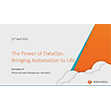
Composite AI: What Is It, and Why You Need It
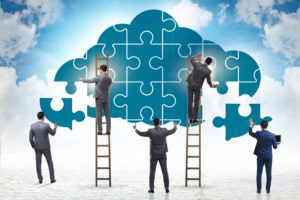
(Elnur/Shutterstock)
You might have noticed a new term, “composite AI,” floating around the cybersphere. Don’t worry–it’s not a complex new technology that you must master. In fact, while the term may be new, the core idea behind it is not. Nevertheless, it’s likely a technique that you should be thinking about incorporating in your enterprise AI processes.
Gartner helped put composite AI on the map last summer, when it published its 2020 Hype Cycle for Emerging Technologies. Simply put, Composite AI refers to the “combination of different AI techniques to achieve the best result,” according to Gartner. That’s it. Simple enough, right?
So, what other AI techniques could that mean? It’s important here to keep in mind that AI is a very broad term. While some might believe that AI refers to the latest, greatest deep learning and neural network algorithms, AI actually covers much more under its sizable umbrella.
Machine learning and deep learning are types of AI. But there are many other types of AI that should be in your wheelhouse that fall outside of the machine learning/deep learning bubble. That includes traditional rules-based systems, natural language processing (NLP), optimization techniques, and graph techniques, according to Gartner.
A composite AI system is to be built atop a “composite architecture,” which Gartner identified as its number one Hype Cycle trend for 2020. A composite architecture (you might have guessed) incorporates packaged business capabilities that run atop a flexible data fabric, thereby enabling users to take be flexible and adaptable amidst rapidly changing systems and requirements.
Multi-Disciplinarian
As the Senior Director of Data and Analytics Product Management at SAS, Saurabh Gupta is quite familiar with the central idea behind composite AI. But Gupta knows the idea by a different name.
“I’m used to saying multi-disciplinary analytics,” Gupta says.
SAS has been helping customers build multi-disciplinary analytics–ah composite AI–systems for many years, and has a multitude of technologies and pre-built applications that it can bring to the composite AI table. Machine learning typically is just part of the solution, Gupta says.
“It is possible, if your problem is straightforward, that just using machine learning is sufficient. But in order to solve the problem fully, you’ve got to use the combination of these techniques,” Gupta tells Datanami. “I think only now are people starting to look beyond the hype of machine learning and the pain of machine learning and are beginning to recognize this concept.”
Selecting the right AI technology and technique to use is not always easy or straightforward. According to Gupta, it depends on the AI practitioners having a deep understanding of the business problem that they’re trying to solve, and the data sets that are available to solve them.
“We always begin with the question in mind,” Gupta says. “So what’s the business problem you’re looking to solve for? And ultimately, what is the business decision you’re looking to make out of that particular business problem?”
Follow the Data
Once the business problem has been clearly defined, then the next step is deciding what data will inform the answer to the problem. This is another critical step in the composite AI (or multi-disciplinary analytics) process.
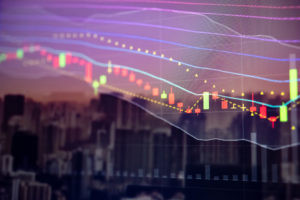
Optimization solutions can be one component of an overarching composite AI framework (Vintage Tone/Shutterstock)
“If the problem I’m looking to solve involves textual data or voice, as an example, then you know a lot of computer vision and NLP will come into play,” Gupta says. “If I have primarily structured data, then I know that a lot of statistics, ML, forecasting will come into play.”
For example, if a retailer is looking to optimize their pricing and promotional activities, they will likely be looking to use transactional data as part of their strategy, Gupta says. The first step will be defining the price elasticity of the products, which could involve machine learning techniques.
The next thing I need to do, if I Need to make a price or a promotional recommendation [is to ask] what am I optimizing for?” Gupta says. “Am I optimizing for revenue? Am I optimizing for margin? Am I optimizing for some combination of revenue and margin, or maybe market share?”
The pricing and promotional optimization exercise will actually require some combination of AI technologies and techniques, including machine learning for price elasticity and promotional lift, along with rules-based approaches and demand forecasting, Gupta says.
“That’s why we often say data science is a [team] sport,” he says. “You may have people who may be an expert in machine learning or they may be people who understand forecasting well.”
Gupta moves back and forth between using the term “composite AI” and “multi-disciplinary analytics.” At the end of the day, they pretty much mean the same thing. But SAS is adamant that it can offer all of the capabilities under a single platform, which Gupta claims is unique in the AI and analytics space.
“In all fairness I really have not seen other vendors use this term yet,” he said. “Go to any vendor’s website, you see vendors talking about unified analytics,” which is a term that SAS pioneered, Gupta says. “They’re taking off a lot of our messaging. But I haven’t heard them talking about multi-disciplinary analytics or composite AI. I’m sure it will catch on. It’s just a matter of time. But you will not really find too many systems who can do this in an integrated fashion.”
Related Items:
A ‘Glut’ of Innovation Spotted in Data Science and ML Platforms
Why Human Integration Is Critical to the Future of AI
Gartner Gets Hyped for Emerging Tech