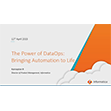
Data Is Everywhere, But Harvest Your Own for Peak AI Performance
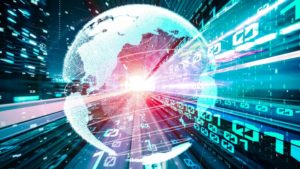
(Blue-Planet-Studio./Shutterstock)
The rapid proliferation of data marketplaces has made it easy for organizations to get their hands on third-party data. And pre-trained deep learning models are also readily available on the Internet. But just as plastic wrapped, ready-to-eat food often isn’t the healthiest choice, pre-packaged data may not have your AI models running at peak performance.
In the early days of big data, organizations focused heavily on data science as the path to machine learning success. Data scientists spent a lot of time and energy training their models from scratch, and then tuning them to achieve the best accuracy. But with the rise of new technologies and techniques, including deep learning and transfer learning, the balance of power is shifting away from data scientists and toward the data itself.
Data reigns supreme in the land of data-centric AI. While data scientists are still a critical piece of the puzzle, their skillsets often are not as essential to success as they once were. Instead, having the data set that most closely represents the real-world conditions that one’s AI is likely to encounter may be a better way to go.
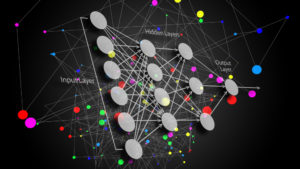
You can fine tune a pre-trained deep neural network by utilizing transfer learning with your own data (Pdusit/Shutterstock)
The wide availability of pre-trained deep learning models and transfer learning techniques is revolutionizing enterprise AI by allowing organizations to get new AI use cases up and running very quickly, says Wilson Pang, the CTO of Appen, a provider of data labeling tools and services.
“A lot of times, you have a model which is already pretrained using some open source dataset or another existing data set from your own company, and then you take that model to the new use case,” Pang says.
With transfer learning, the practitioner may re-use 16 layers out of a 20-layer deep learning model alone, for example, and instead focus on retraining just four layers, Pang says. That allows the user to leverage the training that has already occurred and which exists in the open realm, while fine-tuning the model to work better on specific data that the model has never seen before.
Data-Centric AI
Pang uses a hypothetical example of an AI model for the travel industry. There are lots of images of hotels in ImageNet, the open source repository of more than 14 million images used to train computer vision algorithms. But it likely doesn’t have the right ones.
“I need to understand if the image is about customers,” Pang says. “Is this about a hotel room? This is a lobby in the hotel, this is the restaurant, etc. I need to classify those, but I don’t have tens of millions of images to train those models.”
With transfer learning, Pang can start with an image classification model pre-trained on ImageNet. But instead of using the model as is, Pang can supply the specific images that he needs for his hypothetical travel industry AI model–perhaps numbering in the several thousand–and use those to finish training his model.
“You’re using your own data to really retrain the model, to just tune the parameters for those last few layers,” he tells Datanami. “You get a model that works well for that data set.”
Each use case is different, and there are no absolutes. But transfer learning has wide applicability in the most popular AI use cases, including those involving computer vision and natural language processing.
In NLP, large language models like GPT-3 are trained on vast corpus of text, and require millions of dollars’ worth of compute to fully train. It wouldn’t be practical for most organizations to train their own large language model from scratch. But armed with a pre-trained model and a small collection of custom data, transfer learning can help a big data practitioner swing above her weight.
Focus on the Data
Organizations can save money and get higher performing AI models by focusing on having high quality data from the beginning, Pang says.
“We see use cases where some customers…get all this training data at a lower price, then later on they find that the quality is not as good, and basically they need to redo the training,” he says. “All that money got wasted.”
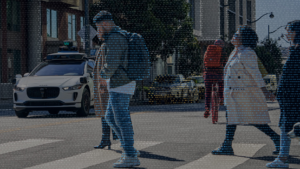
Waymo’s Open Data set is a good starting point for a self-driving car model — but a competitor would need its own data (Image courtesy Waymo)
It’s rare to find open source repositories of high-quality data that are useful for training specific types of AI. That’s why, in almost all cases, it will be up to the individual organization to source that data themselves. “That’s not very common” to buy high-quality data for last-mile AI training. “Normally you have to collect your own data,” he says.
For example, Waymo has open sourced its repository of data collected from self-driving car experiments. That could be useful for a competitor, but only up to a point. It’s likely any competitor would have slightly different data-collection techniques and therefore would need different data to finish the self-driving car model.
“Their data might be very different than the data from Waymo, because their camera is different, the LIDAR is different, the car is different,” Pang says. “But still, we’re talking about connected car data, so you can use the data set from Waymo to do some pre-training, and then do some transfer learning” to fine-tune a new model.
There are lots of great open data sets out there, and Pang encourages people to use them. But he emphasizes that users will more than likely need to bring their own data to bear to get the best performance with their particular AI model.
“I think the focus on the data now is much more important than before,” he says. “People are realize that spending the time and effort to get the data right actually can help the model to improve performance significantly.”
Related Items:
How Data-Centric AI Bolsters Deep Learning for the Small-Data Masses
Is Data-First AI the Next Big Thing?