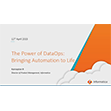
AI Clears the Skies for Tracking Bird Migrations
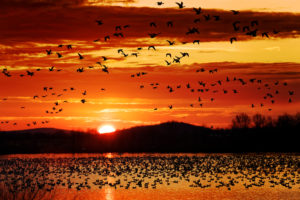
Bird migrations are so large that they appear on weather radar, filling the skies during spring and fall nights.This data could have been invaluable for ornithological research, but researchers have lacked the tools to effectively access and leverage it – until now.
Dan Sheldon, an AI researcher at the University of Massachusetts Amherst, explained the situation. Researchers had been able to conduct limited studies, identifying patterns that could differentiate weather from bird formations – but the scale of the data (hundreds of millions of images comprising many terabytes of data) bottlenecked the research.
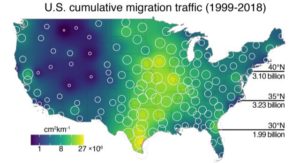
Map colors indicate estimates of migration traffic from measurements at 143 radar stations. Locations are indicated by white circles, with size proportional to migration traffic at the station. The central corridor of the U.S. receives particularly intense migration. Estimated numbers of birds crossing different latitudes are shown to the right. Image courtesy of the authors and Kyle G. Horton.
Enter MistNet, a tool developed by researchers from UMass’s College of Information and Computer Sciences and the Cornell Lab of Ornithology, among others. The tool leverages machine learning (“the latest and greatest,” Sheldon said) to analyze the patterns that distinguish birds from, say, rain, and deliver the bird-related data stored in the weather radar record – which reaches decades into the past.
The research team – led by Sheldon, along with Subhransu Maji and lead author Tsung-Yu Lin – received a grant from the National Science Foundation (NSF) to test new approaches for tackling the data. In their most recent research, they ran a large-scale validation of MistNet, comparing it to two competing approaches and employing it in a number of case studies that demonstrated its relative strengths.
Results from MistNet have broken new ground, shedding light on where (and when) migration has occurred over the last 24 years. Researchers were able to illustrate – and even animate – intensive migration areas in the United States, estimate flying velocity and more.
“We hope MistNet will enable a range of science and conservation applications. For example, we see in many places that a large amount of migration is concentrated on a few nights of the season,” Sheldon said. “Knowing this, maybe we could aid birds by turning off skyscraper lights on those nights.”
MistNet, the authors note, is perfectly timed, arriving in the midst of a surge in large datasets from citizen science projects like eBird, which allows people to submit their bird sightings for use in conservation tasks. The MistNet researchers are also pursuing answers to whether or not migration has shifted with long-term changes – namely, climate change.
“This is a really important advance,” Sheldon said. “Our results are excellent compared with humans working by hand. It allows us to go from limited 20th-century insights to 21st-century knowledge and conservation action.”
About the research
The paper referenced in this article, “MistNet: Measuring Historical Bird Migration in the US Using Archived Weather Radar Data and Convolutional Neural Networks,” will be published in Methods in Ecology and Evolution later in 2019. It was written by Tsung-Yu Lin, Kevin Winner, Garrett Bernstein, Abhay Mittal, Adriaan M. Dokter, Kyle G. Horton, Cecilia Nilsson, Benjamin M. Van Doren, Andrew Farnsworth, Frank A. La Sorte, Subhransu Maji and Daniel Sheldon.