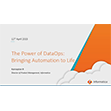
Faking It: Dealing with Counterfeits in the Age of AI
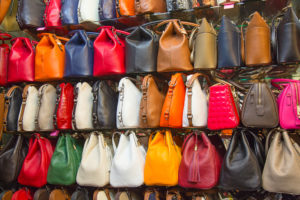
(Fedor Selivanov/Shutterstock)
The news isn’t the only thing being faked these days. Thanks to cutting edge machine learning techniques, it’s easier than ever for people to generate counterfeit videos, audio recordings, and signatures. Today’s algorithms dramatically lower the bar for generating accurate replicates, but do the breakthroughs cut both ways?
It’s been a year since American citizens woke up to discover that Donald Trump was elected president. According to nearly all the pollsters, that wasn’t supposed to happen. But it did, of course, and ever since, we’ve been questioning the accuracy of those public statistics while wallowing in a bounty of fake news.
But fake news is just the tip of the iceberg, it turns out. Thanks to the advent of deep learning, we are on the cusp of possessing the disturbing capability to generate a multitude of fake media, including videos and audio recordings that literally put arbitrary words into people’s mouths.
Synthesizing Obama
Researchers at the University of Washington have created videos that show former President Barack Obama saying things that he didn’t say when the video was filmed.
Their trick, which is detailed in their June 2017 paper “Synthesizing Obama: Learning Lip Sync from Audio,” involves training a neural network on millions of video frames taken from Obama’s State of the Union speeches.
The so-called “video from audio” problem has stymied technologists for years, but the U of W researchers think they’ve broken the code. The key lies in the brute force processing of neural networks combined with the large number of videos of Obama speaking. The combination let them get small details right. “Our approach for generating photorealistic mouth texture,” they write, “preserves fine detail in the lips and teeth, and reproduces time-varying wrinkles and dimples around the mouth and chin.”
Now, Obama did say everything the researchers put into his mouth; he just said them in a different in place and time, such as on talk show or an interview filmed decades earlier. But it wouldn’t have been that hard for them to put new words in his mouth – that is, to create whole-cloth fabrications – because creating a “clone” of one’s voice is actually a much easier problem to solve.
One company that recently tackled this challenge is Lyrebird. Earlier this year, the Canadian startup launched a beta program for an algorithm that can learn a user’s speech pattern in 60 seconds, and then use that pattern to generate any sentence in that user’s own voice. The results aren’t perfect, but the early synthesized Obama and Trump voices on Lyrebird’s website show a lot of promise.
There are good uses for each of these technologies. The U of W researchers say their tech could be used to reduce the amount of bandwidth needed for video coding and transmission, to enable lip-reading from over-the-phone audio, and for special effects. The folks at Lyrebird, meanwhile, say they’ve gotten a ton of feedback from the virtual reality (VR) crowd. Other users are audiobooks or personal assistants that use a variety of voices (yes, Siri and Alexa may be looking for new jobs).
But it doesn’t take an evil mastermind to think up more nefarious uses for this technology. One dodge that immediately comes to mind is the Grandparent Scam, where criminals call elderly people, pretend to be their grandkids, and then ask for money. If a grandparent were to encounter a believable voice and face on Skype and FaceTime, they would be even more susceptible to wiring them money.
Fake Writing
Fraudsters are always looking to use the latest, greatest technology to perpetrate their cons. But even our old-fashioned, paper-based world is susceptible to new forms counterfeiting. Indeed, cybersecurity expert Dr. Richard White says to be wary of “traditional snail mail” for International Fraud Awareness Week, which lasts from November 12 to 18.
While fraudsters are quite good at generating believable forms, emails and Web pages, technology could soon allow them to create handwritten forgeries with minimal effort.
For example, researchers at the University College London recently shared a program that can automatically replicate a person’s handwriting based on a sample of a single paragraph. The program, dubbed “My Text in Your Handwriting,” does this by using machine learning technology to learn about the specifics of a person’s own handwriting style, or his “glyphs,” including character choices, pen-line texture, color, inter-character ligatures, and vertical and horizontal spacing.
“Up until now, the only way to produce computer-generated text that resembles a specific person’s handwriting would be to use a relevant font,” UCL Computer Science Professor Dr. Oisin Mac Aodha said in a 2016 UCL story. “The problem with such fonts is that it is often clear that the text has not been penned by hand, which loses the character and personal touch of a handwritten piece of text. What we’ve developed removes this problem and so could be used in a wide variety of commercial and personal circumstances.”
The UCL researchers hope the technology could allow people who have suffered from strokes to regain the ability to pen handwritten notes. But could the technology also be used to generate counterfeit handwritten notes? Sure. But the UCL researchers are just as adamant that the reverse is true: that the technology could be used to flush out the fakes.
“Forgery and forensic handwriting analysis are still almost entirely manual processes,” UCL professor Dr. Gabriel Brostow. “But by taking the novel approach of viewing handwriting as texture-synthesis, we can use our software to characterise handwriting to quantify the odds that something was forged.”
Spotting Phonies
This idea – using machine learning technology to detect forgeries – is also being put to use in the retail sector. Counterfeit goods amount to upwards of 7% of global trade, amounting to a $460 billion tax on law-abiding folks every year. If you’ve ever been duped into buying a fake Rolex watch or counterfeit Louis Vuitton bag, then you know you’ve contributed your share of this unfortunate tax.
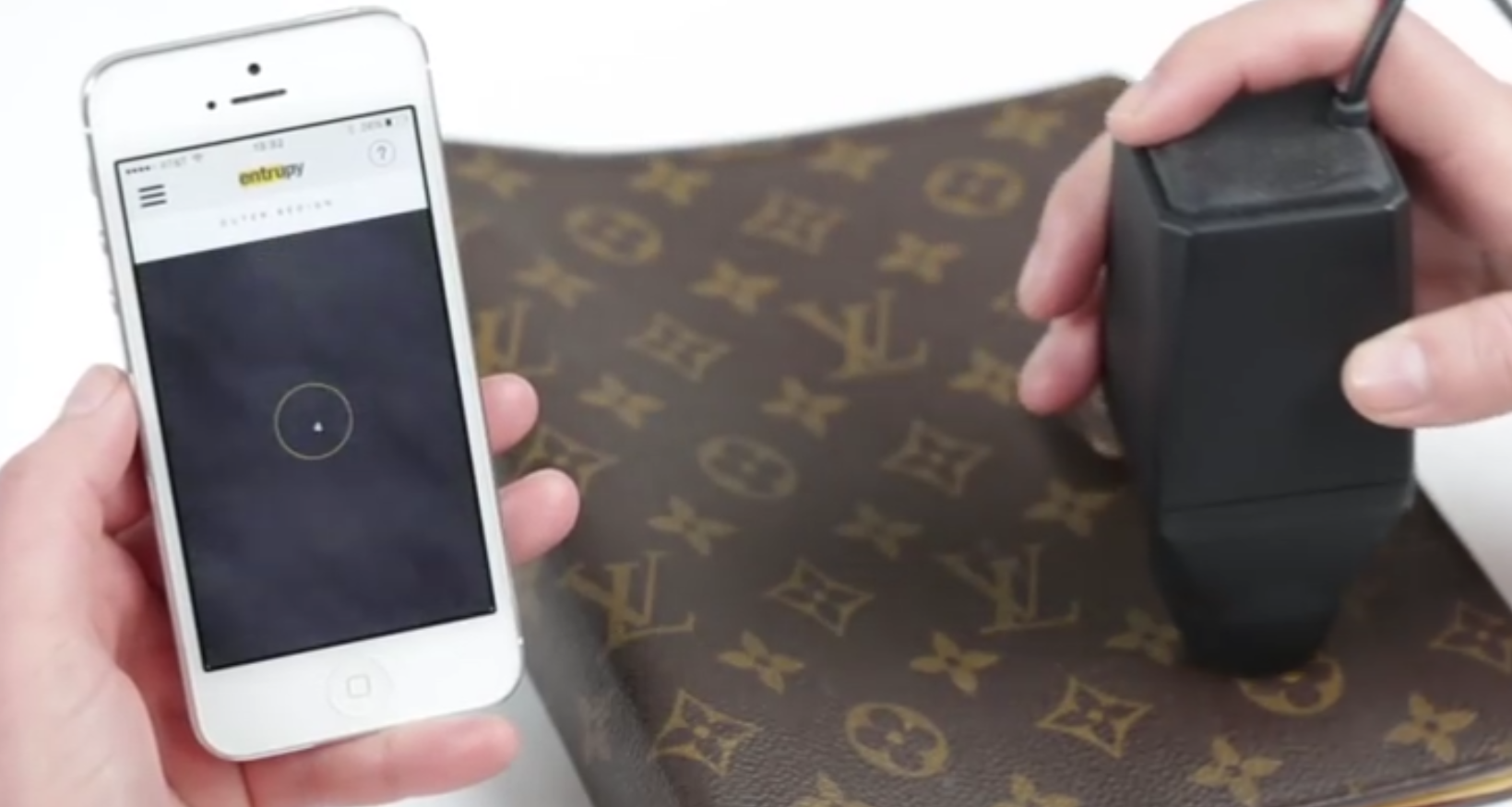
Is it a real Louis Vuitton? Entrupy’s app uses deep learning to make that call.
Now a company called Entrupy is using deep learning techniques to put a ding in the profits of the counterfeit rings. Entrupy customers get a special microscope with up to 300x magnification that they use to photograph detailed images of an item in question. After the customer uploads the photo, the company uses a series of algorithms to compare the new images to samples of authentic merchandise stored in its database. The customer gets a response in 15 to 20 seconds, as well as a certificate of authenticity from Entrupy.
According to an August scientific paper written by the company’s PhDs., the database is composed 3 million images of fabrics, leather, pills, electronics, toys, and shoes. The core machine learning algorithms at play include an eight-layer convolutional neural network used for image classification, and a “bag of visual words” approach paired with a support vector machine to determine authenticity or falsity.
“Our SVM based supervised algorithm provides an accuracy of 95% for authenticating based on a single microscopic image and the convolutional neural network provides an enhanced accuracy of 98% per image,” the Entrupy researchers write.
Of course, there’s nothing stopping the counterfeiters from attempting to defeat such authentication checks by upping their game and generating handbags, watches, shoes, and toys with better materials and superior construction. But that would cut into their margins, and thus may not be desirable.
In the end, it’s clear that machine learning provides a double-edge sword to the world. On the one hand, it lowers the barrier of entry for creating counterfeit media, faked signatures, phony goods. But we’re also seeing how the power of algorithms can work toward
Related Items:
Fake News, AI, and the Search for Truth
Trump Victory: How Did the National Polls Get It So Wrong?
U.S. Eyes Tools to Spot Faked Images, Video