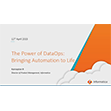
AI Hype Rockets, Hadoop Twins, and Other Learnings from Strata
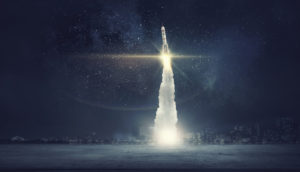
Strange similarities have been discovered to exist between Cloudera and Hortonworks now that the two former Hadoop rivals have merged into a single company. In fact, the resemblances are so great that they appear to be like “twins separated at birth,” one Cloudera executive said at the Strata Data Conference.
“We have discovered that we really were twins separated at birth,” Cloudera CIO and CDO Amy O’Connor said during her keynote address Wednesday morning.
In an interview with Datanami later, she clarified what she meant. “When we got inside each other’s companies and started meeting people, it was like, I know what you do and I know how you do it, because we’re doing the same things,” she said. “…[T]he DNA is very similar. The core of everything is very similar.”
In addition to developing, selling, and supporting similar product lines — a distribution of open source Apache Hadoop along with things like Hive, HBase, Kafka, Spark, MapReduce, and all the rest — Cloudera and Hortonworks were basically mirror images of each other in terms of how they operated internally, O’Connor says.
“It turns out that we were using pretty much the same systems,” she says. “We were using the same CRM systems, the same financial systems, the same forecasting systems, the same systems to manage our professional services organization… There were nuances but in general they were pretty darn similar.”
That similarity has made it easier to integrate the two companies, which were once fierce rivals that rarely passed up an opportunity to denigrate the other. That animosity is now gone, and the company is now moving forward with a singular purpose.
For Love of CAT
The focus of the Strata Data Conference is no longer Hadoop. Rather, AI is what’s moving the needle. But actually implementing AI is tougher than it looks, according to Dinesh Nirmal, IBM‘s vice president of development for AI.
“The reality is only 4% of executives say they have implemented AI,” Nirmal said in his keynote. “Unless you’re an enterprise that was born in the last five years, which is very rare, it is hard, because you have what I call the burden of legacy.”
To help organizations get on the right track and overcome that burden of legacy, Nirmal recommends they follow his handy guide, called CAT. The acronym stands for:
Culture. “Culture has to come from bottom up. Developers have to buy into that culture. It has to be a decision culture.”
Architecture: “You’ve got to make sure you have an architecture that’s lasting and expansive. Kubernetes gives you some level of it, but you need a data layer on top of it.”
Technology: “That becomes a critical pillar. You don’t want to take thousands of shiny objects from 20 different products and bring it all together. You want a single data platform that can give you that’s built on top of OS source.”
Rebranding Data Scientists
Of course, no enterprise is going to implement any strategy without the right people on board. But it turns out the cast of characters that is necessary for implementing data and AI strategy is also morphing, according to Ben Lorica, the chief data scientist at O’Reilly Media and one of the hosts of the Strata Data Conference.
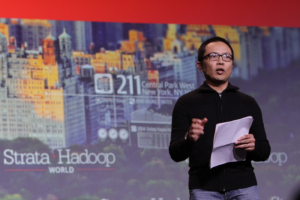
O’Reilly Media Chief Data Scientist Ben Lorica says a wave of new hardware for deep learning this summer will reduce training times by 40x
“Several years ago one of the hottest jobs in the industry was data scientist,” Lorica said during his keynote yesterday. “And that’s still a very attractive job. Although I have to say the term data scientist has become muddled a little bit.”
Lorica said he has heard that some companies are beginning to call their business analysts — the folks who know SQL and drive business intelligence tools — data scientists. “There has been confusion about who to call data scientists,” he said.
At the same time, new titles have begun to emerge for the folks who are skilled at wielding and implementing machine learning models, Lorica said. In particular, the title “machine learning engineer” started to pop up among companies in the San Francisco Bay Area several years ago, and has since spread.
On the spectrum of skills, machine learning engineers sit somewhere between data scientists (the classic definition, not the SQL power users) and software engineers. Machine learning engineers are experts at bringing machine learning technology into production, Lorica said, and most importantly, their salaries are higher than data scientists.
“There’s a certain amount of rebranding among data scientist,” he said. “Data scientists two years ago refereed to themselves as data scientists now want to be referred to as machine learning engineers.”
AI Hype Rocket
There’s no doubt there’s a lot of hype around AI. In the past few years, the interest around AI has exceeded data science, with expectations possibly exceeding what AI is ready to deliver.
Dataiku‘s Lead Data Scientist Jed Dougherty led Strata attendees through a short history of AI products. The beginning of the current hype wave began around 2015, which coincided with the launch of Amazon’s Alexa in 2015.
“Shortly after that, we start to see this uptick in AI interest that’s going up much faster than the data science,” Dougherty said, displaying a Google Trends graph that shows relative interest in AI and data science. “And finally right now, a massive spike! Is that the singularity? Probably not. It’s a hype rocket. But that’s okay — we can ride that hype rocket.”
Interest in AI is exploding in a way that even interest in data science never has, he said. But how do we actually define AI? That’s a topic that Dataiku CEO Florian Douetteau recently addressed in a blog that presents a rating system to determine the sophistication of various AI systems.
Douetteau created rubric that measures a prospective AI system along four axes, including perception, learning, interaction, and complex decision-making. For each category, the system is measured on a scale of 0 to 2, with 0 being the least sophisticated and 2 the most.
According to this rubric, Alexa would score a 1 in all categories, while a predictive maintenance application would score a 1 in only one category. The hotdog/not hotdog model from the hit comedy “Silicon Valley” would also score rather poorly. The only application scoring perfect 2s would be Lt. Commander Data from the Starship Enterprise, but he won’t actually exist for another 200 years, Dougherty points out.
“The moral of the story here is that both data science and AI are fledgling industries and there’s still huge business gains to be made from technologies and techniques that are very far from what we might consider AI when using a realistic AI Score like Florian’s,” Doughtery points out. “And that’s okay. Companies are putting products and techniques in place in every day that have a score of 0 to 2 in the AI score and are profiting quite nicely from these implementations.”
While it might seem that your competitors are about to achieve a singular breakthrough with AI technologies, that is probably not the case. Instead of panicking, Dougherty encourages prospective AI users to start at the bottom of the pyramid and build up.
“Build your foundation then decide whether and how your company would benefit from the types of technologies that register a high AI score before you climb into that hype rocket,” he says.
Related Items:
Hadoop Was Hard to Find at Strata This Week
Why 2018 Will Be The Year Of The Data Engineer