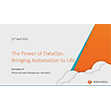
Landing AI Seeks Speed and Simplicity for Computer Vision Apps
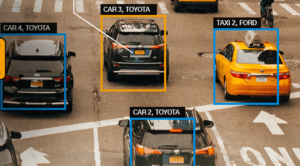
Image source: Landing AI
It may surprise you to learn that, despite the massive hype around large language models, investments in computer vision AI continue to be made. One of the companies accelerating development in this field is Andrew Ng’s Landing AI, which recently rolled out a new program to simplify and speed-up the process of incorporating computer vision models into real-world applications.
Ng is not the biggest fan of big data. In fact, the renowned AI pioneer, who is also a Datanami 2022 Person to Watch, is a vocal proponent of the value of small data. While training on massive image sets has its place, Ng has found that very good results can be had by combining a small set of very well-labeled data and an appropriately designed computer vision model.
Ng’s culture of doing more with less has trickled down through the company and out to customers, says Whit Blodgett, Landing AI’s director of product management.
“Andrews’s machine learning disciples who work at this company have made all the best decisions relative to the industry we’re working with to make these models perfect [in terms of] energy and time efficiency,” he tells Datanami. “You’ll notice our models train in five minutes whereas most computer vision models train in over an hour.”
Landing AI has already built extensive data labeling routines into LandingLens, which different capabilities unlocked the more a customer pays. In June, it launched a software development kit (SDK) to enable developers to incorporate their models into other applications. The SDK currently supports Python and JavaScript, with support for C# planned.
This week the company took another step forward in its journey of democratizing access to AI with the launch of Landing AI Apps Space, which is a repository of applications and use cases designed to help customers explore different ways they might incorporate their LandingLens models into real-world applications.
“It’s essentially an exhibition of different apps you can build around our models,” Blodgett says. “What Landing AI has done is make it very easy to train and deploy models. But there’s the catch that if nobody is using your models, if nobody is running inference with your models, what was that whole effort for? Why do we spending all this time labeling and training if we can’t have it do something useful?”
The Apps Space is tailor made for existing Landing AI customers that have started to train their Landing Lens models, but are having trouble getting that model into a real-world application so that can have an impact. With the App Space, organizations that have already trained a computer vision model and used the new SDK to build a system around it can now extend those models out to their colleagues or communities to build a final application.
“It all comes down to creating a system that does something of value,” Blodgett says. “The training of AI–there’s tons of companies doing that today. But the number of companies making AI available to anybody to utilize in your day-to-day life, bar OpenAI, is quite small. So that’s really what this Landing AI Apps Space is for: It’s meant to give examples of AI systems so that software engineers feel confident going and building their own.”
Landing AI currently offers four types of computer vision models in Landing Lens: object detection, segmentation, classification, and OCR (optical character recognition). Customers would train their models using those models, and then use the SDK to build an application around it. With the Apps Space, data scientists and developers have a way to push the AI model out to their non-data scientist and non-developer colleagues to help them figure out how to incorporate it into what they do.
Blodgett demonstrated how a user could incorporate the OCR capability to build a license plate detection application. Say a company was trying to sell a license plate detection capability to a municipality as part of an Amber Alert program. They could expose the license plate detection AI model to their customers or prospects through a simple QR code read from a mobile phone, and start to play around with the license plate detection AI model right from their phone, Blodgett says.
Another example is around Landing AI’s counting function. The company has developed computer vision models that can count objects in an image very quickly. Whether the object is a dark spot that may represent cancer in an MRI image, or a shadow that may represent a caribou in an aerial drone shot, the AI is able to accurately discern and count thousands of objects very quickly.
Apps Spaces is meant to connect the dots between the extensive data labeling and model training work that data scientists do in LandingLens and the actual deployment of the model into production.
“It’s really meant to have people see how accessible and easy it is to build things and then go and build it themselves,” Blodgett says. “Ninety-nine percent of models that are trained never see production. In our eyes, in Andrew’s eyes, in order for AI to make a positive impact, in order to democratize the use of AI, we need to make that use part the easiest thing.”
Anyone can come and play with these apps, he says. If a customer wanted to actually deploy it, then that is where they will have the discussion with Landing AI around pricing. The company charges based largely on the number of images it trains from, “which is ideal because your’e not training on 10,000 images. You’re training on 10 images or 100 images,” Blodgett says.
Related Items:
How Data-Centric AI Bolsters Deep Learning for the Small-Data Masses
Andrew Ng’s Landing AI Offers Free Trial of LandingLens CV Platform