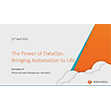
Gretel Keeps the Data Trail Hidden
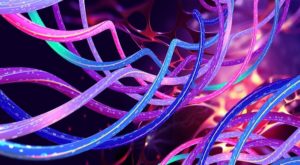
(Yurchanka-Siarhei/Shutterstock)
When Alex Watson co-founded the security company Harvest.ai back in 2014, using machine learning to identify sensitive data to protect it seemed like a good idea–so good, in fact, that AWS bought the company. Fast forward eight years, and Watson’s latest startup, Gretel.ai, is also using machine learning to stop security threats to data, but in an entirely different way.
AWS had been trying to launch a security service for years, and didn’t have much luck, so instead it acquired San Diego-based Harvest.ai in 2017 for a reported $20 million. Watson became the general manager of Amazon Macie, a security service based on Harvest’s AI and machine learning technology that helps customers discover, classify, and protect their sensitive data in AWS.
It was a great experience for Watson, who started his career in the Department of Defense before working at private security software companies. “We went from zero to one of the top 25 revenue-generating services for AWS in the time I was there,” he tells Datanami. “We worked with really big companies that were trying to solve data problems at scale.”
The problem was centered on personally identifiable information (PII). While the data was incredibly useful for analytics, letting people access it carried significant security risks. Building systems that could enable analysts to query this sensitive data while simultaneously abiding by security and compliance requirement proved to be a monumental task for just about any company that wasn’t a tech giant.
Internally, AWS had the luxury of a 500-person compliance team that helped prepare data and steer analysts clear of PII as they accessed sensitive data in the warehouse. That resource provided an incredible advantage for AWS, Watson says, but it wasn’t an advantage shared by customers.
“Even talking to our most sophisticated customers, like those born in the cloud, Airbnb’s of the world–even those incredible companies struggle with how to enable access to data inside the walls,” Watson says. “How do you let your teams query that really sensitive customer data?”
Synthetic Data
That conundrum was the genesis for Gretel.ai, which Watson co-founded with Ali Golshan, John Myers, and Laszlo Block in 2019. If the need to protect PII is causing so much pain, then why not just replace real data that includes PII with synthetic data that does not?
Watson explains:
“What if that idea that you have to wait for anywhere from three weeks to six months to get access to internal data to test an idea–what if that was a false choice?” he says. “What if you could get access to an artificial version of that really sensitive data that didn’t point back to real customers but gave you 95% of the utility? What if you could access that in 5 minutes?”
Gretel isn’t the first company to catch on to the value of synthetic data in the big data age. But its approach may be among the most comprehensive in the market. In addition to NLP technology that identifies PII for customres, the Gretel Toolkit also includes the capability to generate synthetic data, as well as to use privacy-preserving techniques to transform sensitive data so that it’s not so sensitive anymore.
Gretel’s cloud-based synthetic data generation technology uses deep learning technotes to train a model on real customer data. The source data can be anything: text, tabular data, time-series, and it’s currently working on image data.
Once the AI model has been trained, it can be used to generate synthetic versions of the real data that contain none of the sensitive data that makes real data so risky to use. Users can then use the synthetic data for analysis purposes or to train machine learning systems, Watson says.
“We went out there and said, can we help break down data silos and enable data sharing inside businesses?” Watson says. “Rather than building walls around sensitive data, just build another version that it doesn’t matter if it gets breached. It’s not compliance-controlled, because it doesn’t point back to real humans. That’s the goal that we’re building toward.”
Illuminating Synthetic Data
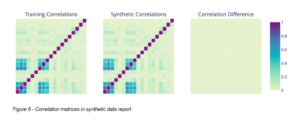
Illumina’s correlation matrices show little difference between real-world data and synthetic data (Image source: Illumina and Gretel)
Gretel is gaining a lot of traction in the medical and financial services industries, which have among the strictest data regulations of all industries. In the financial services area, Gretel often helps companies identify fraud using synthetic data approaches that minimize data risk.
But some of the biggest benefits may come out of the medical field. One company that’s giving Gretel a shot is Illumina, the genetic sequencing company that, like Gretel, is based in San Diego.
According to a case study written by representatives of Illumina and Gretel, Illumina set up a test to see how Gretel’s technique worked with mid-size data set of mice DNA that contained more than 92,000 single nucleotide polymorphisms (SNPs) in 1,200 mice, along with 68 phenotype descriptions.
Illumina conducted two tests that found that the genome-wide association study (GWAS) based on the generated synthetic data closely matched the GWAS done on actual data. It used a Synthetic Quality Score (SQS) that compared the accuracy of the synthetic genotype and phenotype data to the real-world training sets. It also conducted a principal component analysis (PCA) on the results of GWAS, which is a language model, and found the results “look quite promising.”
Some problems cropped up when it used a Manhattan Plot to look more closely at what was going on with specific chromosomes. “The synthetic model was able to capture and replay the strong associations in chromosomes 11 and 5,” the case study states. “However, the synthetic model introduced notable false positive GWAS associations in chromosomes 8, 10, and 12.”
The false positives, the case study says, are most likely due to the small sample size of 1,200 mice. The scientists say they usually recommend at least 10,000 samples for the network to “sufficiently learn to recreate the data, especially with the complexity of the genome containing 92,000 SNPs per mouse.”
The scientists also found the “GWAS association noise floor and y scale is significantly higher in the synthetic data, indicating that the model might be amplifying characteristics in real world data that are being reflected in the GWAS analysis. These differences can also likely be minimized with additional examples and neural network parameter optimization…”
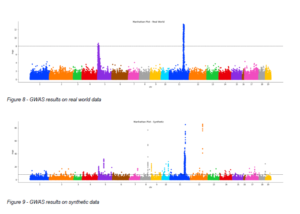
Manhattan Plots showed the limits of a small data set with Illumina’s test of Gretel’s technology (Image source: Illumina and Gretel)
Overall, the test was a success for Illumina. While there were some artifacts with the small sample size, Gretel’s approach “demonstrates encouraging evidence that state of the art synthetic data models can produce artificial versions of even highly dimensional and complex genomic and phenotypic data.”
And at a cost of just $1,440, it was quite a deal, to boot. Illumina says it’s now working to test how the synthetic data approach work with human datasets.
Data Science Enabler
Watson says the Illumina test shows that the precision and characteristics of data can be maintained when generating synthetic versions of real data. This will be especially beneficial in data science use cases where source data is scarce and hard to find, he says.
“No one has enough of the right data. I think that data bottleneck is such a problem right now,” he says. “It’s really expensive to hire annotating services or generate new data or collect more customer data. So a lot of thought-leading ML companies we work with are looking at how do we augment our existing real world customer data with synthetic data to help my machine learning algorithms do better.”
Related Items:
Accenture Report Explores the ‘Unreal’ World of Synthetic Data and Generative AI
Synthetic Data: Sometimes Better Than the Real Thing
Five Reasons Synthetic Data Is the Electrolyte to Speed Up Your AI Initiatives