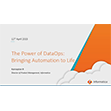
Predictive Maintenance Drives Big Gains in Real World
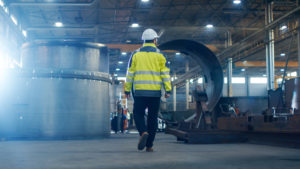
(Gorodenkoff/Shutterstock)
Nobody likes doing work that isn’t needed, since it consumes time and money with no discernable return. In an ideal world, we would perform the minimum amount of maintenance required to maximize performance or uptime. But how do we figure out when the perform that maintenance? Big data provides a better answer.
Predictive maintenance isn’t new. Mechanics have been doing it since they started working on machinery. But thanks to better sensors, bigger storage, and smarter algorithms, organizations are able to monitor machinery and predict impending failure with levels of precision that were previously impossible.
One of the companies finding good returns with predictive maintenance is Mondi, the global manufacturer of wood, paper, and plastic packaging products. The company, which runs more than 100 sites around the world, worked with analytics software company MathWorks to develop a predictive maintenance application for a plastic extruder machine in Germany.
Every time the plastic extruder machine has an abnormal shut down, it costs Mondi at least €50,000 in cleanup costs and lost revenue, according to Philipp Wallner, an industry manager with MathWorks in Munich, Germany.
“If they let the machine run to failure….they have to stop the entire line, get the remaining plastic out of the line, and clean everything, so that can easily take them a couple of hours and cost a lot of money,” Wallner said. “So they try to avoid it.”
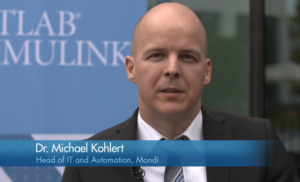
Mondi could save up to €2 million per month using predictive maintenance on 60 machines, says head of IT and automation Michael Kohlert
MathWorks was brought in to analyze data with MATLAB and create a digital model of the machine using its Simulink software. When MATLAB detects that there could be a problem – which can happen up to a half-hour before the machine breaks – the software alerts the machine operator via a GUI dashboard, and the operator can take action to avoid an abnormal shutdown.
The MathWorks software has helped Mondi reduced operating costs and the amount of waste generated by the machine, said Michael Kohlert, head of IT and automation at Mondi, which recorded about €7.5 billion in revenue in 2018.
“Our controller calculated savings of €50,000 to €80,000 for one processing problem we analyzed in this case,” Kohlert said in a video on the MathWorks website. “We want to solve about 90 more problems at our plant, directly at the machines. We have a potential of about €2 million per month to reduce. We are about to continue our cooperation with MathWorks to finalize this project for 60 more machines and lowering waste at our plant and our machines.”
Industrial Adoption
Just about every company that operates large, expensive pieces of equipment can benefit from preventive maintenance. Jet engines, for example, generate about 1TB of data per flight, per engine manufacturer General Electric.
Airlines have dabbled in predictive maintenance for the past few years, and are now expanding their use of artificial intelligence into new areas. Delta Airlines claims its 2018 implementation of the Skywise Predictive Maintenance application helped it cut maintenance-related cancellations from 5,600 in 2010 to just 55 in 2018.
Now the air carrier is doubling down on its use of machine learning with a new plan, unveiled today at the CES show in Las Vegas, to optimize its entire operation with the combination of ML and modeling.
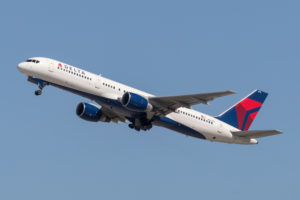
Delta Airlines claims predictive maintenance helped it reduce maintenance-related cancellations from 5,600 in 2010 to just 55 in 2018 (Ryan Fletcher/Shutterstock)
“We’ve cancelled cancellations, but we still have to deal with weather variables like hurricanes or a nasty Nor’easter, and that’s why the team in our operations and customer center is developing the industry’s first machine learning platform to help ensure a smooth operation even in extreme conditions,” Delta CEO Ed Bastain said in a CES keynote today, according to a story in Avionics International. “The system uses operational data to run scenarios and project future outcomes while simulating all the variables of running a global airline with more than 1,000 planes in the sky.”
Modeling the Machine
According to MathWork’s Wallner, today’s systems are capable of processing much more data than in the past.
“I’ve been working in this industry for close to 15 years, and in the beginning when I started off, people said ‘We cannot capture all that data. The sensors are expensive. The sensors might fail, and then you don’t have that data available. And storing data is expensive,” he says.
“But that has completely changed over the last few years,” he continues. “What we do see is storing data is not an issue at all. There is plenty of storage capacity these days. Sensors typically are not an issue anymore. We do see that modern equipment are equipped with plenty of sensors for all the inputs that you require.”
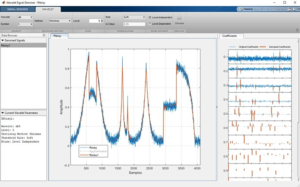
MATLAB processes sensor data using a variety of algorithms built into the software (Image source MathWorks)
If the customer does not have a sensor, they can often make up for it with inferred reading from what’s called a “virtual sensor.” For example, if a customer can’t measure the current on an electric drive, then they can infer the current from other readings, such as the angle of the drive, Wallner says.
One of the biggest problems with building predictive maintenance applications is the lack of failure data to train the models, he says. One solution to that dilemma is using simulation software to create a digital twin of the equipment. Often, the customer can feed data about abnormal readings, such as oil leakage, into the simulation.
“If you don’t have any measured failure data, that would be pretty difficult,” he says. “What we usually see is they have some real failure data and then they build out the simulation model and then fill the parameters of the simulation model with the real failure data they have. And then they become pretty close to that failure data.”
Related Items:
BMW Preempts Problems with Predictive Analytics
Go Fast and Win: The Big Data Analytics of F1 Racing
Real-Life Miners Find Gold with Data Analytics