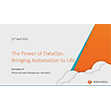
How Magic is the Big Data Storage Bullet?
If we let the buzz make the big technology decisions, it’s time to throw a superhero cape around the shoulders of the bounding, bright elephant and call Hadoop the official IT superhero of the coming decade.
There are many warning that Hadoop is certainly not the magic bullet for the new era of big data, including those who suggest that the core of the problem with Hadoop is that everyone is being rushed into adoption due to the iconic status the platform has received as of late (a point well-argued here)–all without understanding how the fundamental nature of the problems to be solved can truly benefit from Hadoop.
The problem isn’t just a matter of over-hype versus under-understanding. At the high level, it is relatively easy to get started using it without an in-depth knowledge of what gives it its powers and without this, you are more likely than not to design your solution in a way which takes all of those supposed powers away and actually creates datacenter inefficiencies.
Those points aside, there is still an increasingly noisy camp (which gets louder as more millions are thrown in the direction of Hadoop) suggesting that it can solve all problems the datacenter, not the least of which is storage.
According to BI consultant Frank Ohlhorst, in some cases, Hadoop can offer that much-hyped magic bullet for storage woes as “the platform solves the most common problem associated with big data; efficiently storing and accessing large amounts of data.”
Ohlhorst says that storage is still evolving, in part due to the big data influx. Still, while it’s moving toward becoming less of a financial burden for enterprises, the technologies are strained to their limits with data growth and the need to rapidly make use of the onslaught.
He writes that “traditional storage technologies, such as SANs, NAS and others cannot natively deal with the terabytes and petabytes of unstructured information that come with the big data challenge.”
The argument in favor of Hadoop is that it helps to remove much of the management overhead associated with large data sets. Operationally, as an organization’s data is being loaded into a Hadoop platform, the software breaks down the data into manageable pieces, which are then automatically spread across different servers. The distributed nature of the data means there is no one single place to go to access the data. Hadoop keeps track of where the data resides, and further protects that information by creating multiple copy stores. Resiliency is enhanced, because if a server goes offline or fails, the data can be automatically replicated from a known good copy.
Ohlorst gives a nod to the fact that this is not a “magic bullet” approach for solving big data woes. As he says, “Not all enterprises require the capabilities that big data analytics has to offer. However, those that do must consider Hadoop’s capability to meet the challenge. But Hadoop cannot accomplish everything on its own — enterprises will need to consider what additional Hadoop components are needed to build a Hadoop project.”
In addition to admitting that this a perfect solution for some projects, some of the time, Ohlhorst notes that there is still no jumping in feet first with Hadoop—a great deal of thought and planning is required. As he writes, “All things considered, an in-house Hadoop project makes the most sense for a pilot test of big data analytics capabilities. After the pilot, a plethora of commercial or hosted solutions are available to those who want to tread further into the realm of big data analytics.”
Related Stories
Inside LinkedIn’s Expanding Data Universe
Half the World’s Data to Touch Hadoop by 2015?
Six Big Name Schools with Big Data Programs