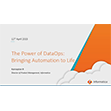
Elastic Plots Its Own Course to Big Data Success
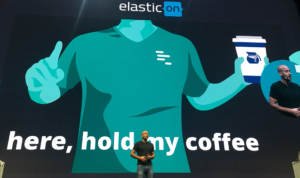
Shay Banon at Elastic{ON} 2018
Elastic ostensibly is best known as the vendor behind Elasticsearch, the scalable Lucene-based search engine that’s among the most popular open source projects on the Web. But Elastic has become so much more than that over the years, and that progress was on full display during the company’s recent Elasticon conference.
About 2,500 people attended the three-day show in downtown San Francisco, just up the road from one of two main offices for the rapidly growing company. The conference was a great showcase of what real-world customers are doing with Elastic‘s products, including: Credit Suisse, which front-ends a deep learning hacker-detection app with Elastic’s tools; Fermilab, which uses Elastic products to collect data from high-energy physics experiments; and General Dynamics, which uses Elastic tools as part of a massive data pipeline into the cloud.
It’s evident from the show that Elastic has become a diverse vendor satisfying a variety of needs for high-end enterprise companies. It’s clearly not just a search company anymore. Here are three takeaways from Datanami‘s visit.
1. Growth Via Adjacency
Elastic has demonstrated remarkable growth since Shay Banon released the first version of Elasticsearch eight years ago, both in terms of total downloads and product scope. Today’s open source Elastic Stack is composed of Elasticsearch, Logstash, Kibana, and Beats, while X-Pack providing enterprise features like security, monitoring, and alerting (all free ), as well as reporting, graph database, and machine learning functionality (not free). The software is also available on the cloud, which the company says is the fastest growing part of its business.
Elastic has grown the way videos go viral on YouTube, which has led to some gaudy download figures for its 100% open-source Elastic software stack. The growth was relatively modest for years, until it started to pick up a few years ago. A year ago, Elastic reported 100 million downloads since the platform was open sourced seven years ago. Now that figure is 225 million downloads. At this rate of growth, which is in excess of 2x, Elastic will exceed 500 million downloads by 2019, and hit a billion by 2020. Those are gaudy figures that are unlikely to be matched by other open source projects.
Elastic’s growth tends to follow a pattern at the customer level. The software is often brought in to solve one use case – say site search. The user discovers the software is pretty good, and then they start using it for something else – say log monitoring — and before you know it, they’re using more of the stack.
Meanwhile, the software has demonstrated a capability to move laterally within an organization. Jeff Yoshimura, Elastic’s vice president of worldwide marketing, shared a story about how one of the biggest banks in the world used Elastic. When a technology executive at the bank discovered many of his departments were using the software, he decided he needed to standardize the engagement with Elastic, and signed a support contract. The company now has thousands of paying customers.
Elastic is consciously encouraging this rapid growth by doing several things well. First, it’s developing good software that’s easy to use, which isn’t an easy thing to do. It smartly expands its products to tackle use cases that its users face.
What started out as a search engine built on Lucene became a log monitor and a visualizer and, lo and behold, it’s now getting into the application performance management (APM) business, largely because emerging Docker and Kubernetes environments can be tough to manage. “We’re working really hard to make Elasticsearch a great engine not only for text, but also for numbers,” Banon said during his keynote address last week. “APM is a natural place for us to go to.”
A similar thing is happening with the new roll-ups feature that Elastic demonstrated at the show. While the company was initially hesitant to add roll-ups, the team eventually relented and is now giving customers the capability to slice and dice the data they have stored in Elasticsearch across various attributes, much like a column-oriented MPP database can do.
It’s all about adding value, Banon says. “Adding a search box to your website eight years ago or taking a log file that was sitting somewhere unattended and then somebody being able to get visibility to what it’s producing, to download software and actually use it to solve a real world problem,” he said during his opening keynote last month. “You didn’t download a platform. You didn’t download a data lake or something like that. You actually downloaded a product that actually brought you value. That’s what we try to do with our users, and trusting us to solve other types of use cases as we grow through our users.”
2. Machine Learning Is Elastic’s Secret Weapon
The long-term pervasiveness of search might be Elastic’s calling card, but machine learning is its secret weapon.
Following its 2016 acquisition of Prelert, Elastic set about re-architecting how the C++ machine learning technology, which was originally developed to work with Splunk, integrates into the Elastic stack. The machine learning capabilities are premium features available only to users who pay for a subscription to the high-end X-Pack offering.
The goal is to make machine learning another easy-to-use but powerful feature that can address a host of data opportunities. So while one day Elastic’s machine learning is helping to spot anomalies in router logs, the next it might be used to proactively develop a forecast that’s used to optimize a supply chain. “We want to go way beyond anomaly detection,” Banon said. “Our goal is to slowly start to address other aspects in the machine learning space.”
Banon’s machine learning guy is Steve Dodson, who originally developed the Prelert technology to replace the increasingly brittle rules-based systems that big organizations use to monitor IT operations. That original focus on addressing IT and security-related challenges is now morphing into a broader goal to bring machine learning technology to bear on other business opportunities.
“Because of our machine learning component, data science teams are now engaging with us,” Dodson told Datanami at Elasticon. “That introduces a lot more conversations. Like for instance, I have this data, can you segment my users for me based on these particular features? Or can you cluster these things and detect outliers in a multi-dimensional space? …We’re starting to deploy more flexible — I’d almost call it traditional — machine learning capabilities.”
While Elastic’s user base still appears to be mostly an IT-centric crowd, it’s starting to cultivate a bigger data science following. The availability of a Python client that can work with popular Python-based libraries, like NumPy, SciPy, and Scikit-Learn, is helping that transition. The fact that the machine learning engine was designed to process data in real time is another.
“At the moment, we’re actually building out a data science group in our machine learning group,” says Dodson, who official title is tech lead for machine learning. “We’re not going to do everything in the machine learning space. We’re going to do this bit and shrink wrap it and make it really easy to use, and if you want to train a classifier using a particular method and model, we’ll show you the best practice for doing that at scale in the Elasticsearch cluster.”
3. IPO Is In The Plans
While there are no concrete plans as yet, Elastic definitely has its sights sets on an initial public offering (IPO) in the future, Banon said at the show. While other big data software companies may be comfortable staying privately held, going public is the best way to solidify Elastic’s reputation and prepare it for the next stage of growth, Banon said.
This makes sense for several reasons. First, Elastic’s number one competitor, Splunk, is already public, so being public would eliminate that advantage that Splunk holds over Elastic, which could matter in close deals for enterprise clients.
Also, going public would demand a level of accountability and transparency in Elastic’s business operations that is not required of private firms. Banon said that going public is essentially a requirement to be considered in the upper rung of software companies.
Lastly, it would provide a way to raise more money, and alternatively provide a way for early investors to monetize some of their investments (although they may want to hang onto the stock if they believe it could appreciate considerably). Banon mentioned several acquisitions that Elastic has already completed, and said there would probably be more.
Elastic is a company on the move. In the wake of the disintegration of Hadoop as the center of the big data ecosystem, the company is finding traction with its mantra of a unified open source stack under a single vendor’s control, that is easy to use, and that solves business problems.
Related Items:
Mr. Robot Thrills Elastic Crowd with Real-World Hacks
Elastic to Release Source Code for X-Pack