minutes. However, since each image is stored and analyzed, the data requirements would obviously be significantly higher, a difficult proposition for a survey that used 175 out of 250 purchased terabytes.
Indeed, according to Nugent, it is from image processing to candidate generation that potential bottlenecks would occur. The data, having been sufficiently whittled down, would easily move to classification and beyond from there.
Astronomical discoveries are always exciting, even for the astrophyscs “uninitiated”. They give us a sense of where we stand in the universe and insight into our beginnings. Nugent and PTF are working to accelerate these discoveries using big data analytics and their preliminary results are promising.
Related Stories
World’s Top Data-Intensive Systems Unveiled
NASA Resource Brings Big Science Data Home
Supercomputing Center Set to Become Big Data Hub
Astronomers Leverage “Unprecedented” Data Set