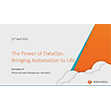
H2O Ups AI Ante Via Nvidia GPU Integration
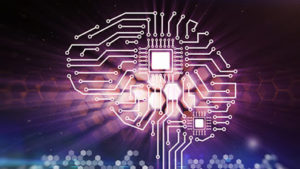
(Tatiana Shepeleva/Shutterstock)
H2O.ai, the machine-learning specialist that unveiled its “Driverless AI” platform this past summer, is upgrading the system via integration with GPU specialist Nvidia’s AI development system.
The Mountain View, Calif., company said last week it has integrated its AI platform designed to address the shortage of data scientists with Nvidia’s DGX platform. DGX is built on Nvidia’s Volta GPU and targets data scientists pursuing AI projects such as training deep learning models.
With many AI experts flocking to AI software giants such as Google (NASDAQ: GOOGL), Facebook (NASDAQ: FB) and Microsoft Research (NASDAQ: MSFT), startups like H2O.ai have emerged to help fill the data science gap. “There is a vacuum in the diverse talent needed to transform businesses with AI,” the company noted in announcing integration of Driverless AI with the Nvidia (NASDAQ: NVDA) platform.
H2O.ai said its GPU-accelerated algorithms are optimized for DGX, which includes a software stack running on Nvidia’s Tesla V100 datacenter GPU. Claiming Driverless AI represents a “killer app” for DGX appliances, the AI approach is designed to combine an “expert system of recipes for AI” with open GPU-based algorithms, the partners said last week.
The integrated offering includes an open-source machine-learning library called H2O4GPU built for the DGX systems. The library is designed to allow developers to build multiple models in parallel while evaluating thousands of features. The result is said to be “Kaggle-level capabilities,” a reference to the high-octane data science competition site acquired in March by Google.
Unveiled in July, Driverless AI combines the startup’s automated machine- and deep-learning tools, AutoML and AutoDL, that provide automated training of models running on GPU-accelerated hardware. Driverless AI automates many of the tasks associated with building, running, and maintaining a predictive analytics pipeline. Those tasks include data preparation, and identifying the best algorithms to use along with development and deploying models.
“Automation is key to industrial strength AI,” asserted Sri Ambati, co-founder and CEO of H2O.ai. The partners claimed the integration of the Driverless AI and DGX would help automate “the entire data pipeline,” thereby reducing the amount of time required for data preparation and model deployment from days to hours.
Driverless AI on DGX systems is available now from Nvidia and Trace3, a member of the GPU maker’s partner network.
While rivals focus on leveraging GPUs to automate AI, market leaders have stepped into the AI hardware race with approaches such as Google’s Tensor Processing Unit (TPU) and other chips designed specifically for deep learning workloads. The search giant initially used TPUs for the inference stage of a deep neural network.
In May, Google rolled out a full-blown AI initiative built around TPUs that included the latest iteration of its TensorFlow machine-learning framework called Cloud TPU optimized for both model training and inference.
Recent items:
H2O.ai Boasts New AI Product Like ‘Kaggle Grandmaster in a Box’
Google Buys Data Science Competition Site Kaggle