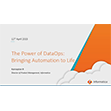
Texas A&M Reinforcement Learning Algorithm Automates Oil and Gas Reserve Forecasting
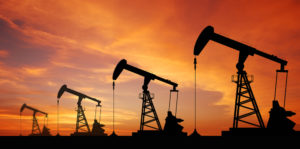
Oil and gas extraction is a messy business, not least because much of the initial discovery process relies on educated guesswork that often proves fruitless. O&G companies are always searching for ways to reduce these false positives and thereby the costs of their discovery operations – and now, a team of researchers at Texas A&M University have produced a new algorithm that automates prediction of oil and gas reserves.
“Subsurface systems that are typically a mile below our feet are completely opaque. At that depth we cannot see anything and have to use instruments to measure quantities, like pressure and rates of flow,” explained Siddharth Misra, an associate professor of geology and geophysics at Texas A&M and one of the authors of the paper, in an interview with Texas A&M’s Vandana Suresh.
Certain factors can foretell a productive well: in this case, pressure and flow measurements from boreholes. Normally, these measurements are typically fed into computationally intensive calculations to predict the structure of the underlying rock, and more often than not the process requires a human in the loop throughout much of the process.
The new algorithm, by contrast, operates via reinforcement learning, steadily growing its predictive ability by guessing about the composition of the rock, being rewarded based on whether or not it was correct, and guessing again.
“Imagine a bird in a cage,” Misra said. “The bird will interact with the boundaries of the cage where it can sit or swing or where there is food and water. It keeps getting feedback from its environment, which helps it decide which places in the cage it would rather be at a given time. Algorithms based on reinforcement learning are based on a similar idea. They too interact with an environment, but it’s a computational environment, to reach a decision or a solution to a given problem.”
After just ten iterations of the reinforcement learning process, the algorithm was quickly and accurately predicting the properties of the scenarios presented to it. While this does not yet approximate a real-world oil and gas development case, it’s a crucial first step for the research. Next, the researchers are looking to increase the complexity of the testing scenarios and the efficiency of the algorithm.
“In this study, we have turned history matching into a sequential decision-making problem, which has the potential to reduce engineers’ efforts, mitigate human bias and remove the need of large sets of labeled training data,” Misra said. “Although [this] is a first step, my goal is to have a completely automated way of using that information to accurately characterize the properties of the subsurface.”