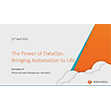
Data Classification: A Critical Piece to Your Data Security Puzzle
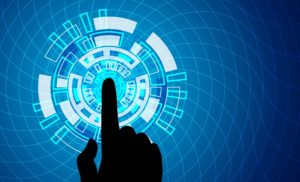
(IceHawk33/Shutterstock)
With constant regulatory scrutiny and data breaches on the rise, companies of all industries and sizes are under pressure to get their data management and security strategies in tip-top shape. Yet, an important element of any data strategy–data classification–is often pushed to the bottom of the list. Indeed, many data teams avoid data classification completely because of how complex it can be to identify and classify sensitive data accurately.
With a better understanding of the process, companies can move past common roadblocks and create an effective data classification policy.
What is Data Classification and Why Is It Important?
Data classification is the process of organizing data into categories, either manually or through the use of technology, with the purpose of making it easier to store, manage, and secure. When done properly, data classification helps with data discovery and management, information security and risk management, compliance, and more.
For example, General Data Protection Regulation (GDPR) has made data classification more important than ever for companies that do business with the EU. Companies that correctly classify data can more easily comply with GDPR (and other regulations) and, in the event of an audit, prove compliance by logging, tracking, and reporting sensitive data usage.
Data classification is also a critical part of data security. Statistics show that nearly 62% of U.S. firms suffered a data breach last year and over 80% contained a human element, including incidents where employees compromised confidential records. These breaches can lead to regulatory fines, legal repercussions, and reputational damage.
Classifying data helps business leaders become more aware of the types of sensitive data being stored and who is accessing it. Appropriate access controls can be set as a result of the data classification process to maintain confidentiality, make data more easily accessible to authorized users, and prevent data loss and corruption.
Who Needs to Consider Data Classification?
According to Forrester, data classification is a foundational capability that companies should develop to optimize security, privacy, and compliance efforts (Forrester, Now Tech: Data Discovery And Classification, Q4 2020).
Really, any company that maintains sensitive data on customers or partners should be weighing data classification projects, but let’s look at the healthcare industry as a specific example:
Healthcare companies store massive amounts of sensitive patient information, including demographic details, medical records, and billing data. They are also required to comply with data privacy regulations, such as HIPAA. A data classification policy can quickly prove that a healthcare company is compliant by keeping all personal health information (PHI) secure, providing a record of how the company is protecting the data, and tracking who accesses the data, when, and why.
Why Are Data Classification Projects So Challenging?
For starters, most data classification projects are not usually well-defined or planned, and companies often don’t have the resources to classify data manually or the budget to implement comprehensive data classification technologies. Other struggles include:
- Locating sensitive data: It’s incredibly hard to locate every single piece of sensitive data that a company collects and stores. Companies utilize many platforms like on-premise or cloud databases, lakes, or warehouses to store large datasets, and a lot of that data is semi- or unstructured. Together, this creates a huge classification challenge.
- Defining the right categories: The actual act of classifying data once it is found can also be complex. Most data can be classified by low-, moderate-, or high-risk (some companies also use severe), but various governing bodies recommend different categories. For example, the Center for Internet Security (CIS) recommends three information classes (Public, Business Confidential, and Sensitive) whereas the U.S. government has a more extensive classification, with seven levels ranging from Controlled Unclassified Information (CUI) to Top Secret and Restricted. And often, the process is prone to human error, as the users classifying the information may make mistakes.
- Dealing with continuously changing data: Data is often copied, modified, moved, or deleted. Keeping classification accurate and up-to-date when data is continuously changing is not easy, nor is it realistic to create a different policy for each new dataset. Without automated and continuous data classification in place, the process can become extremely time-consuming.
- Classification errors (false negatives and false positives): If data is wrongly classified as high-risk, unnecessary security measures may be triggered (this is a false positive). And if highly sensitive data is mistakenly classified as low-risk (a false negative), companies are leaving data vulnerable and may risk data loss and compliance violations.
Four Tips for a Scalable Data Classification Policy
A data classification policy is meant to standardize how sensitive information is handled throughout its lifecycle and to define exactly how a company stores, retrieves, and manages it.
Gartner recommends a data classification policy that is short and easy to understand, has no more than three or four classification levels, is flexible and allows for controlled exceptions, and avoids references to technology, departments, or data types that may age (Gartner, Building Effective Data Classification and Handling Documents, September 2022).
Here are a few other best practices organizations should consider when developing a data classification policy:
- Conduct a data risk assessment: A data risk assessment can help define company policies, regulatory demands, contractual requirements, confidentiality needs, data classification objectives, and key stakeholders with whom to coordinate.
- Inventory all of the data: Before data can be classified, it must be located, identified, and grouped. There are tools that can automate this process using predetermined criteria to continuously identify, classify, and tag data with the appropriate risk category (i.e., low, moderate, or high).
- Implement data security and access controls: Define security and access controls for each classification level. For example, maybe low-risk data is open to all within the organization whereas high-risk data is managed using role-based access control (RBAC) or a just-in-time (JIT) access approach. The data’s storage location and its value to the organization will influence its risk factor and level of control needed.
- Ensure monitoring is continuous and flexible: Because data is dynamic, it needs to be continuously monitored and classification policies need to be flexible enough to handle new data types, changes in data structure, or increasing volumes of data. This can be done via an automated or a manual process, though automation can cut down on workflow bottlenecks and improve efficiency.
Data classification allows an organization to create one, single policy for handling sensitive data across the entire organization and throughout the data lifecycle. An effective data classification policy will protect sensitive customer and business data, support compliance, and enable more secure data sharing to power decision-making.
Just like a company would adjust its cyber security policies as new threats emerge, so too should it evolve its data classification policy. This becomes even more important as organizations adopt new data storage approaches or new regulations emerge. A flexible data classification policy built on the above tenants will scale with your data-driven organization.
About the author: Ben Herzberg is the chief scientist at Satori, a provider of secure data access solutions. Satori’s Secure Data Access Platform integrates into any environment to automate access controls and deliver data-flow visibility via activity-based discovery and classification. Herzberg is an experienced leader in research and development, with experience as a CTO, VP R&D, developer, hacker, and technical manager.
Related Items:
How Providence Overcame Security Obstacles to Unlock Medical Data in the Cloud
8 Best Practices for Approaching Master Data Governance in the Cloud
Five Emerging Trends in Enterprise Data Management