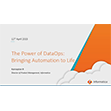
Reporter’s Notebook: The 2018 GTC Experience
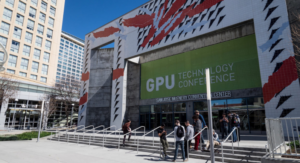
Nvidia’s GPU Technology Conference (GTC) is winding down after four days of frenzied GPU action. It was this reporter’s first foray to this particular show, which attracted 8,500 GPU-loving (or just GPU-curious) folks to downtown San Jose. Here are some highlights.
For starters, there was no new silicon unveiled, no new processors to show off. That immediately relegated this conference to second-class status in the minds of people who love new hardware. Still, Nvidia managed some impressive announcements, including the DGX-2, a cluster of 16 GPUs connected via a new NVLink Switch (NVSwitch) memory fabric that delivers GPU-to-GPU connections at speeds of 300 GB per second.
Nvidia CEO and co-founder Jensen Huang dubbed the 350-pound machine that will ship by September 30 “the world’s largest GPU.” At just $399,000, the DGX-2 offers 2 petaflops of half-precession -point horsepower and can replace $3 million worth of CPU-based servers for HPC, deep learning, or rendering workloads, while consuming just one-eighteenth of the electricity, the company says. Huang drilled that math home relentlessly with a message he repeated over and over: “The more you buy, the more you save.” The San Jose McEnery Convention Center was turned into a GPU church, and Huang the charismatic preacher leading us to the co-processing promised land.
Huang clearly knows how to put on a show – and in some cases steal the show. A normal man, after giving an impassioned, two-and-a-half hour keynote address before 8,000 attendees in which he delved with great detail into what seemed like all of the company’s many business lines, would perhaps want to take a breather, get some water, settle down. But Huang, whose energy apparently knows no bounds, instead dropped in on an executive panel that Nvidia scheduled during the press pool’s lunch. Huang took questions for more than half an hour, to the delight of surprised reporters.
Many of the questions in that impromptu press conference revolved around Nvidia’s autonomous vehicle (AV) business. It was a case of unfortunate timing for Nvidia, which just happened to schedule its annual tech conference – as well as its annual investor’s day – to take place a week after two fatal accidents involving its AV partners, including a self-driving Uber car that hit and killed a pedestrian in Tempe, Arizona on March 18, and a Tesla Model X that slammed into a concrete barrier on Highway 101 in Silicon Valley five days later, killing the driver (it’s still unclear if the Tesla was in Autopilot mode).
Nvidia and Uber decided to shut down their AV testing while investigations take place. The move was also made out of respect to the families of the deceased, which of course is the right thing to do, even if Wall Street reacts by taking billions off your company’s market cap. The AV accidents had the unfortunate side-effect of overshadowing the real progress that Nvidia and its partners are making on the AV front, including Nvidia’s newly unveiled plan to test AV algorithms in a virtual reality-based simulator, dubbed Drive Constellation. “What happened was tragic and sad,” Huang told reporters. “It also is a reminder that’s the exact reason why we’re doing this. We’re developing this technology because we believe it will save lives.”
AVs were sprinkled throughout the show, and Nvidia even parked its self-driving semi-truck in front of the convention center. The Santa Clara company was clearly banking on AVs being the physical personification of the technological shift that its GPUs are driving. While AVs are cool and provide a compelling visual, a more significant shift is occurring behind the scenes, in the data centers of technology giants and the start-ups that hope to become the next Google. That shift, of course, is the rise of deep learning.
AVs may have gotten the headlines for GTC, and the improvements in virtual reality and augmented reality (VR and AR) weren’t far behind. But outside of the consumer realm, the real star of the show was deep learning. On the GTC Expo floor, there were dozens of vendors dedicated to helping organizations leverage GPUs and libraries like TensorFlow, Keras, Caffe, and MXnet to train deep learning models on huge amounts of data – the baseline technique that has become the torch-bearer for the next generation of artificial intelligence (AI). The vendors that talked to this reporter all reported a much increased level of interest in using deep learning.
Deep learning and AI stole the show. In his keynote, Huang marveled at how far we’ve come since the computer scientist Alex Krizhevsky paved the way for a deep learning revolution when he used pair of Nvidia GPUs to train a convolutional neural network (CNN) to classify ImageNet back in 2013. At the time, it took Krizhevsky six days to train his CNN upon the 15 million labeled high resolution images in ImageNet. But the DGX-1 machines that Nvidia is selling today are far more powerful than the GTX 580s that Krizhevsky used back in 2013.
“It’s truly amazing how far we’ve come in the last five years,” Huang said. “We thought it would be fun just to do a report card to see what happened in the last five years. This is what it looks like. It took him [Krizhevsky] six days, but those were six worthwhile days because he became world famous, he won ImageNet, and kicked off the deep learning revolution. Five years later you can train it in 18 minutes, 500 times faster….To be able to do any task of great importance 500 times faster, or to be able to do a task of great importance 500 times larger in the same time — this is some kind of super-charged law that we’re experiencing.”
That law, of course, is a continuation of Moore’s Law, which has been delivering ever-better processing speeds for the past 40 years, since Intel co-founder Gordon Moore first came up with it. However, while Moore’s Law was initially postulated to apply to speedups in the central processing unit (CPU), the IT industry is expanding its reach and getting more oomph from other parts of the computer, including the GPU processors that work in tangent with the CPU to speed up graphics processing, floating point arithmetic, and now deep machine learning models. “This is an innovation that’s not just about a chip. This is an innovation about the entire stack,” Huang said.
Intel was not officially at Nvidia’s show, which is not surprising. The company did host a small soiree offsite, where it invited reporters and others to chat about its own compute accelerators, like Xeon Phi. While Nvidia is getting the lion’s share of the credit for helping to bootstrap a new form of computing – deep learning — seemingly out of thin air, the Intel execs pointed out that their approach requires fewer software modifications than Nvidia’s. Software packages like CUDA are not required when moving X86 workloads to Phi, Intel says.
In the end, it’s clear that the market is ripe for non-CPU approaches to running emerging workloads, like navigating AVs, powering VR, rendering of high-end graphics – and of course deep learning. Huang threw cold water on the idea of using GPUs for trendy workloads, like mining Bitcoin on the Blockchain, where ASICS are taking off. For GPUs, the hottest workload at the moment clearly is deep learning, and if the excitement displayed at GTC this week is any indication, the market will need lots and lots of GPUs in the years to come.
Related Items:
Nvidia Riding High as GPU Workloads and Capabilities Soar
Nvidia Ups Hardware Game with 16-GPU DGX-2 Server and 18-Port NVSwitch (HPCwire)
Hail Can’t Hide from Deep Learning, Researcher Finds