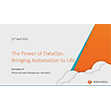
Nvidia’s Hopper GPUs Enter ‘Full Production,’ DGXs Delayed Until Q1
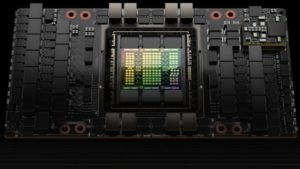
Just about six months ago, Nvidia’s spring GTC event saw the announcement of its hotly anticipated Hopper GPU architecture. Now, the GPU giant is announcing that Hopper-generation GPUs (which promise greater energy efficiency, greater power and lower TCO) are in “full production” and disclosed additional details regarding availability — on-prem and in-cloud — of the new hardware.
The short version: partner systems featuring the Nvidia H100 GPU (from Atos, Cisco, Dell, Fujitsu, Gigabyte, HPE, Lenovo and Supermicro, among others) are slated to begin shipping in October — a slight slip from Nvidia’s Q3 shipping estimate from that spring GTC announcement. PCIe-based systems will be made available first, followed by NVLink HGX platforms later in the year. (Nvidia attributes this delay not to component availability, but rather to the complexity of the HGX solution relative to PCIe solutions.) Meanwhile, DGX systems featuring the H100 — which were also previously slated for Q3 shipping — have slipped somewhat further and are now available to order for delivery in Q1 2023.
On that front, just a couple months ago, Nvidia quietly announced that its new DGX systems would make use of Intel’s forthcoming Sapphire Rapids CPUs — a shift from the AMD Epyc CPUs that had powered their prior-generation (A100) systems. Those Sapphire Rapids CPUs have been much-delayed from their initial ship date projection (2021) and now appear to be slated for production ramp in Q1 of next year. Other targets for the H100 include AMD’s forthcoming Epyc Genoa CPU (also slated for next year) and Nvidia’s own Arm-based Grace CPU (you guessed it — next year). The H100 may be in “full production,” but its primary CPU counterparts will be racing to catch up.
Still, there will be other ways to utilize the new Hopper GPUs. Nvidia announced that the H100 (housed in Dell PowerEdge servers) is now available on Nvidia LaunchPad, which lets users try out Nvidia’s hardware and software stacks in a short-term, hands-on trial environment. The H100s are also making their way to the cloud, of course, with Nvidia announcing that AWS, Google Cloud, Microsoft Azure and Oracle Cloud Infrastructure will all be “among the first” to deploy H100-based instances sometime next year.
On the research front, major supercomputers set to debut the H100 include Alps (CSCS), MareNostrum 5 (Barcelona Supercomputing Center), Venado (Los Alamos National Laboratory), the tentatively named Cygnus-BD system (University of Tsukuba) and the already-operational Lonestar6 system at the Texas Advanced Computing Center. Some of these systems will incorporate the H100 via Nvidia’s forthcoming Grace Hopper Superchips, which will feature tightly linked Grace CPUs and Hopper GPUs.
The systems deploying the H100 will enjoy drastic improvements over the already-popular A100, which has become the de facto standard of comparison over the last few years amid fierce and growing accelerator competition from established giants and specialist startups. The H100, Nvidia says, delivers 30 teraflops (FP64) of computing power (compare: 9.7 for the A100) and offers 3.5× more energy efficiency and 3× lower TCO relative to the A100. (Note: the PCIe version of the H100 delivers 24 FP64 teraflops rather than 30.)
“Nvidia is on track to make Hopper the most consequential datacenter GPU ever, in part because of the 5× performance boost for large language models, but more because of the ever-broadening array of software for industry and the enterprise,” commented Karl Freund, founder and principal analyst at Cambrian AI Research. “There are lot of companies out there just trying to match Nvidia’s performance; they haven’t even begun to address the deep and broad software stack that turns all those transistors into solutions.”
To Freund’s point, Nvidia also announced today that it has begun optimizing leading large language models and deep learning frameworks on the H100, including Microsoft DeepSpeed, Google JAX, PyTorch, TensorFlow, XLA and Nvidia’s own NeMo Megatron.