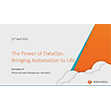
Argonne National Laboratory Applies Machine Learning for Solar Power Advances
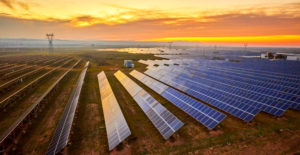
Advancing solar power means developing and identifying ever-more efficient materials for trapping solar energy – and that kind of materials science means applying powerful computing and robust data analysis. Now, researchers at Argonne National Laboratory and the University of Chicago are using machine learning to fast-track materials science for solar energy.
“It is certainly not intuitive at first, but it turns out that machine learning techniques can be used for purposes much different than recognizing images or predicting consumer needs,” said Marco Govoni, an assistant scientist in Argonne’s Materials Science division and co-author of the study, in an interview with Argonne’s Viktor Rozsa. In this case, the researchers applied machine learning to solving the quantum mechanical equations that define the absorption of light into a material.
“An important realization of our work was to understand that we could reuse information obtained for a given solid or liquid without repeating calculations for similar systems,” explained Sijia Dong, formerly a postdoctoral fellow at Argonne. “In essence, we came up with a sort of recycling protocol to reduce the complexity of calculations required to simulate absorption of light by materials and molecules.”
By streamlining the equations like this, the researchers sped up their absorption simulations by a factor of up to 200, dramatically accelerating the types of materials science necessary for next-generation solar energy development. “The time savings in the calculations of the screening using ML are remarkable,” the researchers wrote in their paper.
“Our study also gave insight into how to improve and modify the underlying theory used in the simulations,” said Giulia Galli, a senior scientist in the Materials Science division. “The impact of our machine learning exercise turned out to be further reaching than expected; the data-driven approach we adopted indicated to us new ways to study light-matter interaction in even more realistic and more complex systems than the one we started out studying.”
Now, the researchers are leveraging those same insights for other kinds of light-related problems beyond absorption, beginning with light manipulation for quantum sensing.
About the research
The research discussed in this article was published as “Machine learning dielectric screening for the simulation of excited state properties of molecules and materials” in the April 2021 issue of Chemical Science. The paper was written by Sijia S. Dong, Marco Govoni, and Giulia Galli. To read the paper, click here.
Related Items
How Machine Learning Can Help Us Stop Climate Change
Can Digital Twins Help Modernize Electric Grids?
Smart Grid Effort Seeks to Boost Coordination