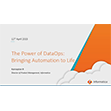
Do You Have Customer Data You Can Trust?
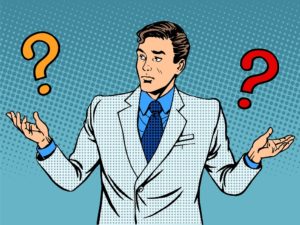
(studiostoks/Shutterstock)
Customer data is the new business success factor, yet over 76% of the 4,500+ companies we’ve worked with did not have accurate customer data. Surprised? Here’s more.
Forty-seven percent of these companies did not have any data quality management solutions in place, while the remaining relied on ETL methods to make sense of increasingly complex customer data. Only 1/3rd of the companies we spoke to (including Fortune 500 firms) were moderately satisfied with the quality of their customer data.
Despite poor data quality, companies still aspire to take on futuristic digital transformation initiatives that demand accurate, timely, complete data. The result? Catastrophic failure.
Therefore, a key question to ask before initiating any digital change is, ‘do you have customer data you can trust?’
If YES, great. If NO, here’s what you need to know.
The Three Most Common Customer Data Challenges
In the numerous demos and conversations, we’ve had with CIOs, business managers, and IT managers, there are three data quality challenges that always stands out:
1. Disparate Data: Almost every company we worked with cited disparate data as one of its most significant data quality challenges. Over the past decade, the demand for acquiring more customer data to fulfill hyper-personalization goals has increased dramatically. Companies are frantic. Hundreds of thousands of dollars are being spent on a range of enterprise apps to collect and store more data. But more data is not helpful if there’s no way to consolidate it. Add to this, third-party and external data make it even more difficult to get an accurate customer view.
The consequence of disparate data can be gaps in knowledge, impacting analytics, and business intelligence. For instance, a financial institution may want to know more about its client base to launch a new service but may overlook firmographic details stored in a CRM module, causing them to miss important insights. While this seems like an extreme example, it illustrates frequent problems companies face when they are connected to way too many apps and sources.
2. Duplicate Data: An inevitable problem, duplicate data results from heterogeneous sources. In some instances, it can also be caused by human data entry errors with multiple reps simultaneously entering information on the same platform.
Regardless of the cause, duplicates can cause a significant waste of resources as records are matched to identify duplicates. Manual data matching is itself an arduous task that results in false positives, meaning employees will have to spend more time in verifying each of these supposed duplicates.
A bank we worked with spent 30 hours each week verifying false positives for every customer record matched against various anti-money laundering lists. It had a significant problem managing customer records that did not have English names, making the process even more tedious. Duplicate data is not just a simple problem of one entity being recorded twice. It’s a complicated problem that is caused by the ever-changing nature of data.
For instance, every time a user uses a different email ID or phone number (which is usually used as unique identifiers), a duplicate is created. The company might end up assuming it has 10 new members, while it may only be 4 or 7. At an exponential scale, where thousands of records are duplicated, this means flawed insights, increased operational costs, and delayed initiatives.
3. Dirty Data: In an ideal world, data from a single source with controls in place is clean. In the real world, data comes in from multiple sources and by its very nature, it is messy. Data that is missing, mislabeled, incorrect, rife with typos, errors, fake information, etc is considered dirty data – and almost every company struggles with dirty data as a persistent problem because data ownership is unclear, data cleaning is labor-intensive and time-consuming and companies would rather ignore problems than fix it.
Even the best companies with consolidated views and unified sources will have trouble handling dirty data. To make it worse, the rapid rate of data decay (usually 5%/month for a B2B company) overwhelms companies. Sales reps constantly clean and regulate their records through manual processes. Over time, it gets exhausting. Teams focus elsewhere and the obsolete, dirty data just piles up until it threatens the success of a digital transformation initiative.
These three challenges make it impossible for companies to have customer data they can trust. But all is not lost. With the rise of self-service data preparation solutions, companies are in a better position to salvage their data. However, a solution is just a tool. Without making some core changes within the organization, no tool, no matter how efficient can help a company regain control of its data.
When companies come to us with these data challenges, we set out to know their processes and organizational attitude to data. Some of the key questions we ask our clients to help us understand their problem with data include:
- What systems are in place to manage the flow of data?
- How many CRMs or apps are they connected to at a time?
- Who owns the data?
- Is there a data quality framework in place?
- Do they have a data quality management solution in place?
- How aware are the employees of the challenges with data?
- How serious is the leadership when it comes to data quality?
- What steps are the company looking to take to resolve these challenges?
These are questions you can ask of your team too when addressing customer data quality challenges. As a starting point, here’s what you can do to initiate the process of getting clean, complete, reliable data.
How to Kickstart the Data Quality Process to Get Clean Customer Data
Going deep into technical details of the data quality process is not in the scope of this post, however, the core process can be summarized in three critical steps.
Here goes.
- Start by Conducting an Audit of Customer Data from the Last Three Months: Check for problems as:
- Human entry errors such as spelling mistakes, excessive use of abbreviations, unstructured data (john vs John), nicknames instead of actual names etc
- Inconsistencies in formatting (NY vs NewYork, mm/dd/yy vs dd/mm/yy)
- Duplicates (do a quick column match to find out the duplicate rate)
- Incomplete information such as missing fields and values
- Sources of data (how many systems are collecting/storing customer data)
Jot down problems as you come across them. The audit should serve as a preliminary understanding of the problems with your data and how it’s impacting business operations, employee productivity, customer satisfaction, revenue, sales, and so on.
- Measure the Cost of Bad Data: Skeptics may dismiss data quality issues as a universal problem that they have no control over. Some would undermine its impact. Some completely ignore the problem even exists.
To counter this problem, it’s important to measure the impact of bad data on every aspect of your marketing, sales, and customer service functions.
For instance, if you’ve spent $1,500 dollars on an email campaign to 5,000 recipients and 5% of your emails can’t reach the right recipient, that’s 250 people that don’t get the chance to spend money with you and you’ve wasted $75 the minute you hit ‘send’. Sure, $75 doesn’t sound like a lot, but it gets worse. Assuming your average sale is $500 and your conversion rate is 10%. You’ve just lost 25 sales, for a total cost of $12,575.
Moreover, if your reps are constantly on the phone verifying information or fixing information in the CRM rather than working on their accounts, you’re losing more productivity hours than you think.
- Begin Searching for the Right Data Quality Management Tool: There are dozens of data quality management tools that offer a range of capabilities. Some may cost you hundreds of thousands of dollars, some only a couple of thousand of dollars. While the budget may be the primary decision-making factor, it’s important to understand what you truly want from the product.
You can take a trial run of the top 10 data quality management tools and check for essential features as:
- Easy data integration with support for multiple files and data formats
- Ability to clean, dedupe and standardize data without the need for coding
- Ability to match data with a high accuracy rate, catering to a variety of data structures, to reduce false-positive rates
- Ability to empower business users and reduce the dependency on IT teams
A dealbreaker for any data quality management should be its ease of use and automation features. If your team has to spend hours learning a new language or using complex programming to perform necessary functions, then you’re better off developing an in-house solution. In a time when data demands real-time accuracy, there is little room for manual intervention. Therefore, automation and the ability to process large amounts of data within a short period is the need of the hour.
Once you have these three steps covered, the rest will depend on how your company maintains the process, develops data quality rules, and ensures everyone’s alignment with preserving the quality of customer data.
To Conclude – You Can No Longer Afford Poor Customer Data
To stay ahead of the curve, to find hidden opportunities, to deliver on digital transformation initiatives, to hyper-personalize, to create unique experiences, you need accurate, complete, timely, unique, customer data. Poor customer data will cause your business to be stuck in limbo, if not completely fail. What are you doing to get customer data you can trust?
About the author: With over a decade of experience working for SaaS companies, Farah Kim is known for her human-centric content approach that bridges the gap between businesses and their audience. At Data Ladder, she works as the Product Marketing Specialist, creating high-quality, high-impact content for a niche target audience of technical experts and business executives.
Related Items:
How the Lack of Good Data Is Hampering the COVID-19 Response
Room for Improvement in Data Quality, Report Says
A Bottom-Up Approach to Data Quality