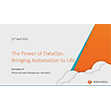
IBM Tackling Hadoop with Machine Data Accelerator
IBM has been fairly visible on the big data front over the last couple of years, putting their analytics to the test in several use cases, including healthcare, state governments, and even the US Open tennis tournament.
Their next challenge is taking on Hadoop and making it more accessible for end users through their Machine Data Accelerator. IBM BigInsights Technical Sales Lead Dirk de Roos discussed how the Accelerator seeks to alleviate bottlenecks in machine log data.
“The Machine Data Accelerator is a set of building blocks to enable people to do machine data analysis or log analysis using our big insights offering,” said de Roos in providing an overview of how the Accelerator relies on IBM’s analytics to tackle massive datasets from machine logs.
For de Roos, big data problems essentially come down to finding patterns in datasets, provided those datasets are about a million to a trillion times bigger than what the end user is used to. “Log data,” de Roos said, “machine data, sensor data, that data tends to accumulate at an incredibly rapid rate, especially nowadays when we have so many sensors and systems that generate these system logs.”
Creating those large datasets are machines and sensors, which today are increasing exponentially in both the amount of data they can generate individually and the total volume of sensors and machines. According to de Roos, Hadoop was built for processing and analyzing said log data. However, there still exists a gulf between Hadoop’s capacity and people’s capacity to work with it. “Hadoop, even in its original incarnation, it was designed for large scale analysis of log data. But even though Hadoop was designed for that, it wasn’t built with out of the box tools.”
To understand how IBM’s technology handles these issues, de Roos first delved into what specifically makes finding statistical patterns in big data difficult. A significant problem, according to de Roos, lies in the diversity of data. Something as simple as timestamp format can throw analytics engines through a loop.
“It’s very important to normalize the data in these log files.” Lack of normalization often leads to errors in MapReduce queries, as the standard Hadoop query function pick up more noise than desirable when there exists a lack of clarity in the data.
IBM’s notion is to build what they call ‘sessions’ of data, which provide more standardization or at least weed out the unclear data. After all, people who are looking at big data are generally looking for patterns and statistical trends and de Roos hopes IBM can help accomplish that.
“What is unique is the ability that we have in the machine data accelerator to build sessions out of fairly big volumes of log data and then do statistical analysis on those sessions. Again it’s unique, it’s interesting from the perspective of people who are looking for patterns and are looking for the needle in the haystack.”
Blocking the noise from the signal has been at the heart of the whole IBM analytics arm, and now they are seeking to apply such techniques to Hadoop.
Related Articles
Preventing Brain Injuries with Predictive Analytics