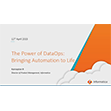
Fake Data Comes to the Forefront
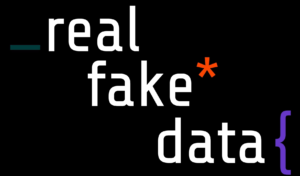
The lack of data historically has been a limiting factor in the development of predictive models. But with the advent of automated methods to generate skads of synthetic data, or what some call “fake data,” the lack of data is no longer the bottleneck that it once was.
One of the latest companies to seize the means of fake data production is Tonic.ai. The San Francisco company this week announced that it has raised $35 million to help it fund the development of its fake-data creation system.
According to Tonic.ai CEO Ian Coe, the company’s software enables customers to “safely and realistically” mimic their existing production data in a way that capture “the full complexity and nuance” of their real data.
“Fake datasets that once took developers days or weeks to build in-house can now be generated in minutes using Tonic,” Coe says in a blog post. “By taking the burden of sourcing data off of developers, we’re quickly becoming an integral part of the modern CI/CD toolchain, allowing developers to move faster, while maintaining compliance and security.”
In some cases, fake data is actually preferred over real data, because it doesn’t carry the same privacy and security risks. This is a conundrum that quality assurance (QA) professionals have been dealing with for years as they look to develop realistic regression tests to ensure that new or modified applications work as they’re designed.
These QA pros have relied upon data masking and obfuscation techniques, but that can take a considerable amount of time to get right. With the “real fake data” generated by Tonic.ai, these folks can get realistic data sets that have all the qualities of real data, but without the possibility of accidentally disclosing somebody’s personal information, which could expose them to fines under GDPR and other data regulations.
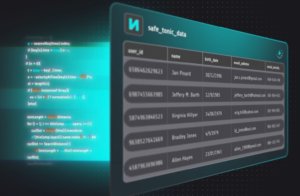
Tonic.ai automatically generates synthetic data that retains the characteristics of real data stored in databases (image Toni.ai)
The company says its generated data “looks, feels and behaves like production data, statistically preserved with differential privacy and really complex mathematics.” While most of the company’s early customers, including eBay, Everlywell, and Kin Insurance, are generating fake data to support QA testing, Tonic.ai also has data scientists in its sights.
“We see a future in which machine learning and AI will be used across the development process, and that process’s interdependency on data operations, data engineering, and data science teams is only going to grow,” Coe says in a press release. “Getting quality, safe data to developers is a gargantuan task in and of itself. But it’s just the start of what we’ve got planned.”
George Mathew, a big data industry veteran who is now the managing director at Insight Partners, the private equity and venture capital outfit that led Tonic’s Series B round, also highlighted the potential use for AI.
“As one of the early players in the synthetic data space, Tonic.ai brought a compelling vision to the industry: to harness the power of synthetic data for all development teams,” Mathew says in a press release. “This is combined with the macro trends towards data privacy, the increasing costs of data breaches, and fast-growing opportunity in artificial intelligence and machine learning workloads.”
Fake data is in a growth stage at the moment, and a number of startups are targeting the space. Another San Francisco startup SynthesisAI is not only generating fake imagery to be used for computer vision models, but also generating the labels that are needed for deep learning systems to train on those images. “Essentially we create 3D hi-fidelity simulations of things that you’re interested in, using techniques from CGI [computer-generated imagery], digital effects, and gaming industry coupled with emerging generative neural networks to create these very immerse, simulated environments,” SynthesisAI CEO and founder Yashar Behzadi told Datanami earlier this year.
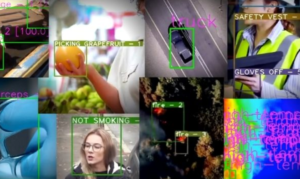
Chooch.ai provides synthetic data for its computer vision training customers (image courtesy Chooch.ai)
Another company generating fake data for computer vision use cases is Chooch.ai. The San Mateo, California company builds a full setup that includes AI training in the cloud and inference on the edge. Its customers in manufacturing, distribution, and defense had a lack of training data, and so earlier this year, it started supplying synthetic data to train the models.
In addition to providing imagery to train neural networks, Chooch.ai provides annotations and other markup, such as bounding boxes around items of interest in the images or video. That that the potneital to save a lot of time for data scientists, says Chooch.ai CEO Emrah Gultekin.
“They love it,” Gultekin told Datanami in an interview earlier this year. “Their lives are currently very miserable. They’re paid a lot of money to draw bounding boxes. It makes no sense.”
A Raleigh, North Carolina-based company called Diveplane is developing a synthetic data platform called Geminai that uses privacy enhancing technology to generate a “verifiable synthetic ‘twin’ dataset with the same statistical properties of the original data,” the company claims. George Leopold wrote about Diveplane’s offering in this October 2019 Datanami article.
According to AIMultiple Product Manager Izgi Arda Ozsubasi, the market for fake data for AI training will grow at a 22.5% compound annual growth rate (CAGR). By 2024, Gartner says that 60% of data used for AI and analytics projects will be synthetically generated, Ozsubasi writes in his report.
Big data used to be a prerequisite for developing the most advanced and accurate models, but that is no longer the case, thanks to the advent of fake data and related privacy-preserving techniques. The market for synthetic data will grow as companies explore the benefits of the synthetic data approach, particularly when paired with pre-trained models.
Related Items:
Synthetic Data: Sometimes Better Than the Real Thing
Five Reasons Synthetic Data Is the Electrolyte to Speed Up Your AI Initiatives
Synthetic Data Market Gets Real