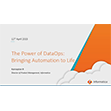
A Bird’s-Eye AI to Coordinate Connected Vehicles
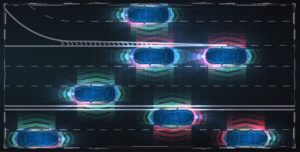
(ZinetroN/Shutterstock)
AI is playing a big role in enabling autonomous vehicles. You likely already know that. But you may not be aware of work that’s occurring to use AI to make roads safer and more efficient for the current mix of users, including manually driven vehicles, bicycles, and even the random pedestrian.
Depending on where you live, self-driving cars are already taking to the roads. We’re currently between level two and level three on the autonomous vehicle scale; fully autonomous driving is usually considered to be level five. But how quickly will level five arrive? Some experts say we’re still decades away from level five.
In the meantime, public officials and technology companies are working to ensure that the transition to autonomous vehicles is done in a safe manner. One of the companies involved in helping that transition along is Derq.
Derq is an MIT spin-off that develops an AI application that can fuse data from multiple sensors, including cameras mounted on vehicles and the side of the road, to monitor and ultimately to help manage the road and improve safety. It works in an area known as vehicle-to-device (V2X) communication.
“We’re trying to create full situational awareness among all the different road users, movements, behaviors, and interactions around the roadway,” says Karl Jeanbart co-founder and COO of Derq. “We like to think of ourselves as a bird’s eye view, a complementary feed of information to what cars are able to see.”
Multi-Sensor Fusion
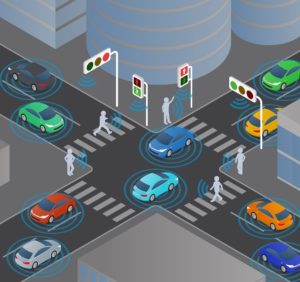
Derq’s AI uses multi-sensor fusion to predict how road users will behave (metamorworks/Shutterstock)
Autonomous vehicles may eventually become ubiquitous, but it seems more likely that AI will become ubiquitous on a mixed road. In other words, yes, we’ll have self-driving Teslas and Cadillacs patrolling the roads. But we’re also going to have your Aunt Millie, who absolutely refuses to give up her 1997 Buick LeSabre. And as her driving skills deteriorate, AI and V2X technology will help to keep her–and the rest of us–safe.
While cars are the primary users of roads, they’re not the only ones. Today’s drivers must share the road with bicyclists, electric scooters, and pedestrians. In some states, pedestrians–even intoxicated ones–have the legal right of way. But even where they don’t, their erratic movements make them a real challenge for drivers (both human and AI) to deal with.
“One challenge today is autonomous driving at scale is not really feasible just because you’re still going to have mixed driving condition with pedestrians and bicycles that are not necessarily connected, as well as non-autonomous, non-connected vehicles,” Jeanbart tells Datanami. “If you’re in an autonomous car and you’re not connected to your ecosystem, you’re not connected to the infrastructure, you’re not going to be able to operate efficiently and smoothly and safely.”
Derq’s initial creation is based on predictive model created by Derq’s co-founder and Georges Aoude at MIT. “It’s an AI model to predict red light-running at intersections with 90% accuracy two seconds sec before the runner actually runs the light,” Jeanbart says. “This gives us two seconds preemptive notice to take an action.”
That action could take one of several forms, including sending an alert to a connected car to watch out for red light runners. Alternatively, Derq’s system could alert the traffic signal to hold the red light for some extra period of time to ensure everybody can safely exit the intersection.
As a key piece of AI infrastructure for connected roads, Derq works with a range of players. It has partnerships with Nvidia and Qualcomm to integrate with their GPU and embedded processors. It also works with municipalities to install its infrastructure into the “roadside furniture” that helps traffic flow, such as the traffic signals. Having standardized communication protocols makes all this possible, Jeanbart says.
“What is very valuable is not just using the senor data, but to also receive data from the vehicles so that your fusion is as complete as possible,” he says. “So if we can receive data from vehicles, receive data from our sensors, receive data from the traffic control equipment, then fuse it all together, we really have that bird’s eye view and have that full situational awareness at an intersection or a roadway.”
Fully Autonomous Left Turns
It’s not difficult to drive a car down a straight road during a sunny day. In fact, even an AI can do it reliably. “We’re not really struggling” with AI in straight, level driving, Jeanbert says. “It will operate well. But when you start entering into those edge cases, when you start interacting with mixed traffic, maneuvering…”
Well, that’s where the fun starts. For example, consider how AI handles a permissive but uncontrolled left turn. Humans can process the various pieces of information required to safely perform the maneuver–looking for gaps in the oncoming traffic, judging speeds and safety windows–but it pushes the bounds of what today’s AI are capable of.
“When you don’t have a dedicated restricted phase for the car to turn left–well this is a nightmare for an autonomous car, because it just cannot handle that type of traffic right now,” Jeanbert says. “And this is where infrastructure is helping this autonomous car work around those edge cases, to look around the corner, do what we call a non-line of sight application.”
Another challenge for self-driving cars: people. While your own movements may be entirely normal and linear, other people do not move in such a predictable fashion. Jeanbert and his team are using AI to predict pedestrian behavior, to understand when and where they’re going to “jump onto the road,” and where “surges” of pedestrian may occur, he says.
“So a group of pedestrians, a cluster is moving around. How are they going to move around? This is another important problem,” he says. “We look at near misses a lot, and conflicts. Why are conflicts happening? Issues around road design, erratic driving. Wrong-way driving is another big one. Lane compliance is another. All those different building blocks make up the overall analytics solution.”
Having a big, diverse set of training data is important to training an algorithm that can protect all the users on the road. Derq’s collection spans not just cars, bicycles, and people, but also this mixture at night and in bad weather, including rain, fog, and snow. All-weather algorithms will be critical to ensuring AI can work reliablyi when the roads get messy.
Fully autonomous vehicles are clearly in our future. But in the meantime, we’ll all benefit from AI-powered roads that help to keep us safe from road hazards, drunk pedestrians, Aunt Millie–and even ourselves.
Related Items:
Don’t Forget the Human Factor in Autonomous Systems and AI Development
Nothing Runs Like a GPU-Powered, Fully Autonomous Deere
Nvidia To Use Virtual Reality for Autonomous Vehicle Testing