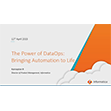
Key Questions to Ask of Your Scientific Data Platform for Single Cell Analysis
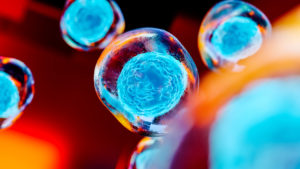
(pinkeyes/Shutterstock)
The idea of precision medicine – delivering the right drug treatment to the right patient at the right time and at the right dose – underpins current thinking in pharma R&D. And, led by innovators in “big pharma,” the role of human genetic information to drive research decisions is now firmly established. However, until single cell analysis came along, researchers were looking at an aggregated picture – the ‘omics of a whole tissue system, rather than that of a single cell type.
Now, single cell analysis has become a major focus of interest and is widely seen as the “game changer,” with the potential to take precision medicine to the next level by adding “right cell or cell types” into the mix.
Advantages Go Beyond Science
With this new ‘omics toolbox (genomics, transcriptomics, epigenomics and proteomics at the single cell level), researchers can, for example:
- Gain insight into the transition from “healthy” to “diseased” states;
- Identify and validate potential biomarkers by identifying distinct subgroups for efficiency or safety;
- Understand the mechanics of disease pathways to discover new targets;
- Plan better/more efficient clinical trials;
- Assess responses to existing drugs or available therapeutic regimens over time;
- Explore extensions to existing indications.
Analyzing historical pipeline data, Nelson et al. concluded that pipeline drug targets supported by human genetic evidence of disease association are twice as likely to lead to approved drugs.
A Problem of Scale
However, with the potential for tens of thousands of cells per patient to be analyzed, and the prerequisite to build data sets from thousands of individuals to ensure the statistical power required for decision making in R&D, there is a problem.
The technical and interpretative challenges associated with such “big data” using current tools are holding back the biological insights that should be coming out.
The Need for Agile, Scalable, and Cost-Effective Data Analytics
Database platforms and websites must evaluate key biological hypotheses by querying a mind-bending amount of single-cell data. Many established approaches and tools are simply not suitable for this challenge.
For example, many current methods require repetitive extract/transform/load operations (data science janitorial work) increasing time and computational overhead with every question asked of the data. Several also significantly constrain the number of total cells/datasets that can be inter-compared.
So, if we consider two common R&D goals, label expansion and novel target validation, as well as a more operational objective, maximizing data analytics productivity, we can propose a series of key questions to consider when you are reviewing current data analytics tools and practices or evaluating a new data analytics platform.
1. Label Expansion
What would be the impact of a system that allows you to go from weeks to days to seconds for testing precision medicine hypotheses with hundreds to thousands of patients?
Exploring what genes respond to (or are suppressed by) an already in market drug or an advanced target, and considering conditions that are associated with those genes, offers developers the opportunity to expand label indications and impact unmet clinical needs. This insight is usually gained by considering a broad combination of measures.
We are on the cusp of an era where gene responses in subpopulations of cells could provide the same resolution power for disease definition that genetic mutations provide currently. Reference datasets can be used, in conjunction with clinical data, to enable a quick survey of individual patient cellular gene responses, new tissues affected, and new cell types effected, for example. When reviewing data analytics tools for your data’s optimum performance, you should consider whether they will enable you to answer questions about new cells and tissues from all available public and internal data quickly. When you can ask questions in seconds and minutes instead of hours or weeks, that is when you will see the impact on your bottom line.
2. Target Validation
Do you have flexibility to integrate different single cell data types – RNA, protein, variants, spatial analysis – with relevant cell and tissue ontologies to speed up target validation?
Modern target validation with human data requires a broad understanding of the impact on gene expression, as well as supporting phenotype data and hospital data that can inform whether a target is associated with an impact on presence/absence of biomarkers and disease incidence or progression. To use your data efficiently, you should find out whether your current solution gives you the flexibility to integrate different single cell data types, with relevant cell and tissue ontologies. New therapy targets expand the portfolio of datasets and support targeted medicine.
3. Maximize Data Analytics Productivity
Is too much time spent chasing data provenance in single cell analysis? Do you have a production-ready solution that can be used routinely by your scientists, without needing input from your IT specialist team?
With data analytics now such a significant element in all R&D efforts, flexible infrastructure, everyday usability, and time to answer become vital ingredients for success. Downtime – when data janitorial work needs to be done, or analysis times become extended – is not just inconvenient; it can materially affect the progress of your science. It is important to look at whether you have mapped out the total cost of ownership, including lost opportunities, and human effort, as you consider how an analytics platform can support your data amplification efforts.
Critical Choices
Importantly, and clearly reflecting the questions we have posed above, much has been written about the current “state of the art” in single cell data analytics, with many describing it as:
“A series of craft methods that need to be transformed into more robust, higher throughput and more reproducible workflows.”
With this in mind, it is essential to critically assess the various data analytics “ecosystems” and “platforms” that compete for your attention and business. Powerful, optimized processing and data management and analysis methodologies are now needed more than ever to extract translational value.
About the author: Zach Pitluk, is the Vice President of Life Sciences and Healthcare at Paradigm4. Zach has worked in sales and marketing for 23 years, from being a pharmaceutical representative for BMS to management roles in Life Science technology companies. Since 2003, his positions have included VP of Business Development at Gene Network Sciences and Chief Commercial officer at Proveris Scientific. Zach earned a Ph.D. in Molecular Biophysics and Biochemistry from Yale University, and has held academic positions at Yale University Department of Molecular Biophysics and Biochemistry, where he was an Assistant Research Scientist, NIH Postdoctoral Fellow. and Graduate Student, and has been named as co-inventor on numerous patents. You can reach him via email at [email protected]
Related Items:
AI-Powered Drug Development in a Post-COVID World
Can AI Find a Cure for COVID-19?
AI Called on to Mine Massive Coronavirus Dataset, CORD-19