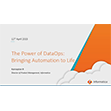
No Time Like the Present for AI: The Journey to a Successful Deployment
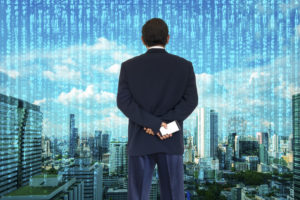
(Montri Nipitvittaya/Shutterstock)
Today, over a decade since MapReduce technologies reshaped the field of data science, we find ourselves seeking more insight from even more data, ever more quickly. The confluence of improvements in computer processing power, networking performance, and storage platforms has enabled a massive leap forward in the next phase of data insight; machine learning (ML), deep learning (DL) and artificial intelligence (AI).
While ML can improve the breadth, depth, and speed of insight that one can extract from one’s data, making the decision to adopt ML techniques is just the first step on a path that is arguably still being paved.
At its core, AI is about data, so you can’t realistically begin an AI deployment journey unless you have well-curated data sets to feed the system. In most cases, data for AI is often derived from years of accumulation or from massive amounts of newly created ephemeral data. In other cases, massive data sets are being created and analyzed in real time. Regardless of source, all must be curated, as this is a critical precursor to successfully leveraging AI/ML/DL technologies.
It’s a daunting task, sure, but the old adage ‘junk in, junk out’ is true, particularly with AI. The value of what you get out of this level of analysis is directly correlated to the quality of the input data. The slightest corruption of input data can be amplified through an AI system in ways that you cannot correct later, so getting it right from the beginning is critical.
The next step in the AI journey is obtaining the necessary expertise to design the AI algorithms and workflows unique to the questions being asked of the data. Do you train resources internally and delay your start? Do you hire experts to join the organization to shorten your time to productivity? Or do you leverage contractors who have no experience with your organization and will need some level of training? The answer often depends on how much time you have to deliver results.
Having resolved the human resource component, the next question you need to consider is where you are going to run your AI workloads. The choice generally narrows to building an on-premises platform or running in the cloud. Both options have strengths and challenges that need to be weighed against your organizational objectives. However, AI compute platforms differ significantly from general IT and web platforms in their density (up to 50Kw per rack), network architectures, and storage patterns. These differences drive infrastructure requirements that impact selection of platforms, data centers, and cloud providers, so thinking this step through carefully is critical.
Opting for a cloud strategy to build your organization’s AI capability can be a sound decision if your utilization is sporadic. This option requires a detailed understanding of your cloud provider’s readiness for AI workloads. For example, your provider will need dense graphics processing unit (GPU) computing platforms, high-bandwidth networks, and a high-performance storage platform.
You will also want to seek a provider with usage models and fee structures that are tailored to AI workloads, to ensure that the right resources are available to you at a reasonable and predictable price. This will help avoid issues along the end-to-end workflow and performance issues around resource sizing and data migration as well as surprise, hidden fees. You will also have to ensure that the security policies of your cloud provider are on par with those of your organization.
Deploying an on-premises AI platform is a good option if your organization has enough work to sustain 50% or greater utilization rates. In addition to being somewhat capital intensive, this route will require expertise in AI system architecture, AI system administration, and a facility that can accommodate the power density of an AI platform. Fortunately, this expertise can be hired internally or derived from supplier or consultant relationships.
The benefits of this approach include the ability to adjust the platform to your organization’s changing needs and the evolving state of AI technologies. Having an on-premises platform is also more cost-effective at higher utilization and builds a core skill set that will benefit your company for years to come.
But, there are also a variety of hybrid AI platform options emerging in the market that may be a better fit for you. For example, in one model, your organization would own the platform (which would be designed by an AI specialty firm). It would be housed in a data center that is tuned for AI densities and managed by administrators familiar with AI platforms and workloads.
There is also the AI platform-as-a-service model, wherein the AI vendor designs the platform to meet your needs, deploys it in your data center, and runs it as a service on your behalf. Both these models can reduce time-to-productivity, augment your internal AI expertise, and deliver on better-than-cloud economics.
Whatever path you choose, the next step in your journey is deciding what precisely you’re going to build. This design phase of AI deployment is a challenge because AI workloads demand balanced systems. Balance refers to the fact that these systems must take into account the interdependencies of networking, computing, and storage to eliminate potential bottlenecks and enable scaling. The specific role that each of these design components plays in relation to the whole varies significantly based on the goal of the AI system and the nature of the data being analyzed, making it complicated to design.
One underlying factor is that AI workloads have immense communications requirements, with data moving back and forth between compute and storage components at different frequencies and in different volumes. That means you need to consider balancing server-to-server traffic, server-to-storage traffic, and data access by users.
However, your networking design also has to factor in how much new data is coming in to the system from external sources and at what frequency. Many AI workflows can also require large external pipes that are not needed for general enterprise groups or are very expensive in the cloud.
In addition to the size of your data sets, the rate of change of the data sets and the provenance of your data sets, you will have to address data hierarchies within your AI workflow and supporting platform with a tiered strategy that can address the large quantities of data and nature of that data’s ephemeral importance.
Your networking design can also leverage high-speed architectures for “near-line” data being operated on and use slower, cheaper technologies for massive data lakes and cooler storage. This strategy enables the dynamic, high-speed data manipulation that most AI workflows require but requires an experienced hand to execute.
But, lest you think the challenges were over, even after you’ve addressed networking issues and other complex, interconnected aspects of designing the system, the next step in the journey – physically creating a functioning AI system – is also a challenge.
Historically, the information technology (IT) infrastructure market has comprised vendors who have a discrete focus in one architectural component; computing, storage, or networking. Yes, the bigger hardware companies have business units that will sell you all of these components but that’s what they’ll do, sell you the components of the solution. You’ll still need to have them integrated by professionals who know how to turn the discrete parts into a fully-functioning AI system.
Pre-packaged, spot solutions provide capable platforms for well-known enterprise workloads. However, AI architectures are evolving and dynamic even though they still need to be tightly integrated and balanced. In addition, technology generally is quickly moving away from pre-packaged solutions and towards software defined architectures and IA is the quintessential expression of that movement.
Beyond that, vendors offering you only pre-packed solutions don’t have the intimate knowledge of all the areas within an AI system, computing, networking, and storage, to effectively guide the creation of an AI platform, where all the pieces have to play together in new and complex ways. Given the disparate backgrounds and divergent interests of those discrete spot component suppliers, they are not likely to easily work together and deliver seamless results for the customer.
Fortunately, the market is changing. In the same way you no longer have to choose just cloud or on-premises, there are custom design shops who can help design a well-balanced AI platform that is tailored to the specific needs of your organization. These unique firms have the engineering depth, breadth of products and the hands-on AI experience to know how designs play out in practice and provide a strong option.
Before you can pull the trigger and execute, though, you need to consider what it will look like when the platform is operational. Specifically, how will you manage this system? This last step in the AI journey is often the scariest due to the newness of the market and the general lack of available expertise and administrative talent. After all, if you don’t have the expertise to design an AI system, then it’s likely you won’t have the expertise to manage it.
The fact is, administration of balanced AI platforms is a skill set not readily available in most enterprises today. Unfortunately, this may be the case for the coming two-three years, as we train more people in the trade. Even worse, the dynamic and evolving nature of AI compounds the challenge by creating a moving target that will leave the industry playing catch up for years to come.
Your next step in the AI journey is likely to be identifying a competent services provider who can manage these environments as you build up your internal expertise. These services providers are often so experienced that they have the ability to manage your AI platform on-premises as well as remotely (via specially developed tool sets).
Not surprisingly, given the level of technical skill required, the pool of such expert vendors is even smaller than the number of options for designers and integrators. Before selecting a vendor, you will want to be sure that your potential vendor has demonstrable experience managing AI systems and, ideally, in designing them, as that gives an additional perspective. You will also want to be sure that your potential vendor has a clear understanding of your AI objectives and the applications you intend to use to deliver on those objectives. It’s important not to give up at this stage, though, and see the journey through to completion or your previous efforts will have been for naught.
We are at the forefront of what is one of the most anticipated and potentially game changing technological disciplines in the history of computing. The raw materials for success are finally at hand and the technology supply chain is in overdrive trying to catch up. That said, don’t let the apparent incompleteness of the technology deter you from jumping into the fray now. There are great companies in the market that can light the path to results for you today and help you stay ahead of your competition in the future.
About the author: Matt Jacobs is the senior vice president of commercial systems for Penguin Computing. Matt is responsible for building out the global partner ecosystem for Penguin Computing, setting vertical market growth plans across commercial markets, and overseeing the commercial sales team. He joined Penguin Computing in June 2000 and was responsible for both sales and setting and executing the company’s high performance computing (HPC) strategy. Prior to joining Penguin, Matt served as the Western Regional Sales Manager for the Server and Storage division of American Megatrends, Inc. He holds a B.A. from the University of Georgia.
Related Items:
The Tipping Point for Artificial Intelligence
Gartner Sees AI Democratized in Latest ‘Hype Cycle’