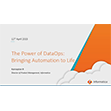
How Machine Learning Is Disrupting the Real Estate Market
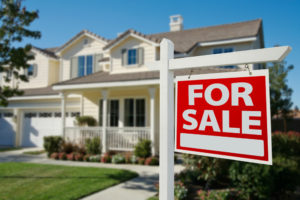
(Andy Dean Photography/Shutterstock)
Big data has changed many aspects of our lives, but the real estate market has remained relatively undisturbed. Now a group of companies are looking to leverage big data and machine learning tech to upend the process of buying and selling real estate.
One company using big data tech to streaming real estate transactions is Landis, which has developed a market-making platform that’s designed to match institutional buyers (primarily in New York City) with residential property owners (primarily in the Midwest).
Figuring out which houses to bid on is a time-consuming, largely manual effort, says Landis co-founder Cyril Berdugo, who previously was head of acquisitions for a large institutional real estate investor called Crown Acquisitions in New York.
“I would get deals sent to me by brokers on a daily basis, and I would have my team of analysts and associates look through deals, underwrite them, value them, put together Excel spreadsheets to see whether they would be interesting for us to bid on,” Berdugo says. “That took us a lot of time.”
One day, Berdugo decided to try and automate the process a bit. He shared some of the acquisition requirements with some agents, including data describing what Crown had already bought and what it was looking to buy in the next year. The results were very promising.
“By empowering agents with a tiny bit of data, we were able to close on three times as many deals as we were able to close on the year before,” Berdugo says. “That completed changed the nature of the game for us at Crown.”
Detecting Sell Signals
Berdugo teamed up with Tom Petit, his former Stanford University classmate, and designed Landis. The goal was to build a business-to-business (B2B) platform that connected institutional buyers and sellers in residential real estate.
The first step in the Landis process – and arguably the most difficult – is collecting data about the real estate that buyers might be interested in. “There’s so much data in real estate that’s not being used. It’s mind-blowing,” says Petit, a data scientist who previously worked at Airbnb.
Property tax records provide an abundant source of data about homes, but scraping the data from county websites and databases that lack standard APIs can be a difficult engineering feat, Petit says.
“It takes quite a bit of work to bring it all together, but it’s all there and it’s accessible,” he says. Federal mortgage data and data from sites like Zillow, such as the Zestimate, help to round out an individual property’s picture in the Landis platform.
Once potential transaction targets are identified, Landis lets the machine learning loose on the data. The company uses a range of classification algorithms to sort out which owners might be flippers, which might be your typical owner, and who might be long-term buyers.
Armed with this insight, Landis can preemptively reach out to the owners – typically mom and pop investors in the Midwest — to see if they might be interested in selling before they have hired an agent or listed the property for sale on the local MLS.
“We can spot from the data patterns when sellers might be selling property, when flippers might be selling property and we can actually pre-empt them doing that,” Berdugo tells Datanami. “We can see it far enough in advance that we can give them a call and say, hey we think you might be selling those properties two months from now.”
The Buying Game
On the other side of Landis’ fence are the big institutional buyers, and machine learning techniques are used here too. By uploading data about previous purchases and holdings into the Landis system – including deals that they bid on but didn’t win – Landis’ machine learning algorithms can automatically identify the homes that possess the features that the institutional buyers desire the most.
“We actually have a scoring mechanism where, for each property, we assess the probability that a specific buyer is going to submit a competitive bid,” Petit says. “And we use this to only show the buyers very targeted deals [based on] what they have bought in the past and what they told us they want to buy.”
Buyers don’t have access to all of Landis’ listings. Instead, they only see properties that would be a relatively good match for them based on the preferences that surface from the data. All of the listings on the Landis platform are private – that is, they’re not on the MLS – which gives the institutional buyers some room to hammer out a deal.
“We can tell the sellers, hey instead of going through a traditional agent that’s going to take three months to sell going through the market, and is going cost you 6% [in commissions], why don’t you give them to us for a few weeks?” Berdugo says. “We already have relationships with investors who can make you a cash offer, not for just one property at a time but entire portfolios.”
Landis charges a 2.5% commission to sellers and a 1.5% commission to buyers, which Berdugo says buyers are very willing to pay. Not only does the company cut the cost of completing a real-estate transaction by eliminating the need for agents, but it speeds it up.
“A traditional real-estate transaction takes six to eight months, which is pretty painful,” Berdugo says. “In our transactions….. it takes 40 days total, which is something our clients are very happy about.”
Related Items:
Machine Learning Used to Value Real Estate