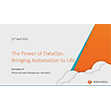
Security Partnership Combines ML with Net Flow Data
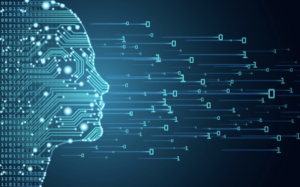
A framework known as network flow is emerging as a cyber-security tool based on the enterprise requirement for broader “network awareness” derived from network flow data.
A recent effort with roots in university research from the 1980s called the Open Argus Project is applying machine learning techniques to network flow data to spot threats in enterprise network traffic, including growing amounts of encrypted traffic.
The Argus Project that includes researchers from Carnegie Mellon University, Duke University and Stanford University announced its first commercial sponsor this week. Network security startup CounterFlow AI will contribute its proprietary technology to the project. The startup based in Charlottesville, Va., also becomes the first company to license and integrate Argus into its ThreatEye platform.
“Machine learning is a perfect fit for analyzing large sets of network flow data, and the best results will come from analyzing the best network data available,” said Carter Bullard, who is credited with developing Argus in the early 1980s at Georgia Tech. “This is now on an accelerated path thanks to CounterFlow AI’s commercial sponsorship.”
Argus backers note that as much as 80 percent of enterprise network traffic is encrypted, making traditional network security approaches like deep packet inspection largely ineffective. The growing availability of structured telemetry data has allowed startups like CounterFlow AI to develop streaming machine learning engines designed to sift through contextual flow data about network traffic to quickly identify anomalies and malicious behavior—much of it lurking in encrypted traffic.
Argus tracks 145 network attributes, including identification, services,
resource utilization, packet dynamics along with network activity metadata and content. CounterFlow AI’s partnership with the privately-funded open source project will provide the startup with the ability to analyze large sets of network flow data on its machine learning engine.
Argus is a privately funded open source project focused on advancing network flow data concepts. The Defense Department and other U.S. agencies along with federally-funded R&D centers and universities use the network flow technology to monitor network traffic for frequent and increasingly sophisticated threats.
“Encryption has become a vast playground for cybercriminals,” Deepen Desai, vice president of security research and operations at network security specialist Zscaler.
“Cybercriminals know that most organizations are unable to inspect [Secure Sockets Layer] traffic at scale,” Desai added. “So, with malicious web sites that can be set up in no time with free SSL certificates, they’re launching attacks that have a good chance of going undetected.”
In response, the combination of the Argus framework’s structured network flow data with CounterFlow AI’s machine learning engine is touted as a means of detecting threats in real time. “The type of features that Argus generates is a data scientist’s dream,” declared Randy Caldejon, the startup’s co-founder and CEO.
Recent items:
How Dark Data, DevOps, and IT Complexity Are Hurting Security
Multi-Cloud Complexity Heightens Security Threats