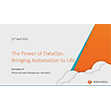
Six Common Signs It’s Time to Invest in Data Reliability
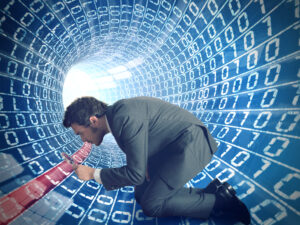
(alphaspirit.it_/Shutterstock)
Every enterprise relies heavily on data to make decisions. This makes data reliability crucial. Without it, you may not find the path to streamlined customer experience and revenue generation. However, data reliability is a fairly new concept in data operations. At its core, it’s about treating data quality like an engineering problem. That means building reliable systems and processes and implementing practices and tools like SLAs, instrumenting dashboards, monitoring, tracking, alerting, and incident management.
But, how do you know if your organization needs to invest in data reliability, and how should they go about it? Like many things in life, data reliability is easier said than done. Many factors can impede it implementation: from complex and dynamic data pipelines, to lack of visibility and governance, to human errors and biases, to insufficient tools and processes.
Here are six common signs that indicate it’s time to take action:
1. No Confidence in Your Analytics/Dashboards
Lack of trust in your analytics and dashboards is a telling sign that you need data reliability. When executives doubt the reports, doubt is cast throughout the entire organization. Whether it’s because they’ve been burned before, or because the numbers aren’t saying what they expected, trust in data is easily lost. But with data reliability measures in place, faith in the numbers can be restored, and teams can confidently move forward with data-driven decisions and directives.
2. Engineers and Data Scientists Ignoring Data Alerts
Like crying wolf, if your engineers and data scientists receive too many alerts about potential data issues, they can become complacent. Too many false positives or trivial alerts can lead to alert fatigue. Your data alerts should be meaningful and timely, so that you can quickly detect and resolve any errors. If not, your organization may overlook a real problem.
3. Failing Data Quality Initiatives
You’ve launched data quality initiatives with the best intentions, but they keep failing, costing more than you thought, or getting blocked. Why? Common reasons include a lack of clarity and a lack of alignment between different stakeholders. If your data quality initiatives are unclear and/or ineffective, data reliability can tie the investment to measurable metrics like NPS scores, and to business outcomes.
4. Data Pipelines Run on Fridays So Engineers Can Debug on the Weekends
Organizations have been known to schedule data pipeline runs on Fridays, so that errors may be debugged over the weekend. Like having someone babysit the data pipeline, this is a coping mechanism for the lack of data reliability. In an ideal world, your data should be ready for consumption at any time, so that you can deliver fresh and accurate data to stakeholders on demand. If you can’t, you’re compromising data quality and timeliness, and putting unnecessary pressure on your engineers.
5. The Number of Duplicate Tables Keeps Going Up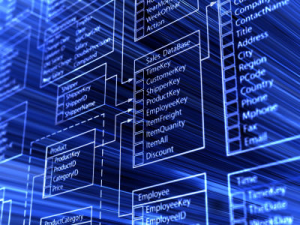
If you have a huge number of duplicate tables, it’s generally because people don’t know where to find data, so they reinvent wheel, over and over again. What follows are inconsistencies and inaccuracies across key metrics, rippling throughout the organization. Invest in data reliability to establish a single source of truth for your data, reducing confusion and errors.
6. You Are Planning an IPO
While extensive resources are available to support finance teams going public, not a lot exists to help data teams faced with the same challenge. Once you determine who has access to what data, you will need to partner with your Privacy, Security, and legal teams to determine how to govern data access moving forward. Once your company goes public, you’ll be required to file accurate and auditable data reports on a regular basis to meet various regulatory standards. If your data is unreliable or inconsistent, you face legal risks and reputational damages from potential errors or misstatements in your filings.
Data reliability is the end-goal state for any organization relying on data (spoiler alert: that’s probably every organization). Through data reliability, organizations build trust across their entire ecosystem of internal and external stakeholders. Bottom line: If teams attempt to use data but it’s wrong or confusing, they’ll be hesitant to rely on it the next time around. If data is trustworthy, teams will use it.
About the author: Kyle Kirwan is the co-founder and CEO of Bigeye, a provider of data observability tools. In his career, Kirwan was one of the first analysts at Uber. There, he launched the company’s data catalog, Databook, as well as other tooling used by thousands of their internal data users. He then went on to co-found Bigeye, a Sequoia-backed startup that works on data observability. You can reach Kyle on Twitter at @kylejameskirwan or on LinkedIn.
Related Items:
There Are Four Types of Data Observability. Which One is Right for You?
Observability Primed for a Breakout 2023: Prediction
Why Roblox Picked VictoriaMetrics for Observability Data Overhaul