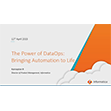
Novel Techniques Extract More Accurate Data from Images Degraded by Environmental Conditions
July 28, 2021 — Computer vision technology is increasingly used in areas such as automatic surveillance systems, self-driving cars, facial recognition, healthcare and social distancing tools. Users require accurate and reliable visual information to fully harness the benefits of video analytics applications but the quality of the video data is often affected by environmental factors such as rain, night-time conditions or crowds (where there are multiple images of people overlapping with each other in a scene). Using computer vision and deep learning, a team of researchers led by Yale-NUS College Associate Professor of Science (Computer Science) Robby Tan, who is also from the National University of Singapore’s (NUS) Faculty of Engineering, has developed novel approaches that resolve the problem of low-level vision in videos caused by rain and night-time conditions, as well as improve the accuracy of 3D human pose estimation in videos.
The research was presented at the 2021 Conference on Computer Vision and Pattern Recognition (CVPR), a top ranked computer science conference.
Combating visibility issues during rain and night-time conditions
Night-time images are affected by low light and man-made light effects such as glare, glow, and floodlights, while rain images are affected by rain streaks or rain accumulation (or rain veiling effect).
“Many computer vision systems like automatic surveillance and self-driving cars, rely on clear visibility of the input videos to work well. For instance, self-driving cars cannot work robustly in heavy rain and CCTV automatic surveillance systems often fail at night, particularly if the scenes are dark or there is significant glare or floodlights,” explained Assoc Prof Tan.
In two separate studies, Assoc Prof Tan and his team introduced deep learning algorithms to enhance the quality of night-time videos and rain videos, respectively. In the first study, they boosted the brightness yet simultaneously suppressed noise and light effects (glare, glow and floodlights) to yield clear night-time images. [See Figure 1a below] This technique is new and addresses the challenge of clarity in night-time images and videos when the presence of glare cannot be ignored. In comparison, the existing state-of-the-art methods fail to handle glare. [See Figure 1b below]
Night-time images
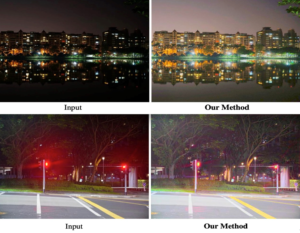
Figure 1a: Unlike existing methods, the new method suppresses the light effects and generates better visibility enhancement results.
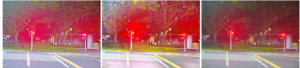
Figure 1b: Existing visibility enhancement and imaging methods as shown above cannot handle the light effects (e.g. glare) and incorrectly intensify them.
3D Human Pose Estimation: Tackling inaccuracy caused by overlapping, multiple humans in videos
At the CVPR conference, Assoc Prof Tan also presented his team’s research on 3D human pose estimation, which can be used in areas such as video surveillance, video gaming, and sports broadcasting.
In recent years, 3D multi-person pose estimation from a monocular video (video taken from a single camera) is increasingly becoming an area of focus for researchers and developers. Instead of using multiple cameras to take videos from different locations, monocular videos offer more flexibility as these can be taken using a single, ordinary camera – even a mobile phone camera.
However, accuracy in human detection is affected by high activity, i.e. multiple individuals within the same scene, especially when individuals are interacting closely or when they appear to be overlapping with each other in the monocular video.
In this study, the researchers estimate 3D human poses from a video by combining two existing methods, namely, a top-down approach or a bottom-up approach. By combining the two approaches, the new method can produce more reliable pose estimation in multi-person settings and handle distance between individuals (or scale variations) more robustly. Figure 2 shows the comparison results between the new method and the existing state-of-the-art top-down and bottom-up methods.
Figure 2: Inaccurate 3D multi-person pose estimation from existing top-down (2nd row) and bottom-up (3rd row) methods. The new method proposed by the Yale-NUS team substantially outperforms the state-of- the-art methods.
The researchers involved in the three studies include members of Assoc Prof Tan’s team at the NUS Department of Electrical and Computer Engineering where he holds a joint appointment, and his collaborators from City University of Hong Kong, ETH Zurich and Tencent Game AI Research Center. His laboratory focuses on research in computer vision and deep learning, particularly in the domains of low level vision, human pose and motion analysis, and applications of deep learning in healthcare.
“As a next step in our 3D human pose estimation research, which is supported by the National Research Foundation, we will be looking at how to protect the privacy information of the videos. For the visibility enhancement methods, we strive to contribute to advancements in the field of computer vision, as they are critical to many applications that can affect our daily lives, such as enabling self-driving cars to work better in adverse weather conditions,” said Assoc Prof Tan.
Click here to read the full article.
Source: Yale University