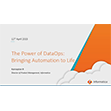
Big Data, Big Problems? Responsible Data Management in 2019
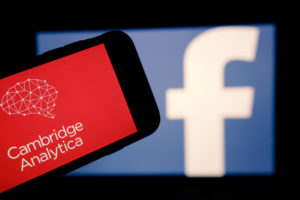
(AlexandraPopova/Shutterstock)
If 2018 taught us one thing about data collection, it would be the existence of a distinct and profound line between companies using data for good and those whose business model relies on its exploitation. As Facebook gears up to pay a multi-billion dollar fine to the FTC as a result of the Cambridge Analytica scandal, it’s time we as an industry evaluate how we handle data.
The recent NewVantage Partners survey found that businesses are ramping up investment in big data and analytics initiatives. In fact, 55% of firms surveyed indicated they would be investing more than $50M in 2019. At the same time, we’ve seen countless examples where companies have gathered more consumer data through opaque and ethically murky ways. Most recently, Google has come under fire for its Screenwise Meter app which allowed the company to monitor and analyze user traffic and data through a VPN. The app has since been disabled on Apple iOS for violating privacy rules but has put a well-earned spotlight on VPN privacy issues. What’s more, now the news is out that Google never disclosed that its Nest Secure in-home solution included a microphone that according to Google “was never supposed to be a secret,” and yet somehow was.
Consumers today are reaching heightened levels of data consciousness and as a result, are taking more precautions about allowing their data to be shared. Data presents a clear business value but it’s up to organizations to understand and recognize the potential ethical implications.
So, how can we advocate for more companies using data ethically in aggregate, separate from those whose business models are dependent on exploiting — or even selling — data?
For starters, we can look to where it’s been done successfully before. After all, being a data-hungry company isn’t necessarily an evil practice. Take the landing gear of a 747 airplane, for example. It generates 4TB of sensor data with every landing and uses that data to improve safety, lower maintenance costs, and minimize travel delays. In 2018, Bechtel, the engineering company that built the Hoover Dam and English Channel Tunnel, disrupted its own technology by tapping into proprietary data to inspect worksites faster and reduced the time spent on drafting plans. Pitt Ohio, the freight company, tapped its historical data and predictive analytics to increase delivery estimates to a 99% accuracy rate while increasing revenue and reducing the risk of lost customers.
As a professional with more than 20 years in enterprise product management at data-specific companies, I’ve seen the intricacies of data management first hand. I’ve witnessed success and mismanagement, unfortunately, and it’s time we hold the decision makers responsible for their roles in assuring proper oversight. Here are three solutions that organizations should prioritize when it comes to responsible data management.
1. Compliance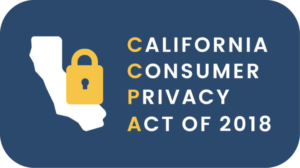
With GDPR now in full effect, companies theoretically should have operating procedures in place to ensure they are regulatory compliant. As of December 2018 however, the International Association of Privacy Professionals (IAPP) found that less than 50% of its survey respondents were in fact GDPR compliant.
GDPR is here to stay and while your business may not have EU customers or contracts, it’s important to remember that GDPR applies to citizens, not organizations. I predict the “right to be forgotten” will become a more universal tenet, and foresee greater personal autonomy when it comes to data ownership. With California’s digital privacy act now signed into law, I suspect other states will follow suit.
Ensuring your organization is not only compliant but staying ahead of anticipated regulation, is an effective and foolproof way to ensure your company handles its internal data properly. Such practices will no doubt yield long term benefits, eliminating time and resources needed to assure your company remains compliant.
2. Data For Good
We’ve entered into heightened consumer awareness of just how much data we produce. And while advancing AI and its use cases continue to divide the American public, consumers will be looking to corporations and organizations to lead the way when it comes to data-for-good modeling.
We’re also seeing the weighted importance of ethics intertwined with the rise of the chief data officer (CDO) role. Gartner’s most recent CDO survey found the number of CDOs citing ethics as part of their responsibilities increased 10 percentage points from 2016 to 2017. That’s a very good thing and starts to address the famous concern Jeff Goldblum expressed about reanimating dinosaurs in the original Jurassic Park movie. This ethical expansion of the CDO role suggests companies are getting more serious about asking the question about what they should do more often.
Deciding how to ethically manage your own company’s data is just as important as identifying business partners and customers who hold the same standard. Looking to organizations whose purpose relates to data-for-good initiatives like DataKind or Digital Impact is a great place to start. Further, there are a number of data and analytics vendors with internal data-for-good initiatives. Salesforce, for example, supports philanthropic and environmental causes via its 1-1-1 Model and Teradata supporting social service causes with it’s Doing Good With Data program.
3. Effective Innovation
We’re at the forefront of leveraging AI for its most powerful uses yet. As the U.S. bolsters its investment in AI technology, we are reinforcing the message that AI is the future and will help solve some of our most complex problems.
But we’ve hit an inflection point when it comes to AI explainability. As it stands there is still a great deal of confusion and misunderstanding around in AI and machine learning today. On the surface level, we understand what the technology is doing but not necessarily how it’s doing it. Though some may dismiss the idea of explainable AI, there is growing recognition that for AI to be its most effective, we must work toward its explainability.
What we must dig into are the algorithms and pathways in which we connect the data that will help us understand how we arrive at the conclusions and outcomes we do. If AI is going to surround us in our personal and professional lives, helping someone understand what it’s doing can’t be like reading the user agreement for iTunes – long, complex, impenetrable by non-experts. Explainable AI means simple pictures and explanations so people can understand and trust the AI processes at work around them.
Collecting data responsibly and using innovative solutions such as graph technologies are key factors in how we can drive AI explainability and outcomes. AI explainability in this way acts as an omniscient force, bringing transparency to the outcomes for organizations, customers, and regulators.
As players in the big data and analytics industry, we must advocate for smarter and safer data-hungry companies. We must encourage and expect businesses to partake in, and promote, the practice of responsible data collection and management before they’re forced to by regulators. The implications of the data we collect ripple far and wide and we can no longer ignore the changing tide.
About the author: Lance Walter is the chief marketing officer for Neo4j. Lance has more than two decades of enterprise product management and marketing experience. He started his career in technical roles at Oracle supporting enterprise relational database deployments. Since then, Lance has worked at industry leaders like Siebel Systems and Business Objects, as well as successful startups including Onlink (acquired by Siebel Systems), Pentaho (acquired by Hitachi Data Systems), Aria Systems and Capriza. Lance’s first experience with alternative database platforms was at Arbor Software, the pioneer of the multi-dimensional database / OLAP market.
Related Items:
High Growth Companies Extending Their Lead with AI
Building a Successful Data Governance Strategy
California’s New Data Privacy Law Takes Effect in 2020