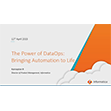
Full-Stack Link Analysis for the Masses
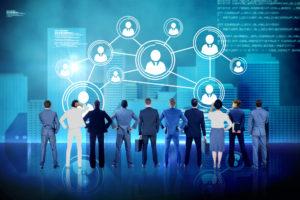
(wavebreakmedia/Shutterstock)
There are lots of ways organizations can find meaningful information hidden in huge datasets. In the recent past, small armies of analysts would pore through the data using rudimentary tools. More recently, large corporations and federal agencies have used large-scale link analysis to piece together full pictures from disparate bits. Now a firm called Gemini Data is looking to bring that type of knowledge discovery to the masses.
Gemini Data develops a full-stack data analysis platform that leverages graph database, machine reasoning, and link analysis capabilities to enable human analysts to discover more connections hidden in data than they would using manual methods. The company says is approach to continuous data analysis, or CDA, enables customers to create a knowledge repository that grows more refined as more data is analyzed.
The platform, which is built on Cassandra and Neo4j NoSQL databases, is used often by IT and security teams who need fast analysis of machine and sensor data. In that sense, it picks up where products like Splunk and Elastic leave off, says Gemini’s Chief Product Officer Navin Ganeshan.
“Unlike the prior breed-of-services that focus mostly on aggregation and providing some basic correlation,” Ganeshan says, “CDA essentially stitches together what we call a knowledge graph, a contextual intelligence layer, that sits above data that then becomes useful for solving investigation and understanding events that occur in your data.”
The knowledge graph is based on a native ontology developed by Gemini that’s composed of a large number classes, or heuristic rules about the world, Ganeshan says. That ontology allows the product to not only automatically identify what pieces of data — such as IP addresses, server names, or AWS Availability Zones – actually mean, but also how they relate to other data entities stored in the graph database.
“By applying machine reasoning at scale, we can actually take it further,” Ganeshan says. “It’s not just A plus B equals C. But because of what else we know, we can actually infer that A has to be equal to H, or H has a particular relationship with B that is not directly evident in the data, but are logical conclusions based on what we know about ontology.”
‘Palantir for the Masses’
Placed in the hands of an analyst, Gemini allows them to start with an event, such as an anomalous router message or a suspicious email address, and then work out from there. The product’s GUI guides the analyst through possible connections to that particular piece of data, allowing the analyst to quickly and iteratively explore how different pieces of data might be connected.
“What makes the process itself work is we actually provide a lot of power to the analyst,” Ganeshan says. “We’re not making pre-determined conclusions of what it could be. All we’re doing is re-arranging and visualizing the information in a way that has insights and enables logical problem solving. We’re taking the grunt work away so the analyst can work through, iteratively, different hunches. We take the effort away that’s associated with that.”
The software also enables lets users track “tribal knowledge” with the goal of helping teams of analysts to work together. “A lot of these are deep cycle problems that are not something where an analyst can spend a couple of seconds and figure out what’s going on,” Ganeshan. “It requires a little bit of a hand-off between different analysts, and possibly even be annotations.”
Machine learning also plays a role, albeit a relatively minor one. While the graph database and the ontology provide the scalable link analysis, Gemini uses machine learning to improve the user interaction with the product, and to narrow down the possible range of data and challenges that an analyst might be interested in. So if the system detects a pattern of events that was previously flagged as malicious, the machine learning algorithms will be on the lookout for a similar pattern, and surface that up to the user as a recommended action
While many analytics software developers these days are looking to use machine learning and deep learning techniques to automate decision-making, Gemini Data espouses a different viewpoint entirely. For Gemini, it’s all about using AI to make existing human analysts more productive.
“The core problem for analyst teams is not a technical one. It’s a people problem,” says Ganeshan says. “There are a lot of platforms that do the detection piece….but then it becomes a challenge for the analysts. How many things can we look at today? That’s the problem we’re out to solve — how to minimize the things they take a look at and accelerate problem solving.”
These are the types of challenges – and the types of solutions — that large corporations and governmental agencies have been working with for years. Faced with a big data glut and a shortage of trained analysts, firms in the national defense, cybersecurity, and counter terrorism sectors have sought more powerful solutions that give them an edge.
“It’s essentially the analysis problems that were observed 10 to 15 years ago by these larger sectors,” Ganeshan says. “This is also where companies like Palantir came from. We’re starting to see that level of capability really filtering down in a way that’s much more useful and more affordable.”
Like Palantir, but without the seven-digit price tag? “Palantir for the masses,” he quips.
Digital Force Multiplier
Palantir and other products like it have been adopted by large law enforcement agencies, and they reportedly have led to big improvements in the way police and others use data. National defense and intelligence agencies have also bought or – more likely – built their own solutions that super-charge the abilities of human analysts. Now that the technology has matured, it’s ready to be more widely adopted.
“This was happening as much as 20 years ago, where an analyst typically has hundreds of incidents or events to potential analyze on a daily basis,” Ganeshan says. “Contextual intelligence becomes a key part of being able to do that, of being able to access everything you need to know about a particular entity, understanding that full historical and 360 degree view context, becomes really important.”
Ganeshan came out of the cybersecurity field, and so did Tony Ayaz, Gemini’s co-founder and CEO. Cybersecurity is definitely one of the company’s target markets, but it’s not the only one. The San Francisco-based company also boasts customers in the automotive, financial services, high tech, and healthcare industries.
There’s a growing awareness of the power of graph databases and the link analysis they enable. Because of their special way that graph databases can render knowledge, they are one of the fastest growing segments in the overall data management space.
One of the things that makes Gemini unique is its full-stack approach. In addition to bringing an optimized Neo4j database replete with a pre-built ontological schema, the product handles the bulk of the data ingest and data munging tasks that so often eat into time that can otherwise be spent on the actual analysis.
“Others who have done this tend to focus only on the analyses, and stayed away from the complexity of actually merging, optimizing, and hosting the access to data,” Ganeshan says. “That’s one thing that’s core to what we do.”
Gemini Data sells its solution three ways, including as a software offering, as a cloud service, and as a hardware appliance.
Related Items:
Why Knowledge Graphs Are Foundational to Artificial Intelligenc
Inside the Panama Papers: How Cloud Analytics Made It All Possible
Graph Analytics Poised to Solve Tough Big Data Problems