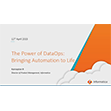
Inside LinkedIn’s Expanding Data Universe
It’s no secret that the reason our beloved social networking platforms are free is because of the sheer value of the data we provide. But beyond providing the info points to help the platform connect you to your peers, your LinkedIn data is being used to make some rather remarkable assessments about the present, not to mention some revealing predictions about the future.
While we will shed some light on what a few of those macro-insights from big social data are (and what tools were used to concoct them) in a moment, it seemed prudent to take a step back first and get the big picture view of LinkedIn…More specifically, how it makes use of what little data users are requested to provide to join its still-expanding network of over 150 million other linked professionals worldwide.
Few are better positioned to speak to the data and macro-insight point than the company’s team lead and senior data scientist for LinkedIn Analytics—not to mention trained economist—Scott Nicholson. We spent some time with Nicholson this week during the Informs Analytics conference where scads of “quants” in operations research have gathered to talk stats, optimization, and big data.
Nicholson’s background as both economist and data scientist have put him in good company here at the Informs event. In addition to a crash course in the tools, infrastructure and data vision that his team operates under, he also shared some compelling visualizations (coming in a sec) that show what’s possible on the micro and macro-economic analysis level when you have user-submitted data on a range of professional life data points in the many millions.
Instead of just seeing LinkedIn for what it is—a social platform—Nicholson says that what it really does, for both users and LinkedIn, is allows an individual to see his or her place within the entire economic universe. Or, for that matter, to zoom in and view the microcosom of their profession, skillset, network, or their job outlook. “We have a view that no one else has,” he said. “We have data that lets us understand what actions people take professionally, and then we take that one step further to see how we can personalize their experience on LinkedIn based on that behavior.”
According to the data scientist, the company is endlessly toying with different ways to make its users interact with the site. He says it’s simple to compel users to make decisions using simple tools and data at the massive scale to predict what will be useful—what will lead to the continual click-throughs.
And this is really the name of the game for a social platform’s business—just as companies that pitch tangible goods want their product to resonate, LinkedIn wants to create a data product that is sticky—one that keeps a user coming back each day.
But what hides behind the vanity of our everyday visit is the data power of an economic and job market powerhouse, not to mention a robust capacity to lump us all into our own professional universes for more analysis.
NEXT — Your Personal Universe. Visualized…>>>
Your Personal Universe. Visualized.
On the macro level, the personalization of connection suggestions, news, and job possibilities is only the beginning. After all, for many profiles, it’s some educational and work history coupled with current location and a listing of some skills.
However, when you put that data together against the background of millions of others, users are able to, as Nicholson says, “see where they personally fit within the entire economic universe.”
By “see” Nicholson is not simply referring to the concept of understanding their position relative to the greater world of work through how many connections one has—he means this literally. Via the company’s partnership with visualization firm Tableau, it’s possible to see entire segments of the professional universe.
The following three slides are some examples of what is possible with 150 million people offering up a steady, updated stream of personal professional data. But just to get us started, below is one of the first visualizations across the network we wanted to share.
With the help of Tableau’s visualization software, LinkedIn has created a number of mind-boggling viz works that let users see the entire LinkedIn network, but more important, to zoom in and view these on a microcosm level.
I’ll let LinkedIn’s chief scientist explain more about how this works if you’re interested in peering into the complex mess that is your worldwide connection sphere.
These types of views are useful for those looking for an overall sense of what their network looks like and provide a nice “wow” factor visually.
However, what is most useful is when these visualization capabilities are fine-tuned to take a close look at a certain industry or set of skills.
NEXT — Your Skill Universe. Visualized…. >>>
Your Skill Universe. Visualized.
When we look at our LinkedIn profiles and the information we’re requested to provide, presumably so we’ll be more apparent to potential employers (or stalkers, of course), our skills are featured prominently.
LinkedIn uses this data to make assessments not only about the particular demand for the skills we’ve stated, but also to view the universe of skills related to those we’ve stated.
This means that the company now has an understanding of what skills are most likely associated with certain people and professions, which in turn allows the recommendation engine to understand that since you fit into the C+ programmer universe, you likely have other skills in that solar system, including C#, for instance.
For LinkedIn, this is valuable information because it allows them to deliver highly targeted jobs, people and news that the user will be interested in now that the pool of skills they’ve become associated with has been “predictively” widened.
According to Nicholson, the same core algorithm that powers their “People You May Know” has been roped into association tasks like these.
NEXT — Your Industry Tanking. Visualized. >>>
Your Industry Tanking. Visualized.
It’s not that we don’t need economists to sit around and conjure up new figures about failing or rising job markets, but there is no doubt that LinkedIn data should play a role in how think tanks consider progress.
For instance, look at the following very simple chart that uses data from the 150 million member stream, complete with updates about jobs lost and gained, that shows job market movement.
On that note, during the same analytics conference this week where we met with Nicholson, Google’s Chief Economist, Hal Varian, talked about how new models with big data, including the use of Google Trends and Google Insights, when layered in with government job and economic data, are putting human economists to some degree of shame.
For instance, in his own display of analytics-beats-human humor, Varian talked this week about the scientific process by which economic decline can be associated with a rise in first free-ad type searches across Google, followed a few months later by a massive entertainment and porn glut as the job-seeking masses simply give up and choose to live in wild abandon and sin.
NEXT — Your Name Equals Your Job. Visualized. >>>
Your Name Equals Your Job. Visualized.
On that humorous note, the following slide from LinkedIn data was one the data science team came up with out of sheer curiosity, according to Nicholson.
This one certainly has the least key economic prediction value of the pack, but it does let LinkedIn data scientists know how to name their offspring to encourage the possibility that someday they will grow up to be a CEO.
The LinkedIn data science team took all the first names across all the broad industries and looked at various roles and leadership positions. The finds were, in a nutshell, that male CEOs in all industries, along with their male sales folk, tend to have short, quip-like names like Chip or Bill.
On the other hand, female CEOs generally eschew these name-shortening tactics and tend to have long names. Case in point? One of the data scientists that Nicholson works with decided to name his new baby Katherine Alexandra—a power-hitter of a name for the little CEO in the making (no Kathy Alex there)…
While this is a fun way to think about what’s possible with a little data scattered across over 150 million people and their associated roles, it showcases a couple of important concepts.
First, despite rapid proliferation, one can argue that there is no junk data. With the inexpensive storage and processing and more mature, robust frameworks like Hadoop and NoSQL approaches, any data can potentially be spun into insight—even if it’s just for meaning-laden entertainment value or baby-naming.
Second, there is no end to the insights that come out of visualizing results. Nicholson has a lot to tell us about what lies behind the curtain to make these insights possible…
NEXT — Behind the LinkedIn Data Science Curtain…>>>
Behind the Curtain: Tools and Infrastructure at LinkedIn
As you can imagine, creating a multi-million user, dynamic, psychic platform all takes some bleeding edge tools and infrastructure. The LinkedIn analytics team relies heavily on a number of open source packages (Hadoop, Pig, R, and so on) as well as proprietary tools, including database technologies from Teradata (the team recently moved off Oracle), visualization mojo from Tableau Software, and also houses an Aster Data cluster.
As a company that got its start in 2003, well before distributed frameworks for handling massive amounts of diverse web data emerged, LinkedIn had to make some swift decisions about how to address its altering data environment. Accordingly, they were one of the first major platforms to declare their work with Apache Hadoop.
Nicholson condenses the tools and infrastructure side of LinkedIn Analytics in the short clip, describing their use of a number of open source packages. Note that during our discussion after his talk, he noted that they are not using R in production, but use it instead for internal modeling.
To build on what he says above, beginning with a pure SQL approach around 2003, LinkedIn had to look to new distributed solutions to address the structured and unstructured data demands, not to mention those required a NoSQL approach. As Nicholson said, the company takes in a great deal of unstructured data from a number of sources, including data from the co-posting relationship it has with Twitter. Additionally, the company’s own groups are the source of thousands of discussions each day, all of which don’t fit neatly into the SQL cabinets that used to suit the company just fine.
While functions on the site like the home-cooked “People You May Know” feature used to be run purely on SQL, adding Hadoop into the mix when it came onto the scene a number of years ago added the right distributed key to the lock and allowed LinkedIn to perfect that technology. While no one we’ve ever talked to from LinkedIn says that they feel Hadoop is a magic bullet for big, complex data, there are certain problems, like this particular recommendation engine (which relies on the in-built Project Voldemort for its efficiency) which are perfect problems for a Hadoop cluster to tackle.
NEXT — The Science of Sticky Services….>>>
The Science of Sticky Services
When it comes to creating a “sticky” web-based service—one that people refresh throughout the day or check at least once per week—only a few have the secret sauce down pat on a massive scale.
On that short list is, of course, Facebook, but for many professionals, another is certainly LinkedIn. But what makes the professional network so sticky, and what would it take to replicate it?
For one thing, the hefty infrastructure we just discussed would be critical to start, but the second key to stickiness is becoming the end-all resource for a certain class of users. For a professional networking site, this means being the completely personalized, highly tailored source for job-related news, potential new business partnerships, a pool of exact-match jobs, and even an ad network that is highly targeted (did we mention that Nicholson’s previous life was in ad targeting?).
To get a sense of what Nicholson means by personalization, open LinkedIn and move over to your own profile to see with new eyes. LinkedIn is using data to tailor every aspect of the user experience so it becomes a daily one-stop point of reference, even going so far to “replace” the newspaper by creating a customized professional newspaper just for a particular user.
Additionally, notice that all the ads are targeted around your skills, the job recommendations are also probably eerily on-spot, and further, the recommendations they’ve presented for people you know really are people you might know (although if you haven’t yet there’s probably a good reason you haven’t connected with them yet).
The zinger here is that the “People You May Know” algorithm, which has become a key component of other “sticky” social sites, like Facebook, for instance, is a flagship algorithm straight out of LinkedIn. As Nicholson said, “this is where a lot of our secret sauce is. It’s our most mature data product, we’re the originators, but we didn’t ever get around to putting a patent on it.”
NEXT — Linking the Chain >>>
Linking the Chain
Although it seems odd at first, Nicholson continually refers to the site features on LinkedIn as “products.” As he described, LinkedIn sees the profiles as the natural resource from which are mined all types of data products.
While the company’s business model wasn’t the topic of discussion, the fact that LinkedIn refers to what most of us would call “website features” as “products” led to some questions from the floor about how it could monetize insights. For instance, given their sophisticated data mining capabilities, couldn’t they allow companies, for a large fee, access to a specific set of candidates that has been custom-selected for them? Or, on a more sinister note, couldn’t they grant access to where a company’s defectors migrated to in advance of a massive string of layoffs?
Or, couldn’t they sell information to companies on the fly that represent the movement of professionals in a certain arena (like banks below) following an industry-wide shakeup?
According to Nicholson, for now, they’ll do projects with economic data value like this as needed in conjunction with entities like the U.S. government, but imagine the business opportunity if your LinkedIn data was for sale.
On that note, he says that he personally is a big fan of openness when it comes to data, but says that so far at LinkedIn there are a number of ways the API could be opened but none are in the works that would allow businesses to layer LinkedIn data on their own for a fee. More important, however, is the concept that one day LinkedIn might share the economic and employment data with the government to layer into its own systems for a few true indications about how the job market is faring. While Nicholson says that’s all great stuff as well, so far it’s all under wraps about any future collaboration of data.
Our thanks to the organizers of the Informs Analytics Conference for having us out and making this valuable session part of their program.
Related Stories
7 Big Winners in the U.S. Big Data Drive
Six Big Name Schools with Big Data Programs
Half the World’s Data to Touch Hadoop by 2015?