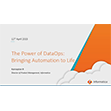
Data Governance: Simple and Practical
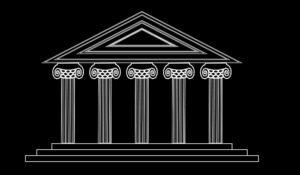
(sondem/Shuttertock)
In 2022, the global data governance market grew to $3.2 billion, a figure that’s dwarfed by the significant cost to operate data governance programs. In spite of the spend, many programs fail to generate high business value because they lack practicality and simplicity: the art of maximizing the amount of work not done.
Where data governance programs often get off track is right before they get on, by defining the charter and roadmap too broadly. The key question is which elements of a data governance program should you chase out of the gate: ownership and stewardship? Committees and councils? Policies and standards? Data quality? Metadata or master data management? Data security and access? Issue management? Technology?
Choose the single element fundamental to fulfill the primary purpose of the program. To do this, answer two questions: what outcomes does the business need and what are the most urgent? From there, codify the primary purpose in your data governance charter and establish its pursuit as the first leg of your roadmap.
Focus on the Purpose and Postpone the Rest
Purpose-driven data governance programs narrow their focus to deliver urgent business needs and defer much of the rest, with a couple caveats. First, data governance programs are doomed to fail without senior executive buy-in and continuous engagement of key stakeholders. Without them, no purpose can be fulfilled. Second, data governance programs must identify and gain commitment from relevant (but perhaps not all) data owners and stewards, but that doesn’t necessarily mean roles and responsibilities need to be fully fleshed out right away.
Identify the primary purpose then focus on it – sounds like a simple formula, but it’s not obvious. Many data governance leaders are quick to define and pursue their three practices or five pillars or seven elements, and why shouldn’t they? They need those capabilities, but wanting it all comes at the sacrifice of getting it now. Generate business value with your primary purpose before expanding.
I’ve consulted with multiple customers whose different symptoms trace back to the same blight: a tech company that couldn’t get the trust in trusted data, an insurer that struggled to improve data quality, and a multinational financial services firm that couldn’t remain compliant.
Each approached data governance with a big bang approach instead of simplistically and practically.
Trusted Data
A tech company described their struggles to me this way: in quarterly business reviews cross-functional leaders couldn’t agree on the numbers, what metrics meant, where to source them, or how to interpret them. The primary purpose of their data governance program needed to be: to improve trust in the data. Their pathway looked like:
- Identify the critical data elements in dispute
- Negotiate and agree to data definitions; codify them in business glossaries
- Make those data elements available in source of truth datasets (if you show up to QBR with a different number, you’re wrong)
- Repipe widely used dashboards to the source of truth
Simplicity. The data governance elements missing from this pathway were everything that didn’t swiftly move the needle on trusted data. What use are policies without trust? Why improve the quality of data that no one understands?
Data Quality
An insurer explained to me their dashboards weren’t always refreshed, and when they were, wide fluctuations in values made it impossible to make informed decisions. Their primary purpose needed to be: to improve the quality of the data. Their pathway looked like:
- Identify unreliable dashboards and critical data elements
- Uncover the most adversely impacting data quality dimensions, for instance:
- Data Timeliness – Frequently failing pipelines without service-level agreements (SLAs) mean users can’t gauge data staleness
- Data Accuracy – Improper tooling to exchange data over a hybrid multi-cloud environment leads to data interpretation inconsistency
- Benchmark data quality measurements; put profiling and dashboarding in place
- Begin the search for the right hybrid multi-cloud technology vendor
- Improve data quality: define SLAs, reschedule pipelines, standardize data assets
Simplicity. Missing from the pathway were committees and councils, which might improve quality, but not as directly or quickly as attacking the root cause head on.
Regulatory Compliance
For a financial services firm, new regulations were inconsistently interpreted and wastefully implemented across the globe. Their primary purpose needed to be: to become truly compliant. Their pathway looked like:
- Establish data governance committees, councils, and working groups with clear responsibilities and accountabilities
- Document policies and standards around compliance definitions and implementation methods
- Implement and enforce
Remember, keep it simple, purposeful, and laser focused, and evolve as business needs change. That way you build your pillars while delivering business value continually.
About the author: Shayde Christian is the Chief Data & Analytics Officer for Cloudera, where he guides data-driven cultural change to generate maximum value from data. He enables Cloudera customers to get the absolute best from their Cloudera products such that they can generate high value use cases for competitive advantage. Previously a principal consultant, Shayde formulated data strategy for Fortune 500 clients and designed, constructed, or turned around failing enterprise information management organizations. Shayde enjoys laughter and is often the cause of it.
Related Items:
The Rise and Fall of Data Governance (Again)
Governance, Privacy, and Ethics at the Forefront of Data in 2021
Finding the Data Access Governance Sweet Spot
July 3, 2025
- FutureHouse Launches AI Platform to Accelerate Scientific Discovery
- KIOXIA AiSAQ Software Advances AI RAG with New Version of Vector Search Library
- NIH Highlights AI and Advanced Computing in New Data Science Strategic Plan
- UChicago Data Science Alum Transforms Baseball Passion into Career with Seattle Mariners
July 2, 2025
- Bright Data Launches AI Suite to Power Real-Time Web Access for Autonomous Agents
- Gartner Finds 45% of Organizations with High AI Maturity Sustain AI Projects for at Least 3 Years
- UF Highlights Role of Academic Data in Overcoming AI’s Looming Data Shortage
July 1, 2025
- Nexdata Presents Real-World Scalable AI Training Data Solutions at CVPR 2025
- IBM and DBmaestro Expand Partnership to Deliver Enterprise-Grade Database DevOps and Observability
- John Snow Labs Debuts Martlet.ai to Advance Compliance and Efficiency in HCC Coding
- HighByte Releases Industrial MCP Server for Agentic AI
- Qlik Releases Trust Score for AI in Qlik Talend Cloud
- Dresner Advisory Publishes 2025 Wisdom of Crowds Enterprise Performance Management Market Study
- Precisely Accelerates Location-Aware AI with Model Context Protocol
- MongoDB Announces Commitment to Achieve FedRAMP High and Impact Level 5 Authorizations
June 30, 2025
- Campfire Raises $35 Million Series A Led by Accel to Build the Next-Generation AI-Driven ERP
- Intel Xeon 6 Slashes Power Consumption for Nokia Core Network Customers
- Equal Opportunity Ventures Leads Investment in Manta AI to Redefine the Future of Data Science
- Tracer Protect for ChatGPT to Combat Rising Enterprise Brand Threats from AI Chatbots
June 27, 2025
- Inside the Chargeback System That Made Harvard’s Storage Sustainable
- What Are Reasoning Models and Why You Should Care
- Databricks Takes Top Spot in Gartner DSML Platform Report
- Why Snowflake Bought Crunchy Data
- LinkedIn Introduces Northguard, Its Replacement for Kafka
- Change to Apache Iceberg Could Streamline Queries, Open Data
- Snowflake Widens Analytics and AI Reach at Summit 25
- Fine-Tuning LLM Performance: How Knowledge Graphs Can Help Avoid Missteps
- Agentic AI Orchestration Layer Should be Independent, Dataiku CEO Says
- Top-Down or Bottom-Up Data Model Design: Which is Best?
- More Features…
- Mathematica Helps Crack Zodiac Killer’s Code
- ‘The Relational Model Always Wins,’ RelationalAI CEO Says
- Confluent Says ‘Au Revoir’ to Zookeeper with Launch of Confluent Platform 8.0
- Solidigm Celebrates World’s Largest SSD with ‘122 Day’
- AI Agents To Drive Scientific Discovery Within a Year, Altman Predicts
- DuckLake Makes a Splash in the Lakehouse Stack – But Can It Break Through?
- The Top Five Data Labeling Firms According to Everest Group
- Supabase’s $200M Raise Signals Big Ambitions
- Toloka Expands Data Labeling Service
- With $17M in Funding, DataBahn Pushes AI Agents to Reinvent the Enterprise Data Pipeline
- More News In Brief…
- Astronomer Unveils New Capabilities in Astro to Streamline Enterprise Data Orchestration
- Databricks Unveils Databricks One: A New Way to Bring AI to Every Corner of the Business
- BigID Reports Majority of Enterprises Lack AI Risk Visibility in 2025
- Seagate Unveils IronWolf Pro 24TB Hard Drive for SMBs and Enterprises
- Astronomer Introduces Astro Observe to Provide Unified Full-Stack Data Orchestration and Observability
- Snowflake Openflow Unlocks Full Data Interoperability, Accelerating Data Movement for AI Innovation
- BigBear.ai And Palantir Announce Strategic Partnership
- Gartner Predicts 40% of Generative AI Solutions Will Be Multimodal By 2027
- Databricks Donates Declarative Pipelines to Apache Spark Open Source Project
- Code.org, in Partnership with Amazon, Launches New AI Curriculum for Grades 8-12
- More This Just In…