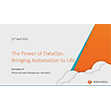
Can Scale Become the ‘Data Foundry’ for AI?
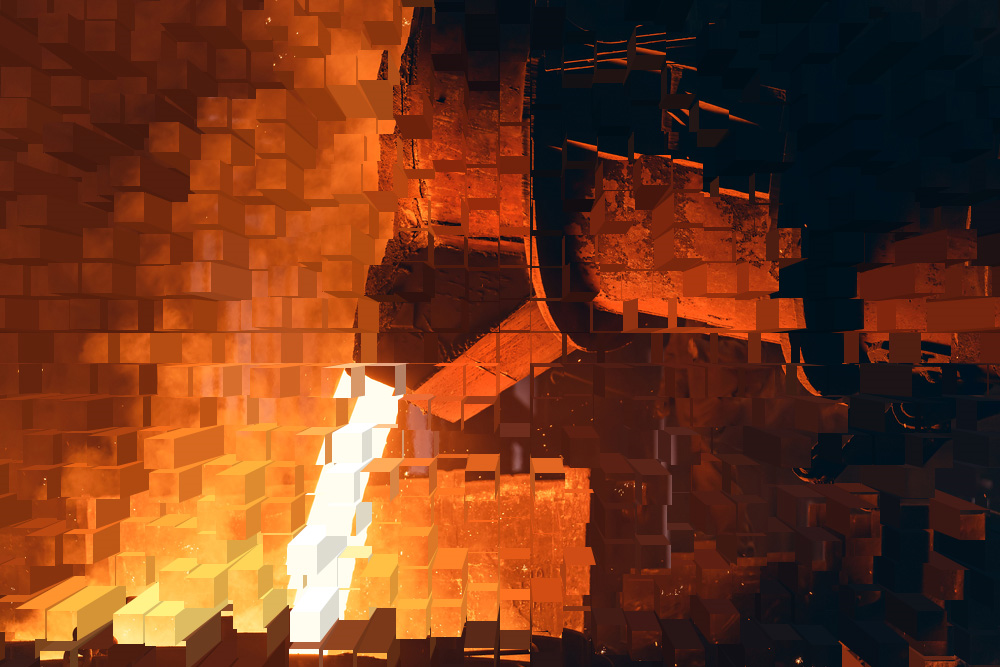
(DedMityay/Shutterstock)
Scale AI, which provides data labeling and annotation software and services to organizations like OpenAI, Meta, and the Department of Defense, this week announced a $1-billion funding round at a valuation of nearly $14 billion, putting it in a prime position to capitalize on the generative AI revolution.
Alexandr Wang founded Scale AI back in 2016 to provide labeled and annotated data, primarily for autonomous driving systems. At the time, self-driving vehicles seemed to be just around the corner, but getting the vehicles on the road in a safe manner has proven to be a tougher problem than originally anticipated.
With the explosion of interested in GenAI over the past 18 months, the San Francisco-based company saw the need explode for labeling and annotating text data, which is the primary input for large language models (LLMs). Scale AI employs a large network of hundreds of contractors around the world who perform the work of labeling and annotating clients’ data, which involves things like describing pieces of text or conversation, assessing the sentiment, and overall establishing the “ground truth” of the data so it can be used for supervised machine learning.
In addition to providing data labeling and annotation services, Scale AI also develops software, including a product called the Scale Data Engine that is geared toward helping customers create their own AI-ready data–or in other words, to create a data foundry.
The Scale Data Engine provides a framework for the “end-to-end AI lifecycle,” the company says. The software helps to automate the collection, curation, and labeling or annotating text, image, video, audio, and sensor data. It provides data management for unstructured data, direct integration with LLMs from OpenAI, Cohere, Anthropic, and Meta (among others), management of the reinforcement learning from human feedback (RLHF) workflow, and “red teaming” models to ensure security.
ScaleAI also develops Scale GenAI Platform, which it bills as a “full stack” GenAI product that helps users to optimize their LLM performance, provides automated model comparisons, and helps users implement retrieval augmented generation (RAG) to boost the quality of their LLM applications.
It’s all about expanding customers’ ability to scale up the most critical asset for AI: their data.
“Data abundance is not the default; it’s a choice. It requires bringing together the best minds in engineering, operations, and AI,” Wang said in a press release. “Our vision is one of data abundance, where we have the means of production to continue scaling frontier LLMs many more orders of magnitude. We should not be data-constrained in getting to GPT-10.”
This week’s $1 billion Series F round solidifies Scale AI as one of the leaders in an emerging field of data management for GenAI. Companies are rushing to adopt GenAI, but often find their data is ill-prepared for use with LLMs, either for training new models, fine-tuning existing ones, or just feeding data into existing LLMs using prompts and retrieval-augmented generation (RAG) techniques.
The round includes nearly two dozen investors, including Nvidia, Meta, Amazon, and the investment arms of Intel, AMD, Cisco, and ServiceNow. The $13.8 billion is nearly double the $7.3 billion valuation Scale AI had in 2021, and puts the company, which reportedly had revenues of $700 million last year, on track for an initial public offering (IPO).
Scale AI has worked with a range of companies, including iRobot, maker of the Roomba vacuum machine; Toyota, Nuvo, Amazon, and Salesforce. It signed a $249-million contract with the Department of Defense in 2022, and it’s done work with the United States Airforce.
“As an AI community we’ve exhausted all of the easy data, the internet data, and now we need to move on to more complex data,” Wang told the Financial Times. “The quantity matters but the quality is paramount. We’re no longer in a paradigm where more comments off Reddit are going to magically improve the models.”
Related Items:
Self-Driving Cars vs. Coding Copilots
Informatica CEO: Good Data Management Not Optional for AI
The Top Five Data Labeling Firms According to Everest Group