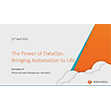
Data At More Than Half Of Companies Will Not Be AI-Ready By The End of 2024
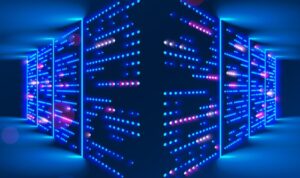
(Andrey Suslov/Shutterstock)
The latest string of evolutions in generative AI has companies everywhere excited about the potential of the technology. Business leaders in every industry are giving in to the FOMO, and racing to check “implement AI” off their list.
However, in this new gold rush of tech possibilities, organizations are also beginning to stumble over unforeseen challenges related to their data. In my experience working with companies across industries, many still have data-related hills to climb with governance, cleanliness and labeling. These are causing hang ups in even some of the largest organizations in the world.
The majority of companies have ongoing data challenges that prevent them from being AI-ready, and I believe more than half of all companies will still be facing those challenges 12 months from now. Companies that want to take full advantage of generative AI’s potential will need to drastically improve their data hygiene practices.
Organizations can move toward a future of generative AI by focusing on improving their data. Here are three foundational areas where tech leaders can take big strides toward making sure their businesses are ready:
Take Time to Clean Your Data
The first step in preparing your data for AI is cleaning it. Even the best AI-driven programs are only as good as the data they are fed, and spreadsheets full of duplicates, errors and missing information will compromise every result.
The cleaning process can be easy to ignore or deprioritize, because it takes a lot of time and there are always projects that seem more pressing or important. However, the payoff is significant where AI is concerned: clean data leads to better results, deeper insights and savings in both time and effort.
Some companies have begun using AI to clean their data, although there are still challenges that limit its success. Aside from still relying on humans to inform the process and ensure the corrections are done properly, AI can’t recreate incomplete or insufficient data. Records are still left with gaps that need to be filled.
Ultimately, cleaning data can feel like a long, unrewarding process, but AI projects are likely to fail without this vital step. In the long run, taking the time upfront is necessary to ensure a company can take advantage of next-generation tools.
Help AI Find Relevant Data with Better Labeling
The idea of a computer program scanning a warehouse of data and plucking out the gems it needs to create insightful results is compelling. It’s also impossible without some sort of road signs and structure guiding it.
AI can do a lot of things, but it is ultimately still just a program reliant on the information that feeds it. Data labeling assigns context to information, so machine learning models can easily find it and use it.
Labeling data can involve a wide range of processes, including annotating, tagging, classifying or transcribing the information. Unless a company takes the time to properly label and annotate its data, even the best generative AI will struggle to provide anything useful.
Like cleaning, labeling data can be a tedious and difficult task; but, it’s also one of the most critical components of creating useful, enriched AI results. Ultimately, a company that wants to take advantage of generative AI must also create the proper labels that will guide algorithms through vast quantities of high-quality data.
Improve Your Data Governance
Good data governance has become an increasingly important practice in the era of big data and digital transformation. As more companies begin to embrace AI, the value and necessity of data governance will continue to skyrocket.
Strengthening your data governance starts with creating or improving a program, building standards and empowering data experts to enforce best practices. Without this crucial structure, the accuracy and viability of AI results will suffer, and your projects will fail.
A strong governance program also helps manage and keep track of all other data components. Once an organization determines its data standards and best practices, and builds strong enforcement structures, the team will have the perfect framework to integrate new information, resolve hygiene challenges and lock down data security.
The increased need for better governance also highlights the evolving role of data analysts, many of whom are anxious about AI’s potential for eliminating their jobs. But, when considering the needs of the data infrastructure — including the governance, but also readiness and shareability — it becomes clear there is still a great need for human experts to oversee accurate and meaningful use of a company’s data.
Organizations can’t afford to be left behind when a new, world-changing technology enters the marketplace. Unfortunately, for many companies, embracing the transformative opportunity of AI is significantly complicated by their ongoing data challenges.
If businesses want to benefit from the potential of generative AI and other projects driven by machine learning, then they need to overhaul their data hygiene. Thoroughly cleaning the data they use, ensuring all the information is properly labeled and overhauling their governance will help companies move to the front of the pack.
About the author: Ben Schein is the Domo, where he heads product design and strategy teams, including product management, UX design, product led growth and strategic architecture. He’s responsible for overall product roadmap and sharing product vision with analysts, customers and other key stakeholders.
Related Items:
Mythbust Your Way to Modern Data Management
Is Your Data Management Strategy Ready for AI? 5 Ways to Tell