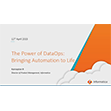
Comparative Analytics Needs A Secure Approach
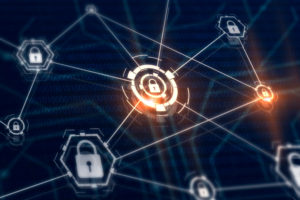
(Michael Traitov/Shutterstock)
Precise comparative data analytics is the proverbial “pot of gold” at the end of the rainbow, but without secure identities, marketers can’t get there.
A realm of critical comparative intelligence is lagging in today’s hyper-analytics world. In today’s modern analytically driven marketing world, practitioners need the capability to compare disparate sets of data easily and represent it easily, yet there are obstacles in the way.
With third-party cookies going away, and privacy laws expanding state by state, brands want to intermingle and analyze the most precise customer data, yet they must battle complexity and ensure privacy with a first-party data strategy. In short, securing data is no longer a nicety, it’s a necessity.
How can brands, publishers, agencies and data providers get to the end of the rainbow? A fundamental approach to data science is the use of comparative analytics. It is used frequently in data comparisons with two or more processes, documents, or data sets to analyze patterns, filter inequities and create decision-tree analytics. Comparative analysis can be used to help assess the different tastes and preferences customers have in high tech devices, foods and beverages, or their choices of clothing.
According to Gartner’s 2020 Data and Analytics Survey, only 54% of marketing decisions are being influenced by marketing analytics. But respondents cite poor data quality, in-actionable results, and nebulous recommendations as top reasons for why they don’t rely on analytics to make decisions. Substantial barriers exist in receiving that information in a simple to understood manner. To make better use of data in business, there needs to be a stronger focus on creating the best representations of data.
One example of the manifestation of having available data is a Venn diagram. The Venn diagram simply shows elements of similarity between concepts, and differences between them as well. And yet, the path to building a Venn diagram is not simple for agencies and marketers who need access to data first. Connecting the underlying identity data in order to compare descriptive or quantitative behaviors, preferences, or purchase history is increasingly difficult but critical step. Line up data first and then aggregate it to uncover a true intersection.
Solving the Problem
How can data experts help brands and publishers address unmet consumer needs for new products or product extensions? The primary way is for data scientists to create secure systems to manage data.
Data signals feed AI and machine learning to drive better segmentation, offers and digital ad targeting at scale. This leads to a deeper knowledge of what consumers desire, and what factors or preferences may be stopping them from engaging.
In order to understand these true intersections companies need a way to bring analytical insights of differing parties together. To date, due to legislation and ethics, few companies, if any, are willing or able to share personal information (PII identifiers) with other companies. In order to address this problem, innovations in providing a secure identity exchange are being developed to break down the barriers, so that analysis can be performed between either two or more customer segments while protecting consumer data.
This means taking a new approach to their data solutions that puts privacy first. The most feasible way is through secure identity exchanges operated independently from brands and marketers. With secure identity exchanges, new possibilities open up, as information can be protected with encryption. Marketers can now use the specific data provided, and overlap the data to gain new insights into new customers and long-term customer behavior. The reason why is that all along the data discovery path, the actual people behind the data now remain anonymous.
Using Comparative Analytics in Data
Tools for performing comparative analytics, with sets of data stemming from differing companies, are much more challenging than self-analytics. With self-analytics, data companies collect data, and then they start to work with it, and they can see the key milestones. In comparative analytics, it’s important to know all the data points, but the data that overlaps may not be exposed.
In comparative analytics it doesn’t matter what a customer looks like to a marketer, it’s all about seeing exactly how customers are different. It’s about understanding a best-selling segment, and what causes that difference.
Companies may have been more liberal in past years in sharing consumer data with partners, or Digital Management Platforms (DMPs). But as the data universe evolves, now it’s time for these companies and brands to evolve their first-party data strategies in a new manner.
Secure Connectivity with a First-Party Data Strategy
As an industry we are at a point where we can fix customer privacy and unsecured data issues by incorporating safe handling of consumer information. A zero-trust model is the logical future of all transactions to make customer data safer and more privacy-compliant. Forward thinking data executives have seen its promise to accelerate commerce and reduce risks.
The good news for marketers is that the security and privacy issues around industry data are being addressed; and with innovations such as secure identity exchanges there will be whole new levels of data depth to understanding customers.
About the author: Brian Mullin is the founder and CEO of Karlsgate, a secure data collaboration company. He has over 30 years of experience in software development with leadership roles at companies across the data-driven marketing ecosystem.
Related Items:
Yes, You Can Do AI Without Sacrificing Privacy
Three Privacy Enhancing Techniques That Can Bolster the COVID-19 Response
Unlocking the Transformative Power of Privacy Enhancing Technologies